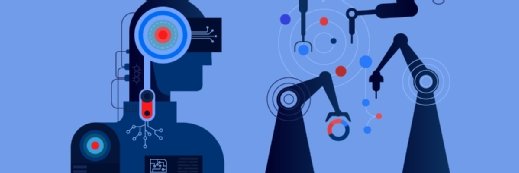
Getty Images
Generative AI may enhance healthcare-associated infection surveillance
Two AI-driven tools can accurately detect cases of central line-associated bloodstream infection and catheter-associated urinary tract infection.
Researchers from Saint Louis University and the University of Louisville School of Medicine have found that generative artificial intelligence (AI) could improve healthcare-associated infection (HAI) surveillance programs, according to a study published last week in in the American Journal of Infection Control.
HAIs remain a significant challenge as healthcare providers work to improve care delivery and patient safety.
The Centers for Disease Control and Prevention (CDC) indicates that roughly one in every 31 hospital patients has at least one HAI on any given day in the United States. In 2015, there were 687,000 HAIs reported in US acute care hospitals, and 72,000 affected patients died during their hospitalizations.
The research team emphasized that the implementation of infection prevention protocols – particularly infection surveillance programs – has reduced the incidence of HAIs considerably. Today, many healthcare organizations have put these programs into place, but some facilities lack the resources to do so.
Advanced technologies like AI have been proposed to help enhance infection surveillance programs, but research in this area is still relatively limited.
To address this, the researchers assessed two large language models (LLMs) – OpenAI’s ChatGPT Plus and an open-source model known as Mixtral 8x7B – based on their ability to identify central line-associated bloodstream infection (CLABSI) and catheter-associated urinary tract infection (CAUTI) in clinical scenarios of various complexities.
The six training scenarios were pulled from the National Health Care Safety Network dataset and fed to the models. The complexity of each scenario was analyzed, and the models were tasked with gauging whether each scenario description – which contained information on patient symptoms, age, date of admission, and dates for the insertion and removal of any central lines or catheters – represented a CLABSI or a CAUTI.
The LLMs' responses were compared to those of human experts to assess each model’s accuracy.
Both ChatGPT and Mixtral 8x7B accurately identified HAI across all six scenarios when given clear prompts. However, missing or ambiguous information – like use of special characters, lack of specificity, abbreviations and dates provided in numeric format rather than spelled out – found in the scenario descriptions limited the LLMs’ ability to generate correct responses.
“Our results are the first to demonstrate the power of AI-assisted HAI surveillance in the healthcare setting, but they also underscore the need for human oversight of this technology,” said lead author of the study Timothy L. Wiemken, PhD, MPH, an associate professor in the division of infectious diseases, allergy, and immunology at Saint Louis University, in a news release. “With the rapid evolution of the role of AI in medicine, our proof-of-concept study validates the need for continued development of AI tools with real-world patient data to support infection preventionists.”
The authors further emphasized that with ongoing user education and AI model refinement, HAI surveillance tools could free healthcare providers to focus on patient care.
“HAI surveillance is a critical responsibility for infection preventionists, and our community needs every possible tool to help us ensure the safety of our patients,” said Tania Bubb, PhD, RN, CIC, FAPIC, 2024 president of the Association for Professionals in Infection Control and Epidemiology (APIC). “This study suggests that AI-powered tools may offer a cost-effective means of improving our surveillance programs by assisting infection preventionists in day-to-day work functions.”
Infection surveillance is just one potential use case for generative AI in healthcare.
Researchers from NYU Langone Health recently described how the application of tools like ChatGPT could accelerate the development of digital health software.
Designing these interventions presents a challenge, as the software engineering process requires significant communication between technical and nontechnical team members.
The research team used ChatGPT to recreate a personalized automatic messaging system (PAMS) integrated into existing diabetes prevention software. Doing so allowed users to develop a new version of the tool in less than half the time needed to design the original.