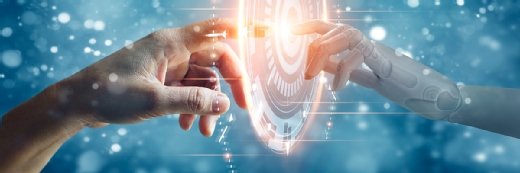
Getty Images
4 Strategies for Addressing, Avoiding AI Algorithmic Bias in Healthcare
The Center of Applied AI at Chicago Booth’s playbook recommends assessing risk for AI algorithmic bias and continuously monitoring.
AI algorithmic bias is everywhere, according to the Center for Applied AI at Chicago Booth in their recently released playbook. Through working with dozens of organizations such as healthcare providers, insurers, technology companies, and regulators, the center states that algorithmic bias is found all throughout the healthcare industry. These biases influence clinical care, operational workflows, and policy.
These algorithms are put in place to help decision makers determine who needs resources. The idea is if two people are scored the same using the algorithm, then they will have the same basic needs. This method is supposed to assist in making a more equitable and efficient method of care. According to the Center for Applied AI at Chicago Booth, the color of an individual’s skin or other sensitive attributes should not matter when determining need, and algorithms that fail this test are biased.
There are reasons behind the algorithmic bias. The first reason could be that an organization tried to hit the correct target but excludes those that are underserved in the population. This could be due to researchers being trained or evaluated in non-diverse populations.
Algorithms may also aim at the wrong target altogether.
According to the playbook, there are four steps that companies can take to keep algorithmic bias out of their organizations.
The first step is to take inventory of the algorithms. Developers should speak with stakeholders to learn about how and when algorithms are being used. Once the algorithms have been identified, appoint an individual to monitor the algorithms, especially among diverse groups.
The second step identifies the key to addressing algorithmic bias; be as specific as possible, the playbook authors recommend. Make should you understand exactly what the target of the algorithm should be.
Once there is that understanding, it is a lot easier to ensure the algorithm is hitting the current target. It is recommended that organizations fill out a table screening for label choice bias. Using the table, organizations will fill out what the algorithm is, the ideal target, the actual target, and the risk of bias.
Step three includes updating any inefficient algorithms or throwing them out if they are not serving the company well.
Finally, researchers should keep monitoring the algorithms and conduct audits to ensure they are reaching the target goals.
“While we have certainly made progress in understanding algorithmic bias, we all still have more to learn. This playbook is intended to be a living document, and we will update it as the field develops,” the playbook concludes.