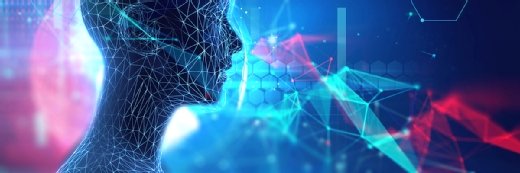
Getty Images
Deep Learning Approach May Reduce Knee Pain Disparities
A deep learning technique used to measure the severity of knee osteoarthritis could significantly reduce unexplained pain disparities.
A deep learning tool may be able to analyze medical images and dramatically reduce pain disparities in underserved populations, a study published in Nature Medicine revealed.
In society today, pain is prevalent but unequally distributed. Researchers noted that knee osteoarthritis, which affects ten percent of men and 13 percent of women over 60 in the US, disproportionately impacts underserved populations. People of color score much higher than knee pain scales than white individuals, according to the research team.
There are two possible explanations for these disparities: underserved patients may have more severe osteoarthritis within the knee, or external factors – like life stress or social isolation – cause or exacerbate knee pain among underserved populations.
Providers typically classify the severity of osteoarthritis using a standard called the Kellgren-Lawrence grade (KLG), a decades-old method developed in white British populations. This standard may miss physical causes of pain in people of color, researchers said. Additionally, the team pointed out that there are known racial and socioeconomic biases in how a patient’s pain is perceived by observers.
“If the pain experienced by underserved populations is caused by objective factors missing from current measures, a range of painful, treatable knee ailments would be misattributed to factors external to the knee,” the team stated.
Researchers set out to discriminate between the two potential explanations for disparities in knee pain: clinical factors within the knee and factors external to the knee. For the study, the team used a diverse sample of 4,172 US patients who had or were at high risk of developing knee osteoarthritis.
Patients also reported a knee-specific pain score, called a Knee injury and Osteoarthritis Outcome Score (KOOS), derived from a survey on pain experienced during various activities.
The group found similar pain disparities across socioeconomic groups. Forty-three percent of lower-income patients and 45 percent of lower-education patients had severe pain, versus 38 percent of patients overall.
Black patients also had more severe osteoarthritis, with 56 percent having KLGs higher than two compared to 46 percent of patients overall. However, this higher disease severity did not fully account for the higher pain levels experienced by black patients.
The results showed that the racial disparity in pain was 10.6 KOOS points without controlling for any severity measures, compared to 9.7 points when controlling for KLG. This means that KLG only accounted for just nine percent of the pain disparity. Results also showed that the KLG accounted for only 16 percent of the pain disparity by income, and just eight percent of the disparity by education.
“These results suggest that objective osteoarthritis severity does not account for a large proportion of the pain disparity between racial and socioeconomic groups,” researchers stated.
“However, this judgment is dependent on the objective measure used (in this case, KLG), which could incorporate a range of inaccuracies.”
The team sought to develop an alternative measure for osteoarthritis severity. Researchers used a dataset of 25,049 x-ray images to train a deep learning tool to predict the reported pain score for each knee.
The resulting measure, indicated by algorithm pain prediction (ALG-P), summarizes the objective features present in the x-ray images that predict pain. The group found that this new measure’s ability to predict pain was at least as good as that of the KLG measure.
The findings revealed that this new measure of severity can better account for disparities in osteoarthritis pain compared to the standard KLG method. ALG-P accounted for 43 percent of the racial pain disparity, 4.7 times more than KLG did. The new method also accounted for 2.0 times more of the disparity by income, and 3.6 times more of the disparity by education.
The team believes that a key advantage of the new deep learning method is that it learned from a diverse dataset, 20 percent of which was made up of black patients as well as many lower-income and lower-education patients.
While there is a real concern that AI could further exacerbate health disparities, the study suggests that diversifying datasets is a critical method to ensure quality care for all patient populations.
The study also has significant implications regarding which patients receive surgery for knee pain, as patients with higher KLGs are usually more likely to undergo surgery.
“We hypothesize that underserved patients with disabling pain but without severe radiographic disease could be less likely to receive surgical treatments and more likely to be offered non-specific therapies for pain,” the group said.
“This approach could lead to overuse of pharmacological remedies, including opioids, for underserved patients and contribute to the well-documented disparities in access to knee arthroplasty.”
The researchers expect that their new deep learning method has the potential to provide helpful clinical decision support for providers in real-world care settings.
“One promising option for integrating our algorithm into clinical practice is to use it as a decision aid, rather than as a replacement, for human clinicians (for example, by showing the clinician a heatmap of affected regions within the knee alongside the ALG-P score),” researchers concluded.
“Such cooperation between humans and algorithms was shown to improve clinical decision making in some settings, although this approach is not without challenges, such as physicians potentially placing incorrect levels of weight on algorithmic predictions. More broadly, our results illustrate how algorithms can be used to identify and reduce disparities in healthcare.”