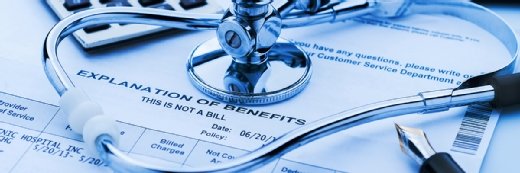
Christian Delbert - stock.adobe.
Cancer Symptom Algorithm Assists Doctors in Foreseeing ED Visits
A recent study described how using a cancer symptom algorithm aided clinicians in predicting which patients are at high risk for unplanned emergency department visits.
A study published in the Journal of the National Comprehensive Cancer Network (JNCCN) described how a cancer symptom algorithm provided insight into which patients may be at high risk for unplanned emergency department (ED) visits, which could provide additional benefits such as proactive care and lower costs.
As a nonprofit alliance of leading cancer centers, the National Comprehensive Cancer Network (NCCN) aims to improve care for patients while advancing research and education. Published in its journal, new research described how a novel tool could improve efficiency in the cancer care decision-making process while providing various potential other benefits, like reduced costs.
Using the Edmonton Symptom Assessment System-Revised (ESASr), researchers measured the most common symptoms that cancer patients exhibit. They classified subjective symptom complexity for each patient using the number and severity level of symptoms. They then correlated this information with the likelihood of having an ED visit within a week.
"Using a tool to generate a symptom complexity score—summarizing a large amount of symptom information and condensing it into a quick, easily interpreted alert—allows clinicians to have a real time, comprehensive overview of the symptom severity a patient is experiencing," said lead researcher Linda Watson, RN, PhD, of Cancer Care Alberta and the University of Calgary, in a press release.
With data from 29,164 unique cancer patients, researchers determined a correlation between symptom complexity and the chances of visiting the ED within a week.
After all study participants engaged in a questionnaire between Oct. 1, 2019, and April 1, 2020, 738 ended up in the ED within seven days. Researchers noted that those with high complexity, defined as exhibiting multiple co-occurring severe symptoms, were three times more likely to find themselves in the ED than those with low complexity, defined as exhibiting fewer or less severe symptoms.
Those with high complexity were also four times more likely than low complexity patients to be admitted to the hospital.
"With this information at their fingertips, clinicians can then proactively offer additional support or symptom management in the lower-cost out-patient care setting and hopefully avoid costly—and potentially distressing—hospital visits. Health care teams have to manage many competing priorities, so finding quick, reliable ways to identify which patients may benefit from targeted symptom management in lower-cost ambulatory care clinics would be helpful," said Watson.
The use of predictive tools to determine at-risk patients is growing more common.
For example, in June 2022, researchers described a machine-learning model that could accurately predict opioid overdose risk.
The development of the model consisted of gathering data from Medicaid beneficiaries in Pennsylvania. All subjects had one or more opioid prescriptions from 2013 to 2016. Researchers defined 284 potential predictors based on healthcare and pharmaceutical encounter claims from this data, all of which they measured in three-month periods beginning three months before the first opioid prescription.
This information was then used to predict the risk of hospital or ED visits for overdose in the subsequent three months.
Researchers tested and validated the model, finding that it successfully predicted opioid overdose in data from the same state in different years and a different state in other years.