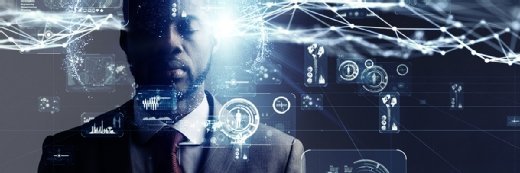
Getty Images
Deep Learning Could Improve Cohort Selection for Clinical Trials
Deep learning technology could identify key features for cohort selection in clinical trials, significantly reducing time and costs.
Deep learning models showed promising results in learning key features for clinical trial cohort selection, which could significantly reduce time and costs, according to a study published in JAMIA.
In biomedical research, a cohort is a group of patients who share a set of similar characteristics for a specific study, researchers explained. In order for research studies and clinical trials to be successful, researchers have to quickly and accurately choose cohort patients, which can be a daunting task.
“The cohort definition is a very time-consuming task because of the large number of patient records that have to be manually reviewed by the researchers. This process is a very challenging problem due to multiple variations of how the information is recorded, medical coding mistakes, sparse data, or missing details, among other issues,” the team said.
“Thus, a robust cohort definition requires careful reading of the patient records in order not to miss potential subjects for the study.”
Past research has shown that artificial intelligence and other advanced analytics tools have the potential to help automate processes in clinical trials, leading to more cost-effective research.
The team set out to test different deep learning models for the task of cohort selection, including a simple convolutional neural network (CNN), a deep CNN, a recurrent neural network (RNN), and a hybrid model combining both CNN and RNN. Researchers trained the models on 311 patient records manually labeled by experts to indicate whether a patient meets a possible criterion from a list of 13 criteria.
The team found that of all the models, the RNN and hybrid algorithms provided the best overall results, while the simple CNN and deep CNN provided slightly lower overall results. All models show low performance for minor criteria like alcohol abuse and drug abuse, which researchers said could be attributed to the high imbalance between their positive and negative instances.
These results have significant implications for clinical trial cohort selection, the team noted.
“The success of epidemiological studies and clinical trials depends on the selection of the right patients. The medical researchers must perform this selection carefully by the careful analysis of an enormous amount of information from different sources,” researchers said.
“This process is an expensive and time-consuming task. Rules-based methods or machine learning classifiers exploiting the ICD-9 codes related to the selection criteria can be applied to alleviate the burden of medical researchers in the cohort selection.”
Researchers pointed out that a major limitation of the study was the small size of the dataset. Future research should leverage larger datasets to improve the performance of the deep learning models. Additionally, the team will examine whether deep learning models can identify dependencies in text labels.
“We plan to explore semisupervised deep learning techniques to overcome the lack of a sufficient number of training examples,” the team concluded.
“A notable shortcoming of the classical approaches for multilabel text classification is that labels are considered as independent units. However, they usually can present strong dependencies among them, especially in the context of clinical trials, in which some patient conditions can be strongly related. Therefore, we also plan to perform a study about if deep learning methods can detect the label dependencies.”