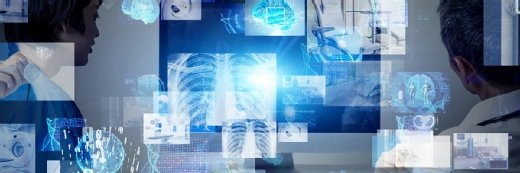
metamorworks/istock via Getty Im
Population Health Data Modeling Evaluates COVID-19 Response
Researchers studied population health data modeling to look at how COVID-19 restricts affected infection and GDP.
Researchers used population health data modeling to determine how COVID-19 restricts impact infections and gross domestic product (GDP).
This study was conducted in Australia and uses Victoria, Australia, as a sample. However, due to the universal nature of the COVID-19 spread, it could provide key insights to public health officials around the globe.
Strategies for handling the COVID-19 pandemic have varied greatly by country. While some countries such as Australia, New Zealand, and Taiwan pursued full elimination strategies, other countries including Brazil and India took a virtually unmitigated approach.
“There is no best approach for all countries to follow when handling the COVID-19 pandemic. Rather, each country will devise an approach given updated scientific understanding, its infection load, its ability to manage borders and quarantine, vulnerability and age structure of its population, health system capacity, economic and other resources, social values and preferences, and, more recently, timelines and progress to vaccination,” the authors wrote.
The United States found itself somewhere in the middle of those approaches with a loose suppression of COVID-19 restrictions. To determine the best policy response through population health data, researchers used a net monetary benefit approach to study policy implementation ranging from aggressive elimination and moderate elimination to tight suppression and loose suppression.
With the use of government population health data from the state of Victoria, Australia, two different simulation models were conducted of all residents for COVID-19 infection for one year. An agent-based model (ABM) was used in the study to estimate the daily COVID-19 infection rates and time in five stages of social restrictions for the four different policy responses.
Additionally, a proportional multistate life table (PMSLT) was created to estimate the health-adjusted life-years (HALYs) associated with COVID-19 and costs for the health systems and GDP. The ABM represented a genetic COVID-19 model of 2500 agents, or stimulants, and was scaled up to the population of interest.
“Models were specified with data from 2019 (eg, epidemiological data in the PMSLT model) and 2020 (eg, epidemiological and cost consequences of COVID-19). The NMB of each policy option at varying willingness to pay (WTP) per HALY was calculated: NMB = HALYs × WTP − cost. The estimated most cost-effective (optimal) policy response was that with the highest NMB,” the study stated.
In 100 runs of both the ABM and PMSLT model for each other the four policy responses, 31 percent of the COVID-19 infections, 56.5 percent of hospitalizations, and 84.6 percent of death occurred in those 60 years and older.
While aggressive elimination resulted in the highest percentage of days with the lowest level of restrictions, days in hard lockdown were similar across all four strategies. The HALYs showed similar results for aggressive elimination and moderate elimination. However, there were nearly 8- and 40-times higher results for tight suppression and loose suppression.
The median amount of GDP lost was lowest for moderate elimination, but there was a significant overlap in simulations intervals between the four strategies.
“From a health system perspective, aggressive elimination was optimal in 64% of simulations above a WTP of $15 000 per HALY, followed by moderate elimination in 35% of simulations. Moderate elimination was optimal from a GDP perspective in half of the simulations, followed by aggressive elimination in a quarter,” the study stated.
The study concluded that “In this simulation modeling economic evaluation of estimated SARS-CoV-infection rates, time under 5 stages of restrictions, HALYs, health expenditure, and GDP losses in Victoria, Australia, an elimination strategy was associated with the least health losses and usually the fewest GDP losses.”