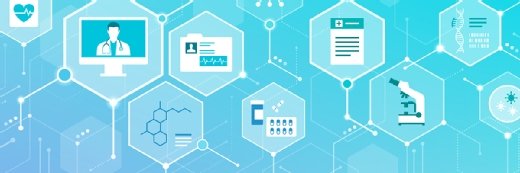
elenabs/istock via getty images
Computational model uses biomarkers to predict Alzheimer's progression
A personalized mathematical model analyzes data from brain scans, cerebrospinal fluid and memory tests to predict how an individual’s Alzheimer’s may progress.
Researchers from Duke University School of Medicine and Pennsylvania State University have demonstrated that a personalized model using individual biomarker data can accurately forecast Alzheimer's disease progression.
The tool, known as the Alzheimer’s Disease Biomarker Cascade (ADBC) model, is a computational model designed to leverage real-world data to predict the course of a patient’s disease. The research team indicated that currently, most mathematical models for Alzheimer's remain theoretical, relying on short-term changes at the cellular and molecular levels to shed light on progression patterns.
These models have significant potential to improve personalized medicine, as they can use clinically relevant parameters to evaluate individual data and shed light on disease drivers. These insights could then be used to assess the impact of potential treatment interventions.
However, the researchers noted that theoretical models are limited because they model Alzheimer’s at the molecular level over periods of minutes and hours, rather than long term.
To close this research gap, the team set out to investigate the feasibility of customizing a computational model to predict Alzheimer's progression using individual biomarkers.
Data from more than 800 participants with varying cognitive abilities were pulled from the Alzheimer Disease Neuroimaging Initiative (ADNI) to develop and test the model. From there, information from patient brain scans, cerebrospinal fluid analysis and memory tests were fed to the tool.
Using these data, ADBC successfully identified 14 personalized parameters in each participant, which reflected meaningful physiological characteristics – biomarker growth rates, carrying capacities and latency values – to inform predictions.
These parameters varied significantly among clinical diagnostic groups, indicating that characteristics of each biomarker may represent clinically relevant features of Alzheimer's progression.
“Alzheimer's disease has long been viewed as a single disorder,” explained Jeffrey R. Petrella, MD, a neuroradiologist and director of the Alzheimer’s Imaging Research Laboratory at the Duke University School of Medicine, in the press release. “This research shows that the disease progresses differently in each person, with unique patterns of biomarker changes.”
The model was also able to accurately forecast future biomarker trajectories, maintaining an average error rate of 9 percent across the study cohort.
The analysis further revealed two distinct ‘endophenotypes’ – groups of patients with differing underlying biomarker profiles and disease trajectories.
While the researchers emphasized that these findings need to be validated in larger, diverse populations, they underscored that the ADBC model could prove valuable for classifying Alzheimer’s progression more effectively and tailoring treatment regimens.
“I would envision using this model in clinical care as part of a precision medicine approach to treatment,” said Petrella. “The model could develop a recommendation of the optimal therapeutic regimen needed to help a patient achieve the best possible result over time while minimizing exposure to side effects.”
Other models have also shown promise in tracking Alzheimer's progression.
In February, a research team from the University of Texas at Arlington detailed the development of a predictive model to forecast how an Alzheimer's patient’s condition may deteriorate over time.
The researchers indicated that the loss of cognitive function present in Alzheimer's is a major cause of disability, often requiring increased caregiver support as the disease progresses. However, anticipating these additional care needs remains a challenge when existing models to predict progression are limited.
To address this, the research team built a learning-based “disease-embedding tree” (DETree) to flag where a patient is within the Alzheimer's disease-development spectrum. The tool combines Alzheimer’s progression modeling with individual prediction to code disease development into stages.
From there, the tool represents each clinical stage and its trajectory as a “tree” that a patient’s individual data can be projected onto to identify their clinical status and predict their Alzheimer’s progression.