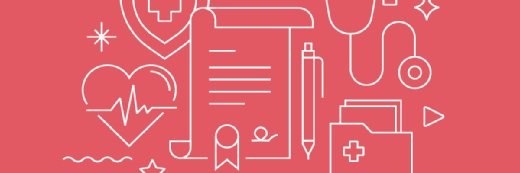
cnythzl/DigitalVision Vectors vi
AI Could Safely Automate Some X-ray Interpretation
Researchers found that the interpretation of 7.8 percent of all radiographs in a recent study could potentially be safely automated by artificial intelligence.
In a study published this week in Radiology, researchers sought to investigate the clinical impact of autonomous radiograph reporting using a commercially available artificial intelligence (AI) tool and determined that the interpretation of up to 7.8 percent of radiographs in the dataset could be safely automated.
To evaluate the tool’s clinical impact, the research team looked at the number of chest radiographs it autonomously reported, the sensitivity of its detection of abnormal chest radiographs, and its performance compared with that of clinical radiology reports.
The study cohort comprised 1,529 adult patients from four hospitals in Denmark. Consecutive posteroanterior chest radiographs and other data were obtained for these patients in January 2020. Images were collected from outpatients, in-hospital patients, and emergency department patients.
Using these data, three thoracic radiologists were tasked with labeling each image as abnormal or normal, with abnormal radiographs being subcategorized as “abnormal, critical,” “abnormal, other remarkable,” and “abnormal, unremarkable.”
The researchers defined normal as a chest radiograph with no abnormal findings, including any chronic changes and/or anatomic variance. Definitions for the abnormal subgroups were outlined by the research team as follows: “critical” findings may have an immediate patient impact and should always be reported; “other remarkable” findings would rarely, if ever, have a direct patient impact but should always be reported; “unremarkable” findings have no patient impact and are not necessary to report.
The AI tool classified each radiograph as either high confidence normal (normal) or not high confidence normal (abnormal).
The thoracic radiologists reviewing the images had access to radiology reports and the de-identified radiologic and medical history of each patient in the cohort — including any previous or subsequent CT scans, chest radiographs, or other images, if available — but were blinded to the AI’s results. The AI software is designed to analyze raw Digital Imaging and Communications in Medicine (DICOM) images and use frontal and lateral views of posteroanterior chest radiographs to generate classifications, but it cannot process previous chest radiographs.
Of the 1,529 patients in the cohort, 1,100, or 72 percent, were classified by the reference standard as having abnormal radiographs. Of these, 617, or 40 percent, were labeled critical abnormal radiographs, and 429, or 28 percent, were classified as normal radiographs.
The AI tool achieved a sensitivity of 99.1 percent for abnormal radiographs, indicating that it was able to correctly identify the presence of abnormalities in 1,090 of 1,100 patients’ images, and a 99.8 percent sensitivity for critical radiographs, flagging all but one of the patients in that subgroup.
The radiologists’ reports achieved sensitivities of 72.3 percent and 93.5 percent for the same groups, respectively.
Looking more broadly at all the normal posteroanterior chest radiographs, the AI achieved a specificity of 28 percent. This means that the interpretation of up to 28 percent of normal posteroanterior chest radiographs, or 7.8 percent of all radiographs, could potentially be safely automated by the tool, the researchers explained.
This rate, however, varies by referral setting and could be in the range of 6.2 to 11.6 percent, with the highest rate being achieved in the outpatient group.
Despite these promising results, the researchers highlighted that the study aimed to assess whether the sensitivity of the AI tool was inferior to that of current standards. They noted that comparing the diagnostic accuracy of radiologists and AI is not meaningful, even if they were to achieve similar performance, as the AI is optimized to minimize false negatives, while radiologists must consider sensitivity, specificity, and other information to draw conclusions in the clinical setting.
Further research in this area could be directed toward larger prospective implementation studies in which all autonomously reported chest radiographs were still checked by radiologists to ensure patient safety, the research team stated.