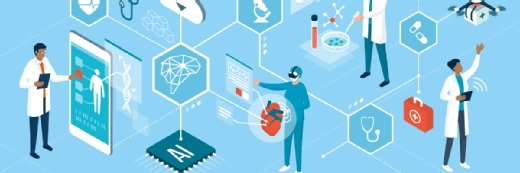
Getty Images
Wearable Biosensor Predicts Aggression Among Inpatients with Autism
The wearable device collects physiological data that can be analyzed via machine learning to preempt aggressive behavior among inpatient youth with autism.
Physiological changes recorded by a wearable biosensor and analyzed through a machine-learning approach can help predict aggressive behavior before it occurs in young psychiatric facility patients with autism, new research shows.
The study published in JAMA Network Open last month by Northeastern University researchers adds to research examining whether imminent aggressive behavior among autistic inpatients can be determined via a wearable biosensor and machine learning.
About one in 36 children were diagnosed with autism spectrum disorder (ASD) in 2020, up from one in 44 in 2018, according to the Centers for Disease Control and Prevention’s (CDC) Autism and Developmental Disabilities Monitoring (ADDM) Network. The prevalence of aggression among children and adolescents with ASD is high, with parents reporting in a 2011 study that 68 percent had demonstrated aggression to a caregiver and 49 percent to non-caregivers.
Prior research work by the Northeastern University team showed that three minutes of wearable biosensor-recorded peripheral physiological and motion signals gathered from 20 youths with autism could predict aggression toward others one minute before it occurred using ridge-regularized logistic regression.
The new study aimed to extend that research to determine whether the recorded data could be used to predict aggression toward others even earlier.
The researchers enrolled 86 participants at four primary care psychiatric inpatient hospitals. The participants had confirmed diagnoses of autism and exhibited self-injurious behavior, emotion dysregulation, or aggression toward others.
The research team collected patient data from March 2019 to March 2020. They coded aggressive behavior in real time while study participants wore a commercially available biosensor that recorded peripheral physiological signals, including cardiovascular activity, electrodermal activity, and motion. Of the 86 enrolled participants, only 70 were included in the analysis. The excluded eight could not wear the biosensor due to tactile sensitivity and general behavioral noncompliance or were discharged before an observation could be made.
During the study period, researchers collected 429 independent naturalistic observational coding sessions totaling 497 hours. They observed 6,665 aggressive behaviors, comprising 3,983 episodes of self-injurious behavior, 2,063 episodes of emotion dysregulation, and 619 episodes of aggression toward others.
Researchers conducted time-series feature extraction and data preprocessing, after which they used ridge-regularized logistic regression, support vector machines, neural networks, and domain adaptation to analyze the extracted time-series features to make binary aggressive behavior predictions.
They found that logistic regression was the best-performing overall classifier across eight experiments conducted. The classifier was able to predict aggressive behavior three minutes before it occurred with a mean area under the receiver operating characteristic curve of 0.80.
“Our results suggest that biosensor data and machine learning have the potential to redress an intractable problem for a sizable segment of the autism population who are understudied and underserved,” the researchers concluded. “Our findings may lay the groundwork for developing just-in-time adaptive intervention mobile health systems that may enable new opportunities for preemptive intervention.”
This is the latest instance of an mHealth tool that can be used to support care for youth with autism.
In September, Atlanta-based researchers announced they had developed a biomarker-based, eye-tracking diagnostic tool to diagnose ASD. The technology includes a portable tablet on which children watch videos of social interaction. The device monitors their "looking behavior" to pinpoint the social information the children are and are not looking at, according to the press release.
Clinicians review the data collected by the device and provide children and their families with a diagnosis and measures of the child's individual abilities, including social disability, verbal ability, and non-verbal learning skills.
Additionally, a University of California, Davis researcher received a five-year, $3.2 million grant from the National Institutes of Health (NIH) to study whether an ASD diagnosis among infants can be assessed effectively via telehealth.
The researcher, Meagan Talbott, Ph.D., and her team will enroll 120 infants between the ages of 6 and 12 months showing signs of delays or differences in their development. They will conduct four telehealth sessions over a year as well as additional assessments when the child is 3 years old to determine whether telehealth can help pinpoint possible ASD.