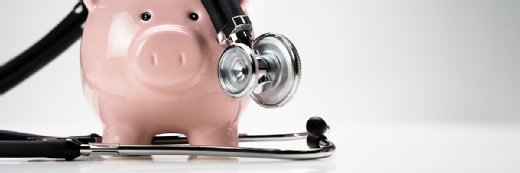
AndreyPopov/istock via Getty Ima
Deep-Learning Mammography Models at Risk of Poor Generalizability
Researchers have found that a previously validated ensemble of models for mammography displayed a decline in performance when applied to a diverse patient population.
A study published last month in JAMA Network Open found that a previously validated, high-performing ensemble of deep-learning (DL) models for automated mammography interpretation did not generalize to a diverse US patient population and showed a decline in performance for some subgroups.
According to the study, a lack of fellowship-trained breast radiologists has led mammography screening programs to turn to artificial intelligence (AI) tools to increase diagnostic accuracy and efficiency in breast cancer screening. However, for these tools to be used effectively, they must be externally validated to show how they perform in various practice settings with different patient cohorts.
This study set out to externally validate one such tool, an ensemble learning model combining the 11 highest-performing individual AI models from the Digital Mammography Dialogue on Reverse Engineering Assessment and Methods (DREAM) Mammography Challenge.
The DREAM Challenge is one of the largest crowdsourced efforts in mammography AI development and leveraged 144,231 screening mammograms from Kaiser Permanente Washington (KPW) for algorithm training and internal validation, the study states. In this cohort, the model was associated with improved overall diagnostic accuracy in combination with radiologist assessment. The model achieved similar performance when it was externally validated on a Swedish cohort from the Karolinska Institute (KI).
However, the study authors noted that both the KPW and KI cohorts were composed heavily of White women and that the model had not yet been externally validated on a more diverse US population. Thus, the researchers set out to validate the model using retrospective patient data collected between 2010 and 2020 from 26,817 women, 40 and older. The women underwent 37,317 routine breast screening examinations and participated in the Athena Breast Health Network at the University of California, Los Angeles (UCLA).
To evaluate the performance of the challenge ensemble method (CEM) from the DREAM Challenge, the researchers compared its performance against the original radiologist reader performance at the time of screening, as well as the performance of the CEM and radiologist combined (CEM+R). To measure performance, they focused on sensitivity, specificity, and area under the receiver operating characteristic curve (AUROC).
To generate its outputs, the CEM model leveraged the confidence scores generated by each of the 11 algorithms. These scores, which were between zero and one to reflect the likelihood of cancer for each side of the breast, were then used as inputs for the CEM and reweighted to output a combined score.
AUROC estimates for the 11 individual models ranged from 0.77 to 0.83, and the CEM model achieved an AUROC of 0.85 in the UCLA cohort. However, this was significantly lower than the AUROCs achieved in the KPW and KI cohorts, which were 0.90 and 0.92, respectively.
The CEM+R model achieved a sensitivity and specificity similar to the radiologist’s original performance but had significantly lower sensitivity and specificity than the radiologist with regard to women with a prior history of breast cancer and Hispanic women.
The drop in performance when applied to these subgroups suggests that the model experienced underspecification, which can be characterized as a gap between the requirements developers have in mind when they build a model and the requirements that are actually enforced by the model’s design and implementation.
Here, underspecification of the model resulted from its narrow training and validation cohorts, which in turn led to a lack of generalizability when applied to a broad, more diverse population.
The authors concluded that these findings indicate the need for more model transparency and fine-tuning of models to specific target populations before their clinical adoption to prevent underspecification and poor generalizability.