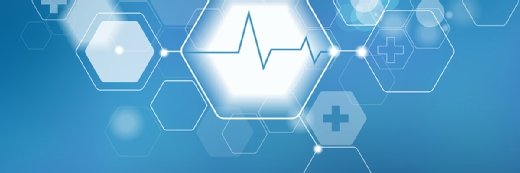
AlfaOlga/istock via Getty Images
Machine Learning Method Identifies Adverse Drug Side Effects
A machine learning tool can predict adverse drug side effects, offering a safer way to develop medicines.
An open-source machine learning tool identified proteins associated with adverse drug side effects, providing insight into how the human body responds to drug compounds at the molecular level, a study published in EBioMedicine revealed.
Researchers from Harvard Medical School and the Novartis Institutes for BioMedical Research developed the machine learning algorithm to offer a new way of creating safer medicines. The tool can detect potential adverse reactions before drug candidates reach human clinical trials or enter the market as approved medicines.
“Machine learning is not a silver bullet for drug discovery, but I do believe it can accelerate many different aspects in the difficult and long process of developing new medicines," said paper co-first author Robert Ietswaart, research fellow in genetics in the lab of Stirling Churchman in the Blavatnik Institute at HMS.
"Although it cannot predict all possible adverse effects, we hope that our work will help researchers spot potential trouble early on and develop safer drugs in the future.”
Adverse drug reactions can range from mild to fatal, the researchers noted. Effects can occur either when taking a drug as prescribed or as a result of incorrect dosages, interaction of multiple medicines, or off-label use. Adverse drug reactions are responsible for two million hospitalizations in the US each year, the team stated, and occur during ten to 20 percent of hospitalizations.
Over the years, researchers and providers have implemented many strategies to curb or reduce adverse drug effects in patients. However, because a single drug typically interacts with multiple proteins in the body, it can be hard to predict what effects a medicine may generate. If a drug does end up causing an adverse reaction, it can be difficult to identify which of its target proteins is responsible.
To develop the machine learning algorithm, researchers used one existing database of reported adverse drug reactions and another database of 184 proteins that specific drugs are often known to interact with. They then built the tool to connect the dots.
The algorithm learned from the data and uncovered 221 associations between individual proteins and specific adverse drug reactions, some known and some unknown. The associations indicated which proteins likely represent drug targets that contribute to particular side effects, and which others may be innocent bystanders.
The machine learning model could help scientists and doctors predict whether a new drug candidate is likely to cause a certain side effect on its own or when combined with particular medicines. The algorithm can help with these predictions before a drug is tested in humans, based on lab experiments that reveal which proteins the drug interacts with.
Researchers expect that this method could heighten the likelihood that a drug candidate will prove safe for patients before and after it reaches the market.
"This could reduce the risks that study participants face during the first in-human clinical trials and minimize risks for patients if a drug gains FDA approval and enters clinical use,” said Ietswaart.
To test the reliability of the algorithm, researchers compared its results to drug labels, conducted text mining of scientific literature, and leveraged other validation techniques. Although the team worked to strengthen the model as much as possible, it still evaluates less than one percent of the 20,000 genes in the human genome.
This shows how far the technology still has to go, the group noted.
"Our work is by no means a complete understanding of adverse drug events because many other genes and proteins might contribute for which no assay is available or no drugs have been tested," said Ietswaart.
The machine learning model is freely available online for scientists to use, improve, and refine.
"This work has been a collaborative ‘open science’ spirit and team effort," said Ietswaart.