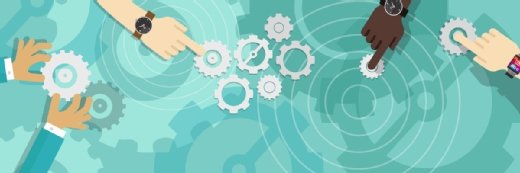
Regenstrief Institute Framework Addresses Patient Matching Accuracy
The eight-pronged framework will work as a ‘measuring stick,’ providing consistent guidelines to assess the accuracy of patient matching algorithms.
Regenstrief Institute researchers recently announced the launch of an eight-point framework that aims to improve patient matching accuracy through algorithm evaluations.
Connecting patent records from disparate sources across medical providers or facilities, known as patient matching, can significantly impact patient care.
Patient health data is often spread across several EHRs from doctors' offices, hospitals, and health systems. Healthcare providers must match those files to get a complete picture of their patient’s health history.
However, patient matching often falls short for many health systems across the United States, posing risks to interoperability, hospital finances, and patient safety. Some studies put the cost of duplicate medical records and mismatched data at $1,950 per patient per inpatient stay.
Additionally, researchers pointed out that one-third of rejected insurance claims are linked to inaccurate patient identification, costing the US healthcare system $6 billion annually.
There must be a standard approach to effectively integrating data for the same patient across information systems and organizations to create a complete longitudinal patient record, the researchers stated.
In the absence of a unique patient identifier (UPI), health systems depend on patient matching algorithms that leverage patient demographic information, social security numbers, and other identifiers extracted from existing medical records to link patient records.
“We recognize that the need for patient matching is not going away and that we need standardized methods to uniquely identify patients,” Shaun Grannis, MD, vice president for Data and Analytics at Regenstrief Institute, said in a press release. “Current patient matching algorithms come in many different flavors, shapes, and sizes.”
“To be able to compare how one performs against the other, or even to understand how they might interact together, we have to have a standard way of assessment,” Grannis continued. “We have produced a novel, robust framework for consistent and reproducible evaluation. Simply put, the framework we’ve developed at Regenstrief provides a ‘measuring stick’ for the effectiveness of patient matching tools.”
The framework provides guidelines for evaluating the validity and performance of algorithms to create a high-quality, gold-standard data set.
The initial three steps of the framework include recommended manual review reporting factors for preparing a gold-standard data set and record pairs through data description, preprocessing, blocking, and sampling. The review should:
- Examine how a complete data set is collected and from where it is sourced
- Evaluate the way data fields are selected and standardized
- Assess processes, such as blocking, to group record pairs based on schemes
- Understand what methods were used to sample record pairs based on blocking schemas
Regenstrief Institute also outlined four reporting elements: human training, adjudication processes, result analysis, and a description of software and reviewers.
The framework explains that the review process should begin with reviewer instruction and includes steps to assess records, evaluate biases and results, and resolve discordance. Additionally, experts should create a training record linkage data set, along with a gold standard, for the chosen reviewers to train with.
Afterward, researchers are encouraged to measure inter-rater reliability to understand the variation between reviewers.
Additionally, experts should provide a description of software, forms, or other support tools used to present record pairs. Finally, studies should have a review of characteristics such as the total number of reviewers, age, gender, race, cultural background, and prior experience with clinical or public health data and record linkage research.
“Automated methods for record linkage are becoming increasingly important as healthcare systems have widely adopted electronic health records and federal interests advocate for accurate patent matching,” researchers wrote. “A critical step in creating and validating patient matching algorithms is establishing a gold standard to evaluate such algorithms against.”
Currently, record linkage is broadly accepted as the solution to improve the quality and completeness of patient records.
The framework can improve upon record linkage; the authors noted that their development “can help record linkage method developers provide necessary transparency when creating and validating gold standard reference matching data sets. In turn, this transparency will support both the internal and external validity of recording linkage studies and improve the robustness of new record linkage strategies.”
Overall, this framework may support technology developers and healthcare organizations in developing a national strategy and approach to patient matching, the researchers concluded.
“We need to have a common way of measuring and understanding how algorithms for patient matching work,” said Grannis. “Our eight-pronged approach helps to cover the waterfront of what needs to be evaluated. Laying out the framework and specifying the tasks and activities that need to be completed goes a long way toward standardizing patient matching.”