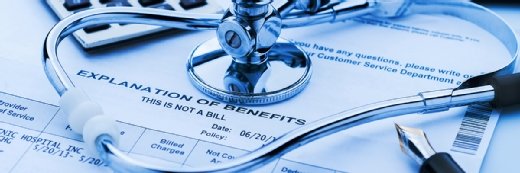
Christian Delbert - stock.adobe.
ML Model Outperforms Standard Methods for Detecting Heart Attacks
A UPMC-developed machine learning model used electrocardiogram readings to accurately reclassify 1 in 3 patients with chest pain based on heart attack risk.
Researchers at the University of Pittsburgh Medical Center (UPMC) have developed a machine learning (ML) tool capable of using electrocardiogram (ECG) readings to detect and classify heart attacks more quickly and accurately than standard approaches.
The model was created to address a common problem with chest pain triage: unclear ECGs.
“When a patient comes into the hospital with chest pain, the first question we ask is whether the patient is having a heart attack or not. It seems like that should be straightforward, but when it’s not clear from the ECG, it can take up to 24 hours to complete additional tests,” said lead author Salah Al-Zaiti, PhD, RN, associate professor in the Pitt School of Nursing and of emergency medicine and cardiology in the School of Medicine, in the press release. “Our model helps address this major challenge by improving risk assessment so that patients can get appropriate care without delay.”
The study assessing the model’s validity, which was published this week in Nature Medicine, indicates that distinct patterns in the peaks and valleys of ECG readings are crucial for clinicians to recognize a heart attack, but up to two-thirds of heart attacks do not have these patterns.
For patients with serious heart attacks caused by total blockages of a coronary artery, known as ST-elevation myocardial infarction (STEMI), failure to recognize clues in the ECG can lead to life-threatening complications.
The ML tool is designed to help detect subtle patterns in the ECG that may be clinically relevant but are easily missed by clinicians. The model also classifies patients based on whether they are at low, intermediate, or high risk of heart attack.
The model was developed using ECG data from 4,026 patients with chest pain at three hospitals in Pittsburgh, Pennsylvania. The tool was then validated using data from 3,287 patients at a separate health system.
To test the algorithm, the researchers compared its performance to three gold standards for evaluating cardiac events: experienced clinician interpretation of ECG, commercial ECG algorithms, and the History, Electrocardiogram, Age, Risk factors, Troponin (HEART) score.
The researchers indicated that they hoped to match the accuracy of the HEART score with their model, but they found that the ML significantly exceeded the performance of the gold standard approaches. The tool was able to accurately reclassify one in three chest pain patients as low, intermediate, or high risk.
These findings led the research team to conclude that the tool has the potential to help emergency medical services (EMS) and emergency department personnel flag people having a heart attack in a more robust way than with conventional ECG analysis.
“This information can help guide EMS medical decisions such as initiating certain treatments in the field or alerting hospitals that a high-risk patient is incoming,” explained study co-author Christian Martin-Gill, MD, MPH, chief of the EMS division at UPMC. “On the flip side, it’s also exciting that it can help identify low-risk patients who don’t need to go to a hospital with a specialized cardiac facility, which could improve prehospital triage.”
Moving forward, the researchers are partnering with the City of Pittsburgh Bureau of Emergency Medical Services to integrate the model into a cloud-based system that would allow hospital command centers to analyze and risk stratify patients based on ECG readings received from EMS, which could support medical decision-making in real-time.
Other AI systems are also being developed to predict heart attack risk.
Last year, researchers at Johns Hopkins University created Survival Study of Cardiac Arrhythmia Risk (SSCAR), an AI system designed to improve the accuracy of predicting cardiac arrest using raw images of patients’ hearts and demographic data.
Using this information, the system analyzes patterns not visible to the naked eye to detect important indicators of ten-year cardiac arrest risk.