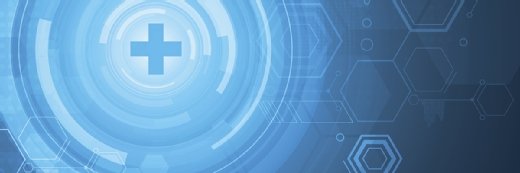
traffic_analyzer/DigitalVision V
AI Predict Mortality Risk Using Socioeconomic, Clinical Data
Researchers created an artificial intelligence tool using socioeconomic and clinical data to predict mortality risks in patients with cancer.
By using socioeconomic and clinical data, researchers developed an artificial intelligence tool to predict 30-day mortality risks in patients with cancer.
Cancer is one of the leading causes of death in the United States each year. Using the artificial intelligence tool, medical professionals can identify patients who are at high risk and provide early intervention and resolutions for reversible complications.
Additionally, the tool can determine those patients approaching end of life (EoL) and refer them to early palliative and hospice care. Doing so will improve the patient’s quality of life and symptom management, according to the study.
“In contrast, aggressive, life-sustaining EoL care can conflict with patient preference and result in lower quality of life, family perceptions of poorer quality of care, and greater regret about treatment decisions. Earlier referral also represents an opportunity to transform cancer care by reducing the potential for unnecessary, toxic and expensive treatments at EoL,” the study authors wrote.
Physicians will often delay advance care planning and EoL conversations until a patient is in the terminal phase of life. Current methods and models of predicting mortality vary in accuracy, only include clinical factors, and do not provide additional information to clinicians.
However, authors observe that including sociodemographic and geographic factors in AI predictive analytic models can identify patients at greater risk of short-term mortality, providing better management and care for patients with cancer.
Researchers conducted the study by selecting 3671 patients from a de-identified database representing a large community-based hematology/oncology practice. Data from the de-identified database included electronic health records (EHRs), billing data, and socioeconomic determinants of care.
The data collected from patients included demographic data (e.g., gender, race, age) and cancer information (e.g., type, stage). Additionally, researchers looked at socioeconomic data, including lifestyle choices and the individual’s living environment. The socioeconomic data was highly weighted in the analysis.
The machine learning algorithm was able to accurately predict the 30-day mortality among patients with cancer. The study also examined the algorithm’s ability to predict 60-, 90-, and 180-day mortality, which reflected similar results.
Researchers concluded that the machine learning algorithm’s ability to identify patients with cancer at risk for 30-day mortality has the potential to improve outcomes for patients with reversible clinical factors. Additionally, the AI system can prevent unnecessary and harmful care for those nearing EoL.
“AI applications will utilize clinical, sociodemographic, environmental, pathologic, radiological, genetic, genomic and pharmacoepidemiological data to recommend, the treatment with greatest efficacy and least risk of toxicity, for a patient with cancer as well as offer direction for best available clinical trial, stratify risk of recurrence and implement prognostic models for a given patient as well as identify risk of disease within family members,” the authors wrote.