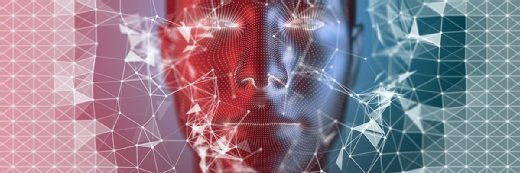
Getty Images
UPMC, Pitt Develop Machine Learning Model to Predict Brain Injury Outcomes
Scientists from UPMC and Pitt developed a prognostic model that can accurately predict survival and recovery outcomes six months after a traumatic brain injury.
According to researchers from UPMC and the University of Pittsburgh School of Medicine, advanced machine learning can be used to predict outcomes in patients with severe traumatic brain injuries (TBI).
In a study published in Radiology, the researchers created a deep learning model that analyzes admission CT scans and clinical data from TBI patients to predict six-month mortality and other unfavorable outcomes. The researchers noted that it typically takes two weeks for patients with severe TBI to emerge from a coma, but these patients are often taken off life support within 72 hours after hospital admission.
“Every day, in hospitals across the United States, care is withdrawn from patients who would have otherwise returned to independent living,” said co-senior author David Okonkwo, MD, PhD, professor of neurological surgery at Pitt and UPMC, in the press release. “The majority of people who survive a critical period in an acute care setting make a meaningful recovery—which further underscores the need to identify patients who are more likely to recover.”
The Centers for Disease Control and Prevention have asserted that TBI is a pressing public health issue. Over 64,000 TBI-related deaths were reported in 2020, and TBIs are most commonly caused by falls, firearm-related injuries, motor vehicle crashes, and assaults. As an injury that affects how the brain works, TBIs are a major cause of death, disability, and serious short- and long-term health problems.
“There is a great need for better quantitative tools to help intensive care neurologists and neurosurgeons make more informed decisions for patients in critical condition,” said corresponding author Shandong Wu, PhD, associate professor of radiology, bioengineering and biomedical informatics at Pitt, in the press release. “This collaboration with Dr. Okonkwo’s team gave us an opportunity to use our expertise in machine learning and medical imaging to develop models that use both brain imaging and other clinically available data to address an unmet need.”
In order to meet this need, the research team began work on their deep learning algorithm. The model was trained and validated using two patient cohorts: one of 537 patients treated for severe TBI at UPMC and another of 220 patients taken from the Transforming Research and Clinical Knowledge in Traumatic Brain Injury (TRACK-TBI) consortium.
The model utilizes multiple patient brain scans, combined with estimates of coma severity and the patient’s vital sign, blood test, and heart function information, to form its predictions. The model was also trained on different image-taking protocols, indicating that the model can account for data irregularities present in different types of brain imaging and image quality.
The model’s performance was evaluated against the International Mission on Prognosis and Analysis of Clinical Trials in TBI (IMPACT) model and the predictions of three neurosurgeons. The model accurately predicted patient mortality risk and unfavorable outcomes at 6 months following the traumatic incident, and it outperformed the predictions of the neurosurgeons.
The research team hopes that their work highlights how AI can provide a valuable tool for improving early clinical decision-making in TBI care and yielding enhanced patient outcomes.