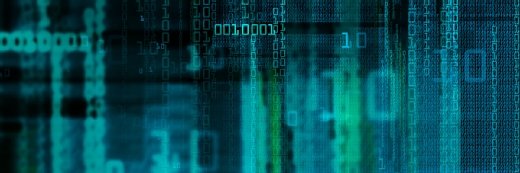
Getty Images
EHR Data Quality Guidelines Needed to Drive Interoperability
The healthcare industry lacks standard EHR data quality assessment guidelines and tools, which hinders interoperability.
Guidelines are needed for EHR data quality assessment (DQA) to improve the efficiency, transparency, comparability, and interoperability of data quality assessment, according to a study published in JAMIA.
Researchers extended a 2013 literature review on EHR data quality assessment approaches and tools to determine changes in DQA methodologies. The 2013 literature review established five dimensions of EHR data quality:
- Completeness (the presence of data in the EHR)
- Correctness (the truthfulness of data in the EHR)
- Concordance (the agreement between elements within the EHR and between the EHR and other data sources)
- Plausibility (the extent to which EHR data make sense in a larger medical context)
- Currency (the accuracy of the EHR data for the time at which it was recorded and how up-to-date the data are)
The researchers reviewed 103 papers published between 2013 and April 2023.
Since 2013, they found a general increase in the number of dimensions assessed per paper. The most common data quality dimension was completeness, followed by correctness, concordance, plausibility, and currency.
The review also revealed the addition of two dimensions to the data quality assessment framework: conformance and bias. Eighteen papers (17 percent) assessed EHR data quality conformance, defined as compliance with a predefined representational structure.
Eleven papers (11 percent) assessed bias. The researchers defined bias as a data quality dimension referring to missingness not at random.
“For example, some authors identified the pattern that sicker patients have higher levels of data completeness which implies that exclusion based on complete records will select a biased sample in terms of patient health levels,” the study authors wrote.
“Additionally, some authors highlighted the differences in data availability from structured versus unstructured data and suggested the bias resulting from using only one of the forms of EHR data,” they continued. “Differential recording of patient attributes by race also constituted an example of bias.”
Biased EHR data may negatively impact patient care when machine learning models are used in clinical decision support tools.
“Despite the consistent patterns of DQA in the literature found by this review, researchers largely developed DQA on a project-by-project basis,” the authors noted. “The methods used to assess data quality were repeatedly implemented across many applications although assessing a consistent collection of dimensions.”
“The repetitive patterns of DQA are not practical in terms of time and resources in our current research environment as EHR data continues to be commonly used for downstream analysis,” they continued. “For this reason, we recommend and highlight the emerging theme of DQA automation as discussed in many of the opinion pieces and tools.”
In addition to automation, the study authors said stakeholders should consider the balance between scalable and task-specific tools.
“As data requirements differ between systems and projects, we will need a flexible tool in order to be able to assess data quality across many applications,” they wrote. “One potential solution to this problem is the usage of a CDM to support interoperability and enable the development of reusable DQA tools.”