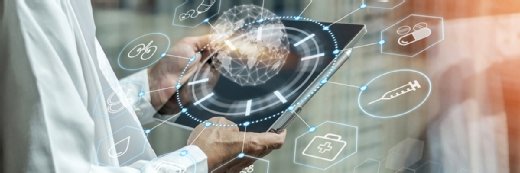
Chinnapong - stock.adobe.com
Publicly Trained LLMs Identify Musculoskeletal Pain Location, Acuity
Publicly trained large language models can accurately flag both the pain location and acuity of musculoskeletal disorders, like lower back, knee, and shoulder pain.
Mount Sinai researchers have demonstrated that publicly trained large language models (LLMs) can identify the pain location and pain location of musculoskeletal conditions like shoulder, knee, and lower back pain, according to a study published last week in The Lancet Digital Health.
Complex musculoskeletal conditions can be challenging to manage in the clinical setting. The research team indicated that the use of novel, publicly trained LLMs could reduce existing barriers to the use of artificial intelligence (AI) at the point of care in clinical settings, allowing clinicians to improve care quality and delivery.
To test this hypothesis in the context of musculoskeletal care, the researchers gathered 26,551 patient notes from five Mount Sinai facilities from November 16, 2016, to May 30, 2019.
From this sample, expert clinicians manually labeled 1,714 notes from 1,155 patients for pain location and acuity. The final dataset was comprised of notes from multiple sources, with 19 percent received from primary care, 51 percent from internal medicine, and 30 percent from orthopedics.
Labels were developed based on the location—shoulders, lower back, knee, or “other”—and the acuity—acute, chronic, and acute-on-chronic—of pain.
This information was used to fine-tune a publicly available foundational language model known as LLaMA-7B. Another LLaMA-7B model was also trained using a combination of the study dataset and the Alpaca dataset, which contains over 50,000 general-purpose instructions paired with expected responses.
The research team then used a method called group shuffle splitting to partition the data into 75 percent training, 5 percent validation, and 20 percent testing groups.
The LLaMA-7B model trained only on the study dataset achieved classification accuracies of 0.89 for shoulder pain, 0.94 for lower back pain, 0.90 for knee pain, and 0.98 for other pain locations.
The LLaMA-7B model trained with the extended dataset demonstrated slightly better accuracy for shoulder pain at 0.93, but performed similarly or slightly worse across the other categories.
However, both LLaMA-7B models mostly outperformed baseline models in terms of sensitivity. The exception was knee pain, in which the Longformer model achieved a sensitivity of 0.94.
The LLMs also accurately categorized pain acuity.
The LLaMA-7B model trained only on patient notes achieved classification accuracies of 0.83 for acute pain, 0.83 for chronic pain, and 0.82 for acute-on-chronic pain.
The LLaMA-7B model trained on the extended dataset demonstrated lower performance across acuities, with classification accuracies of 0.80 for acute pain, 0.81 for chronic pain, and 0.79 for acute-on-chronic pain.
LLaMA-7B also outperformed baseline models across all metrics with the exception of the BERT model, which achieved a sensitivity of 0.63 for acute pain.
“Our findings indicate that pre-trained large language models can serve as a robust foundation for creating fine-tuned models capable of effectively parsing unstructured clinical notes in a directed manner,” explained Ismail Nabeel, MD, MPH an associate professor in the Department of Environmental Medicine and Public Health at the Icahn School of Medicine at Mount Sinai, in a media advisory shared with HealthITAnalytics via email. “Such models can be deployed as specialized conversational agents or chatbots, helping clinicians swiftly access pertinent patients, maintain data privacy, and potentially streamline clinical workflow.”
The research team further indicated that the study shows the promise of publicly trained LLMs in medicine.
"This study demonstrates that we're not restricted to the limited knowledge inside pre-existing large language models,” said the study’s first author Akhil Vaid, MD, an instructor in the Department of Medicine at Icahn School of Medicine. “We can supplement this knowledge through task-specific fine-tuning, and utilize it to derive specific bits of information present within complex, unstructured clinical text."