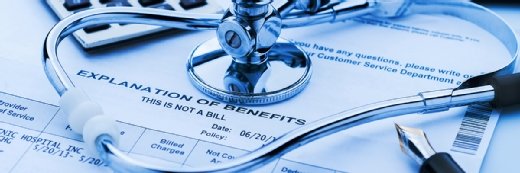
Christian Delbert - stock.adobe.
Machine Learning Identifies Drug Candidates for Cardiac Fibrosis
Mechanistic machine learning tools can provide insights into how different drug candidates could minimize harmful scarring following a heart attack.
University of Virginia (UVA) researchers have developed a machine learning tool to identify factors associated with cardiac fibrosis and predict which drug candidates can help prevent the condition.
Cardiac fibrosis is characterized by abnormal scarring of the heart muscles following a cardiac event or injury, such as a heart attack. This excessive scarring can cause cardiac dysfunction and contribute to adverse patient outcomes.
Anti-fibrotic drugs have the potential to prevent some of this scarring, but identifying disease drivers and understanding how drugs impact them remains a challenge for researchers.
“Many common diseases such as heart disease, metabolic disease and cancer are complex and hard to treat,” said Anders R. Nelson, PhD, a former postdoctoral research scientist at UVA School of Medicine who contributed to the study, in the news release. “Machine learning helps us reduce this complexity, identify the most important factors that contribute to disease and better understand how drugs can modify diseased cells.”
Previous research aimed at identifying drug targets for cardiac fibrosis has focused only on particular behaviors of fibroblasts, the cells that produce the collagen needed to repair heart damage. This process causes harmful scarring in some patients, but not in others.
To better understand these cells and how anti-fibrotic drug candidates affect them, the research team used machine learning to explore which phenotypic features of fibroblasts change in response to treatment.
The approach identified 137 of these features in relation to 13 anti-fibrotic drugs. Using this data, the researchers trained machine learning algorithms to predict each drug’s effect on the cells and their behavior.
The analysis revealed Src inhibition via the phosphoinositide 3-kinase (PI3K) pathway as a potential therapy to treat cardiac fibrosis.
“Bridging machine learning with human learning helped us not only predict drugs against fibrosis, but also explain how they work. This knowledge is needed to design clinical trials and identify potential side effects,” stated corresponding author Jeffrey J. Saucerman, PhD, a professor in UVA’s Department of Biomedical Engineering, a joint program of the School of Medicine and School of Engineering.
The research team cautioned that more work is needed to ensure that these drug candidates work as intended, but noted that mechanistic machine learning tools may be particularly useful for analyzing cause-and-effect in other biological applications.
The researchers also indicated that their model has the potential to help predict the impact of drugs on other diseases, as well.
“We hope this provides an example of how machine learning and human learning can work together to not only discover, but also understand how new drugs work,” Saucerman said.
Machine learning is also being applied in other clinical applications for cardiovascular disease.
Researchers from The Texas Heart Institute recently detailed the development of a machine learning tool designed to characterize and predict diuretic responsiveness in patients with acute decompensated heart failure (ADHF).
The condition is the result of excessive fluid accumulation in the body and is a significant cause of adverse outcomes. Treatment relies on using diuretic drugs to alleviate heart congestion, but treating patients who are resistant to these drugs is a major hurdle for clinicians.
For these patients, it is recommended that care teams optimize the dosage of diuretics before proceeding to combination therapy. To improve this process, the researchers built a machine learning tool that classifies patients into subgroups based on their diuretic efficiency, which could help provide clinical decision support and improve outcomes.