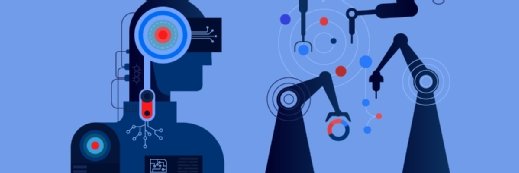
Getty Images
Deep Learning-Based Electrocardiogram Screening Detects Heart Defects
New deep learning model outperformed traditional methods for detecting signs of atrial septal defect, an underreported but potentially serious heart condition.
Researchers from Brigham and Women’s Hospital and Keio University in Japan have developed a deep learning (DL) model capable of screening electrocardiograms (ECGs) for signs of atrial septal defects (ASD), according to a study published last week in eClinicalMedicine.
ASD is a congenital heart defect caused by a hole in the wall dividing the upper chambers of the heart. During development, a fetus’ heart may have several of these openings, which typically close on their own during pregnancy or shortly after birth.
If one of these openings does not close, ASD occurs, which leads to an increase in blood flow through the lungs. This can cause damage to the lungs’ blood vessels over time, resulting in a higher risk of serious complications such as abnormal heartbeat, pulmonary hypertension, stroke, and heart failure in adulthood.
Since symptoms may not manifest until later in life, causing irreversible damage, early detection of ASD is important for improving patient outcomes. The researchers indicated that the condition can be corrected if caught early on, in which surgical closure of the hole may be performed to reduce future complications.
However, because the condition is largely asymptomatic until complications arise, the authors noted that ASD may be underreported. The Centers for Disease Control and Prevention (CDC) estimate that ASD may impact 13 of every 10,000 babies born in the United States each year, but improved detection may paint a clearer picture of the condition’s prevalence.
"If we can deploy our model on a population-level ECG screening, we would be able to pick up many more of these patients before they have irreversible damage," said Shinichi Goto, MD, PhD, corresponding author on the paper and instructor in the Division of Cardiovascular Medicine at Brigham and Women’s Hospital, in the press release detailing the study’s findings.
ASD is typically detected via listening to the heart with a stethoscope or performing an echocardiogram or ECG, but these methods have limitations. Listening to the heart can only find the largest defects, which the researchers stated represents approximately 30 percent of patients, necessitating the need for other, more sensitive screening methods.
Echocardiograms and ECGs help fill this gap, but echocardiograms are labor- and time-intensive, while ECGs are more efficient, but human interpretation of the imaging may miss abnormalities associated with ASD.
To address this, the research team set out to determine whether an artificial intelligence (AI) tool could improve ECG analysis to detect ASD.
They built a DL model using data from 80,947 patients over 18 who underwent both ECG and echocardiogram to detect ASD at three hospitals: US-based Brigham and Women’s Hospital, in addition to Japan-based Keio University Hospital and Dokkyo Medical University Saitama Medical Center. Approximately 857 patients in this cohort were diagnosed with ASD.
ECGs were randomly split into three datasets: 50 percent derivation, 20 percent validation, and 30 percent test, with no patient overlap to train and validate the model. The DL was trained using the derivation datasets from Brigham and Women’s Hospital and Keio University Hospital.
The model was then externally validated and tested using held-out data from Dokkyo, a community hospital with a more general population not specifically screening patients for ASD.
Doing so helped address a potential limitation in the study: reliance on samples from academic institutions, like Brigham and Women’s Hospital and Keio University Hospital, which deal more with rare diseases like ASD.
Overall, the DL was more efficient at detecting signatures of ASD than traditional methods, correctly detecting the condition 93.7 percent of the time. Using known abnormalities, the model found ASD 80.6 percent of the time.
"[The model] picked up much more than what an expert does using known abnormalities to identify cases of ASD," Goto explained. “The model's performance was retained even in the community hospital's general population, which suggests that the model generalizes well."
Other institutions are also leveraging AI and machine learning (ML) to improve cardiovascular care.
In a recent interview with HealthITAnalytics, Christian Martin-Gill, MD, chief of the emergency medical services (EMS) division at the University of Pittsburgh Medical Center (UPMC), discussed how the health system plans to utilize an ML model to classify cardiac events more effectively.
Risk-stratifying patients with chest pain can be challenging due to unclear ECGs, which can lead to delays in care and adversely impact patient outcomes.
ML may help combat this by enabling more efficient ECG analysis and providing a more comprehensive understanding of a patient’s heart health.