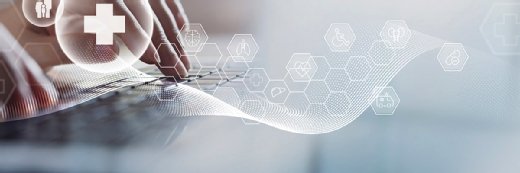
tippapatt - stock.adobe.com
NLP May Help Flag SDOH Needs for Alzheimer’s, Dementia Patients
Natural language processing tool uses unstructured EHR data to identify social determinants of health for patients with Alzheimer's disease and related dementias.
Researchers have developed a natural language processing (NLP) algorithm to identify social determinants of health (SDOH) for Alzheimer's disease and related dementias (ADRD) patients using unstructured EHR data, according to a study published this month in Health Services Research.
The model is designed to flag social determinants related to transportation, food, housing, financial difficulties, social isolation, abuse, neglect, or exploitation, and medication insecurities. The research team noted that SDOH are critical risk factors for adverse health events for ADRD patients.
However, important SDOH information is not systematically collected in many healthcare settings, but can often be found within unstructured EHR data. This makes accessing and leveraging this information more challenging.
To address this, the researchers proposed that NLP may have the potential to effectively extract this information for use in the clinical setting. By automatically extracting these data, the research team indicated that the NLP tool may help clinicians, social workers, and case managers to better identify and take action on patients’ SDOH needs and prevent adverse outcomes.
To develop the model, the researchers pulled 1,000 randomly selected medical notes from 7,401 inpatient social worker and emergency department writeups created between 2015 and 2019 for 231 ADRD patients at Michigan Medicine, the University of Michigan’s academic medical center.
From there, the research team developed a rule-based NLP algorithm and trained it to identify the seven domains of social determinants listed above.
The model was then compared to a regularized logistic regression approach and a deep learning model trained to accomplish the same task. The three tools were compared using accuracy, sensitivity, specificity, F1 score, and the area under the receiver operating characteristic curve.
For each tool, 700 of the medical notes pulled for the study were leveraged for training, while the remaining 300 were reserved for model validation.
Using the 700 training notes, the F1 and AUC for the NLP algorithm were at least 0.94 and 0.95 across all SDOH categories. Using the 300 validation notes, F1 and AUC were at least 0.80 and 0.97 for all SDOH domains except housing and medication insecurities.
The regularized logistic regression and deep learning algorithms both had low performance, with the NLP model significantly outperforming both.
These findings may indicate that NLP models have the potential to help flag SDOH and improve outcomes for patients, but more research is needed.
For now, the researchers will work to prospectively validate the NLP model against an SDOH questionnaire recently deployed within Michigan Medicine’s primary care settings. In doing so, the research team hopes to compare the algorithm’s findings to patients’ responses, which may improve the tool’s performance.
“We are also preparing a pilot program that will evaluate the feasibility of an intervention that addresses these social determinants of health, and connect identified people with community resources,” said Elham Mahmoudi, PhD, a health economist at Michigan Medicine who helped lead the study, in a press release detailing the findings. “In the meantime, we hope our current results show that this algorithm can be used by clinicians, case managers, and social workers to proactively address the social needs of patients with dementia, and potentially other vulnerable patient populations.”