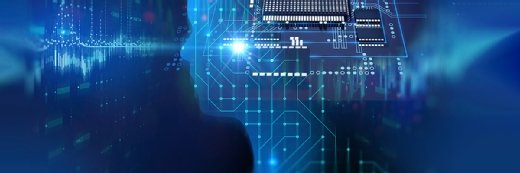
Getty Images
Top Challenges of Applying Artificial Intelligence to Medical Imaging
Medical imaging is one of the best use cases for artificial intelligence in healthcare, but lack of clinician input and data bottlenecks can make the technology less helpful than promised.
Among the many possible applications of artificial intelligence and machine learning in healthcare, medical imaging is perhaps the most promising.
When used to decode the complicated nature of MRIs, CT scans, and other testing modalities, advanced analytics tools have demonstrated their ability to extract meaningful information for enhanced decision-making – sometimes with greater precision than humans themselves.
From improved cancer detection to faster pneumonia diagnoses, AI and machine learning have proven to be viable companion tools in the fields of radiology and pathology.
“A lot of the major advances we've seen in AI have focused on image analysis,” said Walter Wiggins, MD, PhD, assistant professor of radiology at Duke University and clinical director of the Duke Center for Artificial Intelligence in Radiology.
“There is so much information contained in medical images that we haven’t even come close to tapping into through traditional methods of interpretation.”
While AI has seemingly limitless potential to gather new insights from medical images, implementing the technology in clinical settings comes with some major barriers.
The very complexities that make medical imaging an ideal domain for analytics algorithms are the same intricacies that can hinder AI use. Researchers and developers need massive amounts of imaging data to successfully train AI models and ensure they’re ready to be deployed in routine care.
Even after implementation, health systems have to consistently monitor the performance of these tools, as well as verify that providers are using the technologies to effectively enhance care delivery.
As the industry moves forward on its long march toward automation, researchers and provider organizations are working to find solutions to these challenges – an endeavor that will eventually lead to improved clinical performance and better patient care.
Collaborating for better algorithms and better insights
When designing AI models for healthcare, it’s essential that developers build intuitive tools that can easily integrate with clinical workflows.
However, solutions are often built without the input of clinicians, resulting in convoluted technologies that can cause more harm than good.
To facilitate the design of clinically-friendly AI algorithms, radiology residents at Brigham and Women’s Hospital recently developed a data science pathway to introduce fourth-year radiology residents to advanced analytics tools.
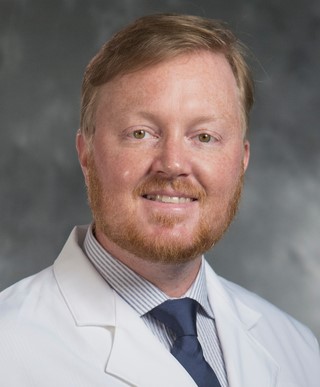
In addition to broadening the availability of organized AI and machine learning curricula, the pathway gives students the opportunity to contribute to every stage of algorithm development.
“We decided to develop this pathway because we saw a need for more formal training of radiologists who were interested in AI, and we wanted to better facilitate collaborations between radiologists and data scientists,” said Wiggins.
“The idea was, if we can better speak the other side's language, we might be able to help them develop models that are designed with a specific clinical question in mind.”
A key goal of the pathway is to ensure that developers are keeping clinical implementation in mind throughout the entire design process, Wiggins explained.
“That was one of the biggest problems we were trying to address, because it seemed like there were a lot of algorithms out on the market that were developed in a vacuum. We wanted to try to match the clinical need with the technology in a way that allows for the development of solutions that can seamlessly integrate into the radiology clinical workflow,” he said.
The partnerships between radiologists and data scientists can also better prepare clinicians for potential issues that could arise in real-world situations.
“If you're going to have doctors using these tools in practice, they need to understand a little bit about how they work, particularly where they can go wrong and where they can fail,” said Wiggins.
"You want to be able to understand the potential sources of bias in AI training data, and how to monitor and mitigate those biases. Providers also need to know how to handle errors that AI may or may not produce in clinical practice."
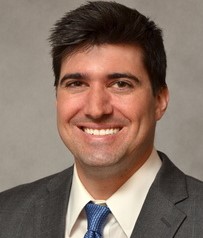
Similar partnerships have emerged across the healthcare industry. Recently, Amazon Web Services and the Pittsburgh Health Data Alliance (PHDA) announced that they would support researchers from the University of Pittsburgh Medical Center (UPMC), the University of Pittsburgh, and Carnegie Mellon University in using machine learning techniques to improve breast cancer screening.
The team – made up of experts in computer vision, bioinformatics, and breast cancer imaging – will develop machine learning models to analyze mammograms and forecast the short-term risk of developing breast cancer.
“The project is focused on doing risk prediction in patients with breast cancer by a combined imaging modality of both pathology and radiology images,” said Michael Becich, MD, PhD, associate vice chancellor for health informatics and health sciences at the University of Pittsburgh.
“Amazon saw a great opportunity to accelerate this effort by offering their robust platform for doing the computation that's needed for these very large imaging data sets.”
A team from the University of Minnesota (U of M) also recently partnered with an industry giant to advance analytics in medical imaging. In a collaboration with Epic, researchers developed an AI algorithm that can evaluate x-rays to diagnose possible cases of COVID-19.
The model will be available at no cost to other health systems through Epic, with 12 M Health Fairview hospitals currently using the new AI tool.
“We were able to obtain a small grant to support all of our computer science researchers’ efforts on this project, which really helped to get this going. The timeline of what it would've taken to launch this was greatly accelerated in response to the pandemic,” said Christopher Tignanelli, MD, assistant professor of surgery at the University of Minnesota Medical School.
After validating the algorithm, U of M researchers worked with Epic to build the infrastructure around the AI model so that it could seamlessly and immediately translate the algorithm’s results into medical record software.
“We started focusing on how we could give the U of M team a good platform on which to run the algorithm. We want to bring these predictions and scores to clinicians and nurses in the ED within seconds to minutes after these images are captured,” said Drew McCombs, a software developer at Epic.
“We’re also looking at building an easy way for them to be able to share this algorithm with other groups. So, making sure that we build our core pieces of the platform that allow them to take their model and easily deploy it across different sites.”
According to Tignanelli, a project like this wouldn’t be possible without cross-sector partnerships and collaboration.
“It really does take a large multidisciplinary team to develop these algorithms and implement them, and an effort like this should include specialists in computer science and informatics, clinical content, and implementation science,” he said.
Identifying – and overcoming – key challenges
Much like the hurdles that accompany the use of analytics tools in any area of care, the issues that can come with AI in medical imaging often stem from healthcare’s greatest bottleneck: data.
“Most of the challenges in AI are not technical applications of AI,” said Becich.
“There's a robust number of algorithms, particularly causal discovery algorithms, and other advanced machine learning models that continue to grow. However, most of their validation and implementation in medical practice are handicapped by access to large, high-quality datasets.”
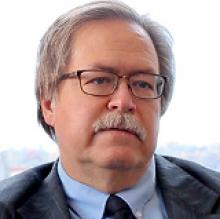
Recent efforts have aimed to increase the availability of data for analytics algorithms. In 2019, a team from MIT developed an automated system that can gather more data from images used to train machine learning models. The method could accelerate the process of collecting training data for machine learning algorithms, speeding up diagnosis and treatment time.
While accessing large volumes of quality data is a significant challenge, it’s only part of the battle, Becich noted.
“We also have to find a way to allow all of the imaging work that occurs in a multi-dimensional network of practices to flow into commercial and innovation activities. You have to create the pipeline, the data lake, the warehousing, and the regulatory and compliance frameworks that are needed to make imaging AI real and easily accessible to the development community,” said Becich.
“That’s what the PHDA aims to do – to make the flow of data robust so that developers building these AI applications can allow for not just the right kind of validation and proof, but also transparency and reproducibility in a variety of clinical practices.”
Tignanelli and his team learned the value of reproducibility and generalizability when developing their own AI tool.
“Initially, we wanted to develop two algorithms: A general population algorithm and a pneumonia algorithm. The pneumonia algorithm would essentially identify COVID-19 pneumonia versus other pneumonias – viral, bacterial, fungal. The general would just be COVID-19 versus everything else,” Tignanelli explained.
“When we went to validate the pneumonia algorithm, we realized that it's not really generalizable. We couldn't find a good use case for it. We ended up scrapping that altogether, and we decided we wanted to develop the most generalizable algorithm possible. So, that essentially made the general population algorithm move up to the forefront.”
However, the process doesn’t end after a health system develops and deploys an AI algorithm. Leaders should continuously monitor the performance of analytics tools to make sure they’re providing clinicians with actionable, meaningful insights.
“If an organization implements a new scanner model or upgrades its software, the image data could change in some way. This could potentially throw off an AI algorithm that was trained prior to that change,” Wiggins said.
“The big question is going to be, how do we monitor for those types of changes? How do we analyze the performance either in big cohorts just at an institution level, or look at data from each scanner to make sure that the algorithm running on that data is producing reliable results?”
This will be particularly critical when the industry starts implementing AI models that don’t require as much human involvement, Wiggins added.
“If there's bias or a systematic error, a radiologist may not be able to pick up on it right away,” he said.
“Organizations should have some sort of automated or semi-automated system in place that can flag studies for potential problems, as well as evaluate how well the algorithm is performing versus its historical baseline.”
In the case of U of M and Epic, researchers have recognized the importance of continuously monitoring algorithms, both before and after deployment.
“In our study, we had to consider varying prevalence rates. How do we ensure that the algorithm will perform as prevalence changes?” said Tignanelli.
“We made sure to test against a range of different prevalence levels, and we're going to do a real-time validation once this algorithm does go live.”
Picturing the future of imaging analytics
Looking ahead, healthcare experts see a bright future for AI and medical imaging. As the technology continues to advance, and researchers continue to refine these tools, AI and machine learning have the potential to enhance all aspects of imaging analysis.
“There are several roles that AI could play in medical imaging going forward,” said Wiggins.
“There are use cases where AI is meant to provide automated analysis for triaging and studies to make sure that we’re getting to the studies that are most likely to contain critical findings. Other AI technologies are aiming to try to enhance the quality of images that we're getting so that we can either reduce scan times or reduce radiation dose, depending on which imaging modality we're talking about, which could be good for patients as well.”
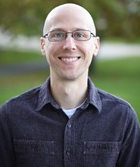
Becich also noted that AI and machine learning tools will have important implications beyond care delivery.
“There is an extremely rich set of data resources around imaging – particularly radiology and pathology imaging – not just for clinical practice, but also for discovery research,” said Becich.
“With AI applied to imaging both in radiology, which has been well-established with its digital platforms for a couple decades now, as well as pathology, we're heralding a new wave of applications to make practices more efficient and more personalized.”
Additionally, with new research being conducted regularly, the development and implementation processes will only improve.
“This is just the tip of the iceberg. We've already made a large list of additional use cases and we're expanding our infrastructure and our footprint in this space. We're obtaining a larger graphics processing unit server. We've tripled the size of our team from start to now, and significantly extended our radiology team,” said Tignanelli.
“Now that the first pathway is already done, we anticipate that the process of building more and better algorithms should be much quicker.”
The prevalence of cross-sector partnerships is also playing a major role in the advancement of imaging analytics technologies, McCombs stated.
“We're making sure that the folks who are using these algorithms are part of the early phase as well as the designing approach for the tools. We want the algorithms to take into account the real-time nature of the clinical care setting, so that providers could take that algorithm and be sure that it can effectively offer clinical decision support,” he said.
A field as complex and intricate as medical imaging is certainly well-suited for the capabilities of AI and machine learning. With the right data, expertise, and use cases in place, the healthcare industry could be in a position to enhance clinical decision-making and improve patient care.
“Between the advances in image analysis and data analytics, radiology and pathology are very ripe for embracing AI,” Wiggins concluded.
“There are a lot of insights that stand to be derived from medical images, and AI can help facilitate that.”