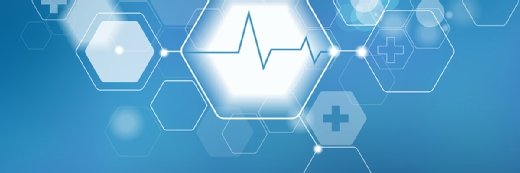
AlfaOlga/istock via Getty Images
AI Model Can Accurately Detect Multiple Retinal Diseases in Real Time
A new study shows that a deep-learning algorithm can recognize multiple retinal abnormalities simultaneously, which could help improve the early diagnosis of retinal diseases in underdeveloped areas.
New research has found that a deep-learning (DL) algorithm can detect multiple retinal diseases simultaneously in a clinical setting.
In a study published in JAMA Network Open, researchers developed and validated a DL model that can detect retinal abnormalities using ocular fundus images. Then, they evaluated whether the model could be effectively applied in a clinical setting.
The researchers noted that retinal and optic nerve diseases are one of the most common causes of irreversible vision loss worldwide. Diagnosis of these diseases requires the availability of experienced ophthalmologists. However, the distribution of these clinicians varies from region to region, with underdeveloped areas typically having little access to ophthalmologists who can screen individuals for signs of retinal disease.
To combat this challenge, the researchers suggest implementing AI-based methods that would establish cost-effective and reliable access to screenings in underdeveloped areas. To develop their model, Retinal Artificial Intelligence Diagnosis System (RAIDS), the researchers retrospectively collected 120,002 ocular fundus images from ten iKang Health Care centers and the Beijing Tongren Hospital between June 2018 and June 2020.
These images were then assessed by three random examiners from a pool of 40 certified ophthalmologists and assigned a retinal disease diagnosis. The diagnosis was final if two of the three examiners agreed. If two examiners couldn’t reach an agreement, the image was reassessed by a panel of six senior experts. These images were then separated into a training set and a validation set and given to a multitask convolutional neural network (CNN).
To validate the clinical applicability of RAIDS, the researchers conducted an investigation leveraging 65 examination centers in 19 Chinese provinces. In each center, RAIDS was implemented through the cloud and connected to the ocular fundus cameras. Study participants then underwent fundus photography performed by trained operators, and any resulting images that were deemed nonassessable by operators — because of blur or defocus — were excluded from further analysis. The images were then analyzed by RAIDS and the experts.
The external validation for the model followed a similar setup, and the performances of RAIDS and nine ophthalmologists were compared. Overall, RAIDS achieved an accuracy higher than or equal to those of the retinal specialists in seven of the 10 retinal diseases being evaluated, including AMD, retinal vein occlusions, macular holes, epiretinal macular membrane, hypertensive retinopathy, myelinated fibers, and retinitis pigmentosa. In terms of speed, RAIDS assessed each image in less than a second compared to the top speed of the retinal specialists, which was 7.68 seconds.
When used on its own, RAIDS achieved a 95 percent reduction in exam time versus ophthalmologists working alone. When paired with an ophthalmologist, RAIDS helped reduce exam time by 75 percent.
The researchers suggested that the success of RAIDS could help solve problems associated with lower screening rates and early detection of retinal disease in underdeveloped regions. Since it can be used alone or with an ophthalmologist, the researchers stated that RAIDS could potentially be used in both local hospitals and reading centers.