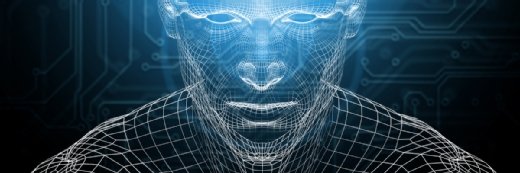
Getty Images
Machine Learning, EHR Data Reveal Chronic Disease Associations
A machine learning tool mined EHR data to uncover novel chronic disease associations, which could help identify new research paths.
A machine learning algorithm can mine EHR data and discover novel associations between common chronic diseases and lesser-known conditions, which could support earlier monitoring or medical intervention, according to a study published in PLOS One.
The use of EHRs in large health systems offers the opportunity to conduct population-level analyses that explore disease progression. The team wanted to identify novel comorbidities from routinely collected, anonymized EHRs.
Researchers developed a machine learning algorithm, called the Phenome-Disease Association Study (PheDAS), to perform association studies and identify comorbidities across time in EHRs. The team validated the tool using three example conditions: Alzheimer’s disease, autism spectrum disorder, and optic neuritis, which can be the first indication of multiple sclerosis.
The group used de-identified EHRs of patient groups with each of the three conditions and appropriate control groups with comparable demographics but without disease diagnosis. Researchers mined real-time journal article abstracts because well-known disease associations will likely have papers published on them than novel associations.
The algorithm searched for and tallied mentions of associations to each condition from article headlines, abstracts, and keywords. The team found 194,736 articles with search terms related to Alzheimer’s, 45,419 with terms related to autism, and 18,894 with optic neuritis terms. Roughly 15,000 codes from the International Classification of Diseases (ICD) system were mapped to 1,865 PheCodes, which are combinations of ICD codes for distinct diseases, traits, or conditions.
The researchers ranked associations by comparing each PheCode-disease finding to the number of papers found that mention both the diagnosis and the associated condition as a proportion of the number of papers published on the disease of interest. After adjusting to well-known associations and those with less likely clinical relevance, the novelty score moves the paper proportion from an absolute scale onto a relative scale.
The results showed that PheDAS correctly identified well-known associations with each of the three target conditions. The algorithm also found lesser-known conditions that may support earlier monitoring or medical interventions and could suggest new research opportunities.
“We are excited about the opportunities to discover new risk factors and associations of diseases in the clinical record,” said Bennett Landman, associate professor of electrical engineering, computer engineering and computer science. “Overall, our goal is to advance engineering and clinical science to improve the understanding and care of patients.”
The researchers also noted that some associations will be so random that they are unlikely to be related or have extremely limited relevance. A new “Novel Finding Index” will help guide researchers to significant associations that may be clinically relevant but haven’t been thoroughly studied in medical literature. The index gives well-known disease associations a low ranking.
For example, in the case of Alzheimer’s disease, well-known associations included psychosis, cerebral degenerations, and gait abnormalities, which were given a low novelty score. The algorithm identified infections and inflammatory processes across several organ systems as novel associations, so these associations received higher scores.
The three example conditions validated the tool, which researchers can now use to evaluate other diseases if they have approved datasets from the Synthetic Derivative, a database containing anonymized clinical information derived from Vanderbilt’s medical record of 2.2 million people. Data from medical imaging is now also added to the Synthetic Derivative, creating easier, user-friendly access to large amounts of data.
“Our lab primarily focuses on medical imaging, including magnetic resonance imaging and computed tomography,” Landman said. “These new tools will allow us to better interpret imaging findings in the context of a patient’s broader story.”
The results have promising implications for the study of common and rare disease associations.
“Our results demonstrate wide utility for identifying new associations in EMR data that have the highest priority among the complex web of correlations and causalities,” the team concluded. “Data scientists and clinicians can work together more effectively to discover novel associations that are both empirically reliable and clinically understudied.”