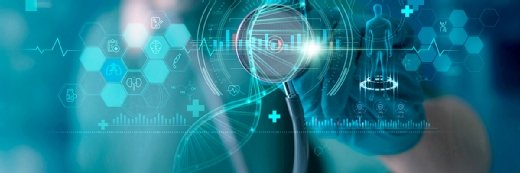
ipopba/istock via Getty Images
Mayo Clinic: Deep Learning May Improve Head, Neck Radiotherapy
A new Mayo Clinic study suggests that a deep learning tool could improve standardization and time efficiency for radiotherapy planning.
Researchers from Mayo Clinic demonstrated that an automated deep learning (DL) tool could help improve radiation therapy planning for patients with head and neck cancer, according to a validation study published in Frontiers in Oncology in April.
Radiotherapy planning for head and neck cancers is a complex, time-intensive process because it considers inputs and perspectives from multiple medical specialties. The presence of various organs and anatomical structures in close proximity to one another is another consideration clinicians must bear in mind, the study authors explained.
To address the time-consuming nature of radiation therapy planning, researchers set out to investigate whether a DL tool developed by Mayo Clinic and Google Health could help reduce the time needed for radiotherapy planning while improving plan quality and patient outcomes.
The research team began by asking two expert radiation oncologists to revise manually drawn head and neck contours. This contouring helps clinicians determine what healthy tissue surrounding a tumor may be at risk of radiation exposure.
These contours were then compared to those generated by the DL model, which was trained using 445 de-identified computed tomography (CT) scan contours from patients with head and neck cancer previously treated at Mayo Clinic.
Contours created by the DL model and by humans were then presented to eight blinded radiation oncologists for review and revision.
Overall, the DL tool was shown to produce head and neck contours that were appropriate for clinical use with minor to no revisions 90 percent of the time, versus 53 percent of the time for manually drawn contours. The model also reduced the time needed to produce contours by 76 percent.
"Head and neck radiation treatment planning is resource-intensive and cumbersome," said Samir Patel, MD, a radiation oncologist at Mayo Clinic and co-principal investigator of the study, in a press release discussing the findings. "This auto-contouring algorithm saved time in the cases we analyzed."
The algorithm may help extend the reach of Mayo Clinic’s radiation oncologists to the global radiation oncology community, Patel indicated.
This research is part of a larger strategic collaboration between Mayo Clinic and Google Health launched in 2019 to help improve care delivery for complex and serious medical conditions.
"This joint research demonstrates the power of Mayo Clinic and Google's collaboration, bringing together world-class radiation oncologists and medical physicists at Mayo Clinic with software engineers and researchers from Google to tackle an important issue for patients with cancer," said Cían Hughes, informatics lead at Google Health, in the press release. "This is early-stage work, and further clinical research will be required to see how this can be safely integrated into practice."
This research, the results of which were hinted at during The Check Up, Google’s annual health event, will continue outside of Mayo Clinic, according to the press release. Plans for future collaborations with other health systems and institutions to evaluate the algorithm’s efficiency in additional settings are currently underway.