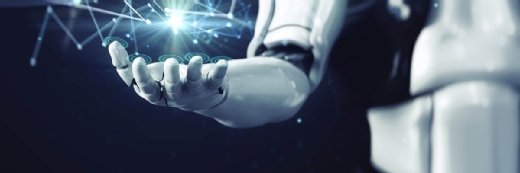
Getty Images
University of Massachusetts DL Model to Predict Alzheimer’s Onset
New NIH-funded project aims to spur the development of a deep learning tool for predicting Alzheimer's two or more years before symptom onset.
Researchers from the University of Massachusetts (UMass) Amherst have received a two-year, $278,118 grant from the National Institutes of Health (NIH) to build deep learning models for the early prediction of Alzheimer’s disease using clinical data.
Data from the Centers for Disease Control and Prevention (CDC) indicate that the public health burden of Alzheimer’s disease is steadily growing, impacting an estimated 6.7 million people in the United States alone. This number is expected to almost triple, impacting nearly 14 million people by 2060.
Currently, treatments for the disease are only effective in its early stages, but predicting Alzheimer's remains a challenge. The research underway at UMass Amherst aims to help close this gap by enabling the early detection of Alzheimer's – two or more years prior to the onset of symptoms – via deep learning.
By leveraging advanced analytics to forecast and identify risk of Alzheimer's development, the researchers hope that patients can take advantage of newly-developed interventions and medications to improve outcomes.
“This research brings us closer to putting people in clinical trials at a point where the brain biology is still intact and something can be done,” said Madalina Fiterau, PhD, assistant professor in the Manning College of Information and Computer Sciences at UMass Amherst and principal investigator of the study, in a news release. “Sixty percent of a patient’s brain matter disappears by the time of diagnosis, and at that stage it’s irretrievable. What we would like to do is identify those changes early, at least two years before onset, and then, based on that, figure out which treatments work.”
Early prediction of Alzheimer’s will better position researchers to investigate potential interventions to interrupt the course of the disease, the research team noted.
“We would not have been able to say this three years back, but now that many new drug candidates are emerging, we are at the point where forecasting techniques can actually be deployed to identify potential subjects for a disease-modifying therapy,” explained Joyita Dutta, PhD, the project’s other principal investigator and associate professor of biomedical engineering at UMass Amherst.
Previous studies have attempted to develop deep learning models to predict neurodegenerative disease, but these models have limited generalizability. Most utilize data from the Alzheimer’s Disease Neuroimaging Initiative (ADNI) dataset, but this results in the models using engineered data, such as average cortical thickness information generated through software, rather than actual images of a patient’s cerebral cortex.
“ADNI contains a vast set of specialized features that are extracted from the brain images that require a lot of feature engineering and domain expertise,” stated Fiterau. “Data collected in the wild is not going to have the specialized features. You’re going to have the [magnetic resonance imaging (MRI)] scans, but no annotation.”
“That’s the purpose of the grant: figuring out how to take a model that’s been trained on this specialized, carefully curated data set and see what its performance can be on real data collected in the wild,” she continued.
Doing so will allow predictive algorithms for Alzheimer’s to utilize standard MRIs instead of specialized data, which is more difficult to obtain.
“I work extensively with [positron emission tomography (PET)] scans for my research but not every clinic collects PET images of Alzheimer’s patients,” Dutta noted. “At the same time, MRI tends to be the go-to imaging modality for individuals with neurological complaints. However, clinically available MRI scans are often conducted using protocols that are different from ADNI. Predictive models, therefore, need to be generalizable to ‘data collected in the wild’ in order to be practically useful.”
To that end, the researchers will leverage deep learning to pull features from patients’ brain MRIs, which will then be used as proxies for specialized features present in the ADNI dataset. Key regions that are known to be significantly impacted by Alzheimer's – the cerebral cortex, the hippocampus, and the fluid-filled ventricle cavities – will be weighted within the model to accurately predict patients’ risk of the disease.
The research team also plans to address potential model biases created by demographic gaps in ADNI data, including the overrepresentation of highly educated populations and the underrepresentation of minoritized individuals.
This research is part of a broader effort in the healthcare industry to leverage advanced analytics to predict and flag the early onset of neurodegenerative diseases.
In a March interview with HealthITAnalytics, experts from Indiana University Health and the Davos Alzheimer’s Collaborative (DAC) discussed a recent collaborative pilot project aimed at improving the early detection of cognitive impairment in the primary care setting through digital screening and artificial intelligence (AI).