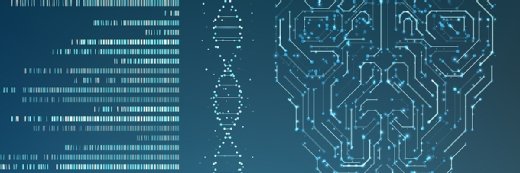
Nobi_Prizue/istock via Getty Ima
How to Improve Data Normalization in Healthcare
Data normalization is critical for healthcare interoperability, but how can organizations address challenges to normalizing their data and improve the process in the future?
Data normalization refers to the process of standardizing data to reduce ambiguity and make the data useable across systems. In the context of healthcare, health information is normalized to promote data sharing and analytics across the care continuum. This makes data normalization key to advancing interoperability and health information exchange (HIE).
Interoperability is defined in the 21st Century Cures Act as health information technology that "(A) enables the secure exchange of electronic health information with, and use of electronic health information from, other health information technology without special effort on the part of the user; (B) allows for complete access, exchange, and use of all electronically accessible health information for authorized use under applicable State or Federal law;” and “(C) does not constitute information blocking as defined in section 3022(a)."
Interoperable health systems are able to share information with one another easily and securely while also improving patient outcomes and reducing costs.
How is healthcare data normalized?
Healthcare data normalization begins when patient records are collected from various sources, including EHRs, lab systems, billing systems, insurance providers, and pharmacies. The data must be taken from these records, standardized, and then integrated into a data warehouse or repository.
What challenges do healthcare systems face with data normalization?
Normalizing healthcare data presents multiple challenges for providers.
The fact that health data comes from multiple different entities indicates that there may be differences in how the data is coded or presented. This issue is particularly salient for records and systems created before the development and adoption of interoperability standards.
However, lack of standardized codes and common language across disparate systems is one of the most significant issues for data normalization across the board. Differences in code systems could lead to the same information being represented more than once or to a piece of information being lost or not represented at all.
Health systems must also implement a data normalization infrastructure, either in-house or via a third party. Both options have various costs associated with them, but hurdles related to skilled resources and data processing are some of the largest.
For a health system to create a robust in-house data normalization system, it would need a team of highly skilled software engineers, health informaticists, and clinicians to provide support. Data engineers would also need to be hired in order to set up data processing pipelines. These individuals would all need to be paid, in addition to the expenses incurred to ensure that the system is effective.
To make sure the system is effective, stakeholders must consider factors like data quality, storage, and import. Data quality can impact extract, transfer, load (ETL) processes, which, if overused, can create more expenses. Late binding, the process of storing variations of data without a standardized code framework, makes importing the data easier, but it complicates the cleansing, processing, and reporting of that data.
A third-party data normalization platform would outsource some of these concerns, but such a system could present higher upfront costs.
Data privacy and security are also concerns for health systems engaged in data normalization and interoperability. The ability to share health information across systems can improve patient outcomes, but it can also present more opportunities for that information to become compromised.
To prevent this, those involved in data normalization and sharing must keep data security considerations at the forefront of their interoperability efforts, especially those at organizations responsible for compliance with HIPAA and HITECH.
How can healthcare systems improve data normalization?
Refer to the ISA and standardize across your system first
There are a handful of interoperability rules that providers and payers must comply with, such as the CMS Interoperability and Patient Access final rule, but outside of these, systems have some freedom with regard to how they code and standardize their healthcare data.
However, this doesn’t mean that providers need to come up with their own coding schema. ONC’s Interoperability Standards Advisory (ISA) Reference Edition lists standards and implementation specifications that can be used for clinical health IT interoperability. Any updates to the Reference Edition are posted online on a rolling basis so that interested parties can stay up to date.
After reviewing the Reference Edition, interoperability and data management personnel can use it to standardize data coding across the various departments within the health system as a first step toward becoming fully interoperable. Implementing standards across the system before trying to address data sharing across networks will help ensure that data is accurately codified and allow for any issues to be addressed early in the process. Additionally, if a majority of organizations adopt ISA standards, both data normalization and meaningful data sharing will become easier.
Before implementation, organizations should verify that they are adhering to any applicable federal, state, and local laws, in addition to any program regulations or other requirements regarding the use of a specific standard or specification, as these supersede the ISA.
Implement data integrity strategies
In addition to implementing coding standards within a health system before sharing between systems, ensuring data integrity within the system is also key to improving data normalization.
EHRs allow a patient’s record to follow them across the care continuum, potentially improving care and clinician decision making. However, data entry inconsistencies and mistakes can result in incorrect or missing information being integrated into a patient’s EHR.
Many of these data integrity missteps have the potential to cause patient harm, so having data integrity strategies in place for common issues like patient matching and identification is crucial. Poor data standardization can also lead to incomplete and inaccurate data collection, which harms data normalization efforts by limiting that data’s useability. Poor data quality that can result from data integrity issues can also impact the useability of normalized data.
Research suggests that implementing a data completeness tracking system (CTX) may help improve EHR data integrity. After developing the CTX, the researchers deployed across six care sites within the Accessible Research Commons for Health (ARCH) collaborative for a year. Then, they conducted a set of semi-structured interviews and a useability test with users and data curation leadership across participating sites.
The users reported that the CTX had increased their capacity to notice data completeness issues and empowered them to get involved in improving the quality of the data within their repositories.
Similar research has found that strong health data governance also improves data integrity. By utilizing governance best practices, providers were able to improve EHR data quality, use, and exchange while improving patient safety, interoperability, and clinical efficiency.
Consider creating unified patient records
Health systems will often have multiple “data islands” that contain information related to just one patient, according to Sriram Bharadwaj, vice president of digital innovation and applications at Franciscan Health, in a conversation with HealthITAnalytics.
Bharadwaj further explained that improved patient care requires that data islands be bridged so that information can be pulled together and accessed at the point of care. This requirement led Franciscan Health to implement unified patient records, which are the result of connecting non-aggregated data islands for a patient across the continuum of care.
Unified patient records are useful for supporting whole-person care, but they also compliment data normalization efforts. Franciscan Health’s approach invites health system employees to be part of the implementation process, encouraging them to point out problems and propose solutions with unified patient record “pods.” Involving employees, as in the CTX study, empowered them to become engaged in the data management process.
The creation of unified patient records would also require an effort to standardize data within Franciscan Health’s system first, which is an important initial step toward meaningful data normalization and sharing in the future.