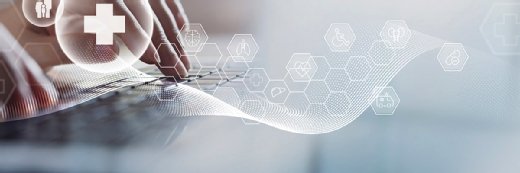
tippapatt - stock.adobe.com
In Brain Imaging, Deep Learning Beats Standard Machine Learning
Deep learning models were able to detect patterns and discriminative features in brain imaging better than standard machine learning algorithms.
Properly trained deep learning models could offer better insights from brain imaging data analysis than standard machine learning approaches, according to a study published in Nature Communications.
Structural and functional MRI and genomic sequencing have generated massive volumes of data about the human body. Scientists can gather new insights into health and disease by extracting patterns from this information. However, this is a challenging task as the data is incredibly complex and relationships among types of data are poorly understood.
Deep learning technology can characterize these relationships by combining and analyzing data from many sources. While these algorithms have demonstrated their ability to solve problems and answer questions in several different fields, researchers noted that critical commentaries have negatively compared deep learning with standard machine learning approaches for analyzing brain imaging data.
But these conclusions are often based on pre-processed input that deny deep learning the ability to learn from data with little to no preprocessing – one of the main advantages of the technology.
A team from the Center for Translational Research in Neuroimaging and Data Science (TReNDS) leveraged deep learning to better understand how mental illness and other disorders affect the brain.
Researchers compared representative models from classical machine learning and deep learning, and found that if trained properly, deep learning methods could potentially offer significantly better results, producing superior representations for characterizing the human brain.
“We compared these models side-by-side, observing statistical protocols so everything is apples to apples. And we show that deep learning models perform better, as expected,” said co-author Sergey Plis, director of machine learning at TReNDS and associate professor of computer science.
Researchers did acknowledge that there are some cases where standard machine learning performs better than deep learning. Diagnostic algorithms that plug in single-number measurements, like patient’s body temperature or whether a patient smokes cigarettes, would work better using classical machine learning approaches.
“If your application involves analyzing images or if it involves a large array of data that can’t really be distilled into a simple measurement without losing information, deep learning can help,” Plis said. “These models are made for really complex problems that require bringing in a lot of experience and intuition.”
The disadvantage of deep learning models is that they need to be trained on a lot of data at the outset. But once these models are trained, they can effectively analyze massive amounts of complex information as well as answer simple questions.
“Interestingly, in our study we looked at sample sizes from 100 to 10,000 and in all cases the deep learning approaches were doing better,” said Vince Calhoun, director of TReNDS and Distinguished University Professor of Psychology.
Another advantage of deep learning is that scientists can reverse analyze deep learning models to understand how they reach conclusions about data. In the case of the current study, the trained deep learning models learned to identify meaningful brain biomarkers.
“These models are learning on their own, so we can uncover the defining characteristics that they’re looking into that allows them to be accurate,” said Anees Abrol, research scientist at TReNDS and the lead author on the paper.
“We can check the data points a model is analyzing and then compare it to the literature to see what the model has found outside of where we told it to look.”
The team believes that deep learning models are capable of extracting explanations and representations not already known to the field and help in expanding knowledge about how the human brain functions. Researchers said that further investigation is necessary to find and address the weaknesses of deep learning models.
Still, the group maintains that from a mathematical point of view, it’s clear these models outperform standard machine learning tools in many settings.
“Deep learning’s promise perhaps still outweighs its current usefulness to neuroimaging, but we are seeing a lot of real potential for these techniques,” Plis said.
A separate study recently published in Nature Medicine also demonstrated deep learning’s potential to improve imaging analysis. The team showed that a deep learning model may be able to detect breast cancer one to two years earlier than standard clinical methods.
“Our results point to the clinical utility of AI for mammography in facilitating earlier breast cancer detection, as well as an ability to develop AI with similar benefits for other medical imaging applications. We have developed an approach that mimics how humans often learn by progressively training the AI models on more difficult tasks,” said lead author Bill Lotter, PhD, CTO, and co-founder of DeepHealth.
“By leveraging prior information learned in each successive training stage, this strategy results in AI that detects cancer accurately while also relying less on highly-annotated data. Our approach and validation extend to 3D mammography, which is particularly important given its growing use and the significant challenges it presents for AI.”
As is the case with most AI-based tools in healthcare, deep learning still has some challenges to overcome before it can be used in real-world clinical settings – but the technology has certainly proven its potential for the future of care delivery.