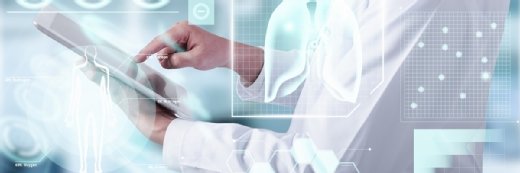
Getty Images
Deep Learning Tool May Support Personalized Cancer Care
Johns Hopkins researchers have developed a deep learning method to predict cancer-related protein fragments that trigger tumor cell-killing immune responses.
Researchers have developed a deep learning (DL) model capable of predicting cancer-related protein fragments that may elicit an immune response, which may aid the advancement of personalized cancer therapies.
The team, comprised of investigators from the Johns Hopkins Institute for Computational Medicine, the Johns Hopkins Kimmel Cancer Center, Johns Hopkins Biomedical Engineering, and the Bloomberg-Kimmel Institute for Cancer Immunotherapy, described the tool in a recent Nature Machine Intelligence study.
The tool, known as BigMHC, is designed to predict and identify protein fragments on cancer cells that have the potential to trigger a tumor cell-killing immune response. In doing so, the model may help researchers gain insights into immunotherapy response.
“Cancer immunotherapy is designed to activate a patient’s immune system to destroy cancer cells,” explained Rachel Karchin, PhD, professor of biomedical engineering, oncology, and computer science, and a core member of the Institute for Computational Medicine, in the press release. “A critical step in the process is immune system recognition of cancer cells through T cell binding to cancer-specific protein fragments on the cell surface.”
The protein fragments that trigger this response are sometimes the result of mutations or other changes in the genetic makeup of a cancer cell. These mutation-associated neoantigens are present in each patient’s tumor and determine the tumor’s unique makeup.
The press release notes that researchers can analyze the genome of a tumor to identify which of these neoantigens it has. This process helps determine which neoantigens are most likely to elicit a tumor-killing immune response, which researchers could use to develop personalized immunotherapies and cancer vaccines.
However, the current methods available to flag these neoantigens are often costly and time-consuming, requiring labor-intensive wet laboratory experiments. These difficulties have limited the neoantigen validation data available to researchers, curbing innovations in the area.
To combat this, the researchers developed BigMHC, a deep neural network-based method for neoantigen validation. The model was trained in a two-step process via transfer learning.
In the first step, BigMHC was taught to identify antigens on the cell surface. These antigens present at an early stage of immune response, making data about them easier to obtain and add to datasets. Using these large datasets as the basis of the model’s learning, the research team then trained BigMHC in T-cell recognition, for which fewer data are available.
By training the model on antigen presentation, the researchers could “transfer” the model’s knowledge to immunogenic antigen prediction without the need for a large amount of data.
BigMHC significantly outperformed four other prediction models for antigen presentation and seven other models for identifying immunogenic neoantigens.
“There is an urgent, unmet clinical need to tailor cancer immunotherapy to the subset of patients most likely to benefit, and BigMHC can shed light into cancer features that drive tumor foreignness, thus triggering an effective anti-tumor immune response,” said study co-author Valsamo “Elsa” Anagnostou, MD, PhD, director of the thoracic oncology biorepository, leader of the Johns Hopkins Molecular Tumor Board and Precision Oncology Analytics, and associate professor of oncology in the Kimmel Cancer Center.
Moving forward, the team will work to test BigMHC in multiple immunotherapy clinical trials in an effort to gauge its ability to help researchers sift through thousands of neoantigens to determine which are most likely to trigger an immune response.
“The hope is that BigMHC could guide cancer immunologists as they develop immunotherapies that can be used for multiple patients, or develop personalized vaccines that would boost a patient’s immune response to kill their cancer cells,” said lead author Benjamin Alexander Albert, a former undergraduate student researcher in the departments of biomedical engineering and computer science at Johns Hopkins University and a current PhD student at the University of California, San Diego.