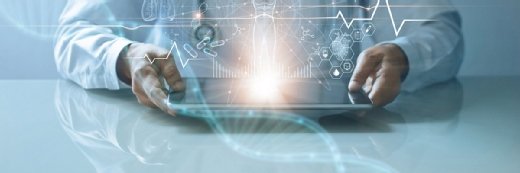
Getty Images/iStockphoto
AI Tool Uses Epigenetic Factors to Predict Cancer Survival Outcomes
By assessing gene expression patterns of epigenetic factors, an artificial intelligence model can predict patient outcomes across multiple cancer types.
Researchers from the UCLA Health Jonsson Comprehensive Cancer Center have created an artificial intelligence (AI) model that can successfully predict cancer survival outcomes by analyzing epigenetic factors, according to a recent study published in Communications Biology.
By investigating the gene expression patterns of epigenetic factors — those that influence how genes are turned on or off — in tumors, the research team found that each factor could then be categorized into groups. These insights can then be incorporated into an AI tool and used to predict cancer outcomes across cancer types better than some standard clinical measures, such as cancer stage and grade.
The researchers further noted that the study could help lay the foundation for developing targeted cancer therapies designed to regulate epigenetic factors, like histone acetyltransferases and Switch/Sucrose non-fermenting (SWI/SNF) chromatin remodelers.
“Traditionally, cancer has been viewed as primarily a result of genetic mutations within oncogenes or tumor suppressors,” said co-senior author Hilary Coller, PhD, professor of molecular, cell, and developmental biology and a member of the UCLA Health Jonsson Comprehensive Cancer Center and the Eli and Edythe Broad Center of Regenerative Medicine and Stem Cell Research at UCLA, in a press release.
“However, the emergence of advanced next-generation sequencing technologies has made more people realize that the state of the chromatin and the levels of epigenetic factors that maintain this state are important for cancer and cancer progression,” she continued. “There are different aspects of the state of the chromatin — like whether the histone proteins are modified, or whether the nucleic acid bases of the DNA contain extra methyl groups — that can affect cancer outcomes. Understanding these differences between tumors could help us learn more about why some patients respond differently to treatments and why their outcomes vary.”
The research team emphasized that previous research has shown that mutations present in genes that encode epigenetic factors can influence someone’s cancer susceptibility. However, how these factors impact cancer progression is still not well understood.
To begin bridging this knowledge gap and learn more about how epigenetics influence patient outcomes, the researchers evaluated the expression patterns of 720 epigenetic factors to classify tumors from 24 distinct cancer types into clusters.
Of those cancer types, the research team found that for ten cancers, those clusters were associated with variations in patient outcomes, such as overall survival, disease-specific survival, and progression-free survival.
These differences were particularly significant for all survival measurements in cases of liver hepatocellular carcinoma and lung adenocarcinoma, brain lower grade glioma, kidney renal clear cell carcinoma, and adrenocortical carcinoma.
Clusters that were associated with poor outcomes were also associated with larger tumor size, higher cancer stage, or more severe spread indicators.
The researchers then took the epigenetic factor gene expression levels and used them to develop an AI model to predict patient outcomes for the five cancer types that presented significant differences in survival measurements.
The tool was successfully able to sort patients with these cancers into two groups: one with a higher chance of poorer outcomes, and another with significantly higher odds of better outcomes.
The research team also found that the genes most important for the AI’s analysis had significant overlap with those that defined the clusters the team had previously identified.
Moving forward, the researchers suggested that the AI should be tested on other, independent datasets to assess its broader applicability.
“Our research helps provide a roadmap for similar AI models that can be generated through publicly-available lists of prognostic epigenetic factors,” said the study’s first author, Michael Cheng, a graduate student in the Bioinformatics Interdepartmental Program at UCLA. “The roadmap demonstrates how to identify certain influential factors in different types of cancer and contains exciting potential for predicting specific targets for cancer treatment.”
These findings are the latest to highlight how AI may improve cancer care in the future.
Last week, researchers from University of Florida (UF) Health showed that combining liquid chromatography-high resolution mass spectrometry (LC-HRMS) with machine learning (ML) could make brain tumor evaluations more efficient.
The research sought to generate insights into how these tools can refine the metabolomic and lipidomic characterization of meningioma tumors, as choosing a treatment approach can be challenging depending on the tumor’s grade.
To address this, the researchers built an ML model that can evaluate meningioma tumors with significant accuracy more quickly than a clinician.