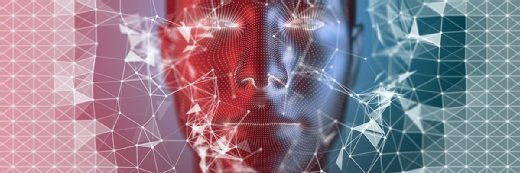
Getty Images
New Imaging Analytics Method May Boost Schizophrenia Treatment
Researchers have developed tools to improve imaging analytics, enabling the team to make connections between brain activity and schizophrenia.
An innovative imaging analytics method helped researchers categorize subgroups of schizophrenia patients using functional magnetic resonance imaging (fMRI) data, according to a study published in NeuroImage.
The technique could enable researchers to identify key patterns in brain imaging for those with mental illness, and assist in the diagnosis and treatment of patients with mental illnesses that are difficult to identify. Additionally, the method could show medical practitioners whether the current treatments have or have not been working based on image groupings.
Diagnosing and treating mental illness is an incredibly complex process, researchers noted. The same illness can present differently across patients, and there is usually no singular treatment that will be effective for all patients. Once a treatment is in place, its success can also vary from patient to patient.
Researchers from the University of Maryland, Baltimore County (UMBC) set out to respond to this variability by giving medical practitioners an objective way to analyze fMRI results for patients within relatively homogeneous diagnostic subgroups. The team also wanted to allow providers to compare fMRI results over time for the same patient.
The group developed the new method, called independent vector analysis (IVA) for common substance extraction (CS). Previously, there was no clear way to categorize schizophrenia in patients based on brain imaging alone. With this new technique, researchers can show that there is a significant connection between a patient’s brain activity and their diagnoses.
"The most exciting part is that we found out the identified subgroups possess clinical significance by looking at their diagnostic symptoms," said Qunfang Long, a PhD candidate at UMBC in electrical engineering. "This finding encouraged us to put more effort into the study of subtypes of patients with schizophrenia using neuroimaging data."
The IVA-CS approach used to identify these subgroups also preserves nuances in the data, but still provides statistically significant groupings.
"Now that data-driven methods have gained popularity, a big challenge has been capturing the variability for each subject while simultaneously performing analysis on fMRI datasets from a large number of subjects. Now we can perform this analysis effectively, and can identify meaningful groupings of subjects," said Tülay Adali, professor of computer science and electrical engineering and director of UMBC's Machine Learning for Signal Processing Lab.
The method has the potential to improve treatment of certain mental illnesses, and allow providers to know if a particular therapy is working effectively. A patient with schizophrenia could receive treatment and return in six months for another evaluation. If their fMRI data resembles that of the control group of mentally healthy patients more than that of other patients with schizophrenia, that is objective evidence that the treatment is working.
On a larger scale, this data provides a better look at patients’ medical outcomes as a result of treatment.
The team will work to further improve the IVA-CS method, with researchers leveraging longitudinal data to determine which treatments work best for subgroups of patients with specific mental illnesses. The method will also be used in a longitudinal study of adolescents to see whether there are links between fMRI images and the addiction and substance use patterns of those adolescents over time.
With this new approach, the researchers expect to improve treatment and diagnosis for schizophrenia and other mental illnesses.
“These findings emphasize the importance of interpreting subtypes of schizophrenia in terms of both the neuroimaging data analysis and the clinically diagnostic data. A better understanding of the underlying heterogeneity of the disorder in the future may lead to improved categorization and treatment strategies,” researchers concluded.