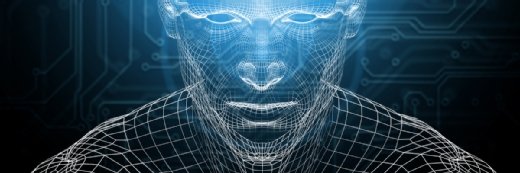
Getty Images
Machine Learning Tool Predicts Staffing Needs During COVID-19
The machine learning model tracks local hospitalization volumes and the rate of confirmed COVID-19 cases to predict staffing needs during the pandemic.
Researchers from Cedars-Sinai have developed a machine learning tool that can forecast data points related to the COVID-19 pandemic and predict staffing needs.
For more coronavirus updates, visit our resource page, updated twice daily by Xtelligent Healthcare Media.
When COVID-19 began to spread across the US, healthcare organizations were faced with the challenge of accurately forecasting the number of patients who would need hospitalization. Entities also needed to have an idea of how many patients would be in the ICU and would need ventilators, as well as how much personal protective equipment (PPE) staff would use.
A data science team at Cedars-Sinai adjusted a machine learning platform’s algorithm to predict factors related to coronavirus. The platform now tracks local hospitalization volumes and the rate of confirmed COVID-19 cases, running multiple forecasting models to help anticipate and prepare for increasing COVID-19 patient volumes with an 85 percent to 95 percent degree of accuracy.
"Our goal is to have the capacity and the right care available every day to treat the patients who need us, which fluctuates on a daily basis," said Michael Thompson, executive director of Enterprise Data Intelligence at Cedars-Sinai, which developed the platform and runs the forecasts. "We need to match that daily demand with the necessary resources: beds, staff, PPE and other supplies."
The researchers’ estimates could enable managers to appropriately schedule employees, gauge how long current medical supplies will last, and prepare for increases and decreases in hospitalized patients.
The team initially developed the algorithm to optimize patient care at the hospital. The platform has analyzed thousands of data points, such as patient vital signs and length of stay, to predict the most effective treatment for patients, pinpoint which patients have a high likelihood of readmission, and forecast which patients will be most satisfied with their hospital experience.
With the machine learning algorithm, the platform can constantly learn from its mistakes and grow increasingly more accurate, the research team noted.
"If it predicts that tomorrow we’ll have 100 COVID-19 patients, but that actual number turns out to be 90, then the platform automatically goes back and tries to relearn what changed to cause the outcome to be different," Thompson said. "The platform hones its ability to recognize patterns and becomes smarter every day."
Other large health systems and healthcare organizations have developed algorithms to better prepare for surges during the COVID-19 pandemic. Researchers at Vanderbilt’s Data Science Institute are developing a big data model that will address the impact of COVID-19 on procedures and resources now and in the future. The model will allow users to visualize and simulate varying patient loads and possible hospital mitigation policies, including the deferral of elective surgeries, re-sterilization of PPE, and additional staffing.
“This project involves all of the data scientists at the Data Science Institute, as well as geospatial mapping experts in the Vanderbilt Research IT Service and the Jean and Alexander Heard Libraries. It also allows our master’s students to collaborate on work that is of paramount importance,” said Douglas Schmidt, Cornelius Vanderbilt Professor of Engineering and co-director of the Data Science Institute.
“This is an opportunity for these young data scientists to develop their skills and have a tangible impact on addressing COVID-19.”
In April, Cleveland Clinic also unveiled predictive analytics models to help forecast patient volume, bed capacity, ventilator availability, and other metrics. The models provide timely, reliable information for hospitals and health systems to optimize care delivery for COVID-19 and other patients.
“These predictive models were developed jointly by two organizations that understand patient populations, data and modeling,” said Chris Donovan, executive director of Enterprise Information Management & Analytics at Cleveland Clinic.
“We are sharing the models publicly so health systems and government agencies globally can use them in their own communities. Our hope is that others contribute their ideas and improvements to the models as well.”