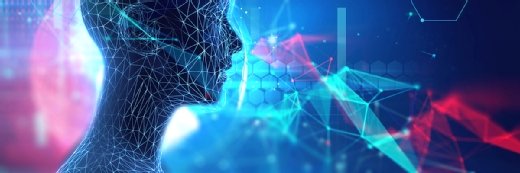
Getty Images
How Elevance Health, MVP Health Care Are Leveraging Data Analytics
The two payers share how they leverage data analytics, predictive modeling, and data quality frameworks to improve outcomes for members.
Payers rely on accurate, high-quality data to make health insurance processes more efficient and support improved patient outcomes. However, each beneficiary generates a plethora of data points throughout their care journeys that payers must sift through.
Many payers have turned to data analytics solutions to transform healthcare big data into actionable insights, including Elevance Health and MVP Health Care.
Elevance Health, formerly Anthem, is an Indianapolis, Indiana-based payer serving over 118 million customers across the United States through its affiliated companies. MVP is a full-service insurance entity serving approximately 700,000 customers across Vermont and New York.
Ashok Chennuru, chief data and insight officer at Elevance Health, and Patrick Roohan, vice president of quality and clinical analytics at MVP Health Care, spoke to HealthITAnalytics via Zoom and email to discuss the organizations\u2019 data analytics strategies.
USING ANALYTICS TO DRIVE BETTER OUTCOMES FOR MEMBERS
Chennuru and Roohan both emphasized that their organizations\u2019 analytics approaches center on using data-driven insights to improve outcomes for their members.
However, this process requires that Elevance and MVP generate insights into their customers\u2019 needs, which comes with a variety of challenges.
Roohan highlighted that the COVID-19 pandemic, alongside the mental health and substance use epidemics, has created unprecedented hurdles, such as economic uncertainty. These make improving health outcomes more difficult.
To overcome these issues, MVP\u2019s strategy largely focuses on collaborating with healthcare providers, promoting preventive care, identifying care gaps, and managing chronic diseases. Much of these efforts aim to help educate members on why chronic disease management and preventive care are valuable.
Doing so requires that MVP gather and analyze significant data on its member populations, such as what conditions are prevalent in a community or how cultural differences may impact healthcare attitudes. These insights can then be extrapolated to identify unmet needs in a population.
By integrating both health and social factors data, MVP can work to flag the healthcare needs impacting certain members and contributing to negative patient outcomes.
\u201cPeople are not going to get preventive care when they don't have food, and they don't have housing,\u201d Roohan stated.
He explained that MVP has partnered with insurance company Belong Health to help integrate these data and connect members with resources to address these needs.
Currently, the two organizations are working together to help MVP\u2019s Dual Eligible Special Needs Plans (D-SNP) members access community health providers and resources to tackle persistent social and medical needs.
Elevance utilizes a similar strategy that involves using algorithms to personalize member experience.
In particular, the payer leverages predictive analytics to improve outcomes in areas such as diabetes management and fall risk.
\u201cWe know helping people with diabetes manage their chronic conditions involves encouraging them to monitor and better manage their A1C levels by obtaining regular tests,\u201d Chennuru said. \u201cTo enable this, it is important to identify individuals at risk of increased A1C level and/or unable to have the condition under control.\u201d
To do so, Elevance built a predictive model that uses various clinical indicators such as comorbidities, demographics, and previous A1C patterns to predict A1C trends for health plan members.
Chennuru indicated that using the tool, Elevance can segment members into moderate and high-risk categories for diabetes progression. Members predicted to have moderate A1C risk are then shipped an at-home lab A1C test kit.
For members flagged as having high A1C risk, their providers were notified, which allows care teams to better engage with the patient and encourage improved disease management. Throughout this campaign, Elevance has observed an increase in the use of A1C test kits.
The second predictive model is designed to identify women over 65 at risk of fracture due to a fall.
The algorithm incorporates a wide variety of data points, such as past diagnoses, procedures, and medications, in addition to factors like the presence of musculoskeletal-related conditions, connective tissue disorders, analgesic or opioid drug usage, and frailty indicators.
Elevance used the model as part of a pilot program for its Medicare members, during which individuals at high risk for fractures received outreach recommending that they undergo a bone density scan. According to Chennuru, the scan aimed to help members be better informed about their risk and take the necessary precautions.
\u201cThis approach helped our teams determine outreach to the right individuals who needed help and significantly improve the rate of preventive scans for engaged members,\u201d he explained. \u201cFor example, informed by data-driven insights, we have sent care teams to members\u2019 homes to check that their home environment does not present health concerns, such as items that increase fall risk, and help address any issues as appropriate.\u201d
Approaches like these are key to supporting value-based care and advancing person-centered care for at-risk populations, but data quality can make or break a population health initiative.
INTEGRATING HIGH-QUALITY DATA FROM MULTIPLE SOURCES
High-quality data is crucial to achieving whole-person care, which has piqued providers' and payers' interest in what role real-world data may play in improving outcomes.
To this end, Elevance uses a data quality management framework to help ensure high-quality analytics outputs are being generated.
\u201cPayers have to ingest, standardize, and cleanse/automatically correct hundreds of data elements with minimal delay to support analytic needs such as value-based care analytics, cost of care analytics, clinical analytics/care gaps, provider performance/quality analytics, and employer reporting,\u201d Chennuru stated.
This process requires Elevance to address the quality of data from internal payer administrative sources as well as external sources while adhering to data regulations, like HIPAA data privacy and security requirements.
\u201cThe quality of the analytic output is only as good as the underlying data, and payers across the industry face common challenges with low data quality as well as the scale of integration/standardization required to enable downstream analytics,\u201d Chennuru continued.
The payer\u2019s data quality management framework is designed to proactively identify and resolve issues with incorrect or incomplete data by measuring the quality score of data across different domains and continuously monitoring data for quality defects.
To do this, the tool compares Elevance data with reliable internal and external sources to identify accuracy issues and uses AI-driven checks to detect validity problems. Anomalies in the data are flagged as "dirty" records and undergo human review when necessary.
Elevance also employs automated processes to address incorrect data. These efforts not only enhance the accuracy of provider data but also improve the efficiency of AI-driven processes for matching consumers to providers, Chennuru noted.
MVP\u2019s data quality strategy involves collecting and analyzing various data across multiple high-quality sources. The payer relies on claims data, EMRs, social determinants of health (SDOH) data, health assessment data, and other sources, such as hemoglobin A1C control information, to evaluate customer care patterns and provide targeted support for members.
Some of these sources, like EMRs, are more limited than others, and MVP is actively working to obtain more of these data, Roohan noted. At the time of this interview, the payer has access to high-quality EMR data for approximately 20 percent of its members.
These data are then used to identify areas where MVP can support members, like the D-SNP population.
However, moving forward, MVP aims to expand its data sources and analytics beyond this population, intending to leverage data from Medicaid, Medicare, and commercial lines of business to address challenges across different customer segments.
PROVIDING TAILORED SUPPORT TO TACKLE PREVENTIVE CARE BARRIERS
Addressing care barriers impacting diverse customer segments necessitates tailored interventions based on that population\u2019s data.
Using its data sources, MVP found that many women it serves do not undergo regular mammography screening, which can lead to adverse health outcomes.
By utilizing a combination of advanced analytics and machine learning (ML), the payer is working to improve screening rates for its members.
Roohan explained that the analytics process first identifies two distinct groups: compliant women who don't require intervention and chronically non-compliant women in preventive care. From there, hypotheses are generated to identify the reasons behind non-compliance, such as transportation issues, work constraints, limited access to imaging centers, or daycare problems.
The insights gathered through this process are used to target specific women and address their unique barriers to mammography. The success of these efforts is evaluated by looking at how breast cancer rates change as a result of targeted interventions.
In addition, MVP also measures the effectiveness of its tailored interventions using key performance indicators based on 40 to 50 different metrics. These metrics are informed by benchmarks and standards outlined by the federal and state governments to rank health plan performance.
Roohan noted that this helps the payer provide a consumer-centric approach that prioritizes curiosity about what drives improved outcomes for members.
\u201cWhy is one group getting [mammographies] over another group? What do we know? What could possibly be the reason for that?\u201d Roohan asked to illustrate his point. \u201cIt's not as black and white as we all hope it to be, but that data is actually helping us to point to directions for quality improvement efforts, which we do with providers and members virtually every day.\u201d
Editor\u2019s Note: This article was updated to clarify that Elevance Health has predictive analytics programs outside of those used for diabetes management and fall risk at 10:30 am ET on July 6, 2023.