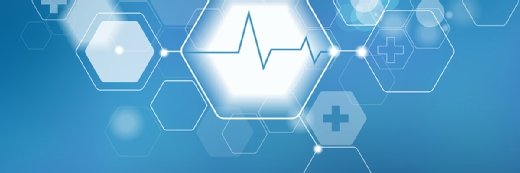
AlfaOlga/istock via Getty Images
Apple Watch, ML Can Predict Pain in Sickle Cell Disease Patients
Researchers used data collected from Apple Watches and machine learning analyses to predict pain in people with sickle cell disease.
In a paper published earlier this month in JMIR Formative Research, researchers explored the feasibility of using the Apple Watch to predict pain scores in hospitalized sickle cell disease patients and leveraging these scores to build machine-learning (ML) algorithms to predict the pain scores associated with vaso-occlusive crises (VOCs).
According to the Centers for Disease Control and Prevention (CDC), sickle cell disease refers to inherited red blood cell disorders characterized by abnormal hemoglobin, resulting in hard, sticky, C-shaped red blood cells. The disease is associated with potentially severe complications, such as stroke, blood clots, anemia, infection, vision loss, and pain.
In this study, the researchers also noted that VOCs are a serious complication and the leading cause of hospitalization for individuals with sickle cell disease. The Indiana Hemophilia & Thrombosis Center indicates that VOCs often present as acute pain syndromes in the emergency department settings, but they can be difficult to diagnose and treat because there are no laboratory tests or clinical findings for the indication of VOCs.
Instead, proxies or tests to rule out other non-VOC complications are performed, including blood or fluid cultures, X-rays, or evaluations of baseline hemoglobin. These challenges with diagnosing and treating VOCs have led some researchers to consider using mobile health technologies and algorithms to predict pain in people with sickle cell disease, the study authors stated.
In their study, the researchers sought to combine the data collection abilities of a consumer wearable, such as the Apple Watch, with ML techniques to better understand the pain experience and predict pain trends in sickle cell disease patients presenting with VOCs.
To do this, the research team approached patients with sickle cell disease older than 18 admitted to Duke University SCD Day Hospital for a VOC between July 2021 and September 2021 to participate in the study. In total, 20 patients, all of whom identified as Black or African American, were enrolled.
Study participants were given an Apple Watch Series 3 to be worn for the duration of their hospital visit, which was attached to each patient’s wrist and set to “other” exercise mode to gather continuous heart rate, heart rate variability, and calorie data.
Vital sign and pain score data were pulled from patient EMRs and analyzed using three ML models: multinomial logistic regression, gradient boosting, and random forest. Two null models, which used no biometric measures in their prediction and predicted pain scores based on the frequency of the scores in the training set, were also included to assess the accuracy of pain scores.
During data collection, a total of 15,683 data points across the study population were gathered. Each participant spent an average of two hours and 17 minutes wearing the Apple Watch. The ML models were trained with half of the dataset, while the other half was used for testing.
Model performance was measured in terms of accuracy, which was quantified via F1-score, area under the receiving operating characteristic curve, and root-mean-square error (RMSE). Overall, the ML models outperformed the null models, with the random forest model achieving the best performance, predicting pain scores with an accuracy of 84.5 percent and an RMSE of 0.84.
The researchers concluded that these results validate the feasibility of using noninvasive data from a wearable device to predict pain scores during VOCs. This method is a low-cost approach that could benefit clinicians and sickle cell disease patients during VOC treatment, they stated.