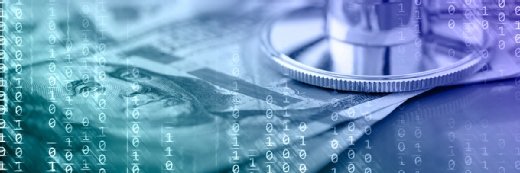
Viorika/istock via Getty Images
Will Clinical Decision Support, Health IT Cut Diagnostic Errors?
Diagnostic errors are common across the care continuum, but clinical decision support tools - if implemented correctly - could help reduce patient safety events.
Healthcare professionals are continually being asked to do more with less. With more patients but less time; more data but fewer meaningful tools to help them process it, it seems almost inevitable that diagnostic errors and patient safety shortfalls will creep into a system under stress.
While some of these errors can be fixed quickly with a phone call apologizing for misreading a test result, others are may result in serious liability concerns, including unnecessary treatments, emotional anguish for patients, missed opportunities for care, and even mortality.
Diagnostic errors happen across the care continuum and as patients move through multiple points of potential failure, says a new report by Coverys, and many of them are severe in nature.
After analyzing more than 10,000 closed medical liability claims, the company found that a third of all claims – and 47 percent of indemnity payments – are related to diagnostic errors.
Nearly a quarter (24 percent) of diagnostic errors take place in the emergency department or urgent care, while 36 percent of claims originate in the outpatient setting.
More than half (54 percent) are classified as “high severity.” Thirty-six percent resulted in death.
“Diagnostic accuracy is far from simple, and diagnosis-related events have come to represent the single largest root cause of medical professional liability claims, leading to serious and sometimes deadly results,” said Robert Hanscom, Vice President, Business Analytics for Coverys and co-author of the report.
A third of diagnostic error claims allege that mistakes during patient evaluation led to an adverse outcome, while more than 50 percent of claims are rooted in misinterpretation of test results or failure to order, perform, or receive data on testing.
Breakdowns in the diagnostic process have become a top concern for patient safety advocates, especially in light of the concurrent rise in health IT use to supplement the clinical decision-making process.
The ECRI Institute, which monitors the industry’s top patient safety trends in an annual report, designated diagnostic errors as its number one concern for 2018.
“It’s a multifactorial problem,” says Gail M. Horvath, MSN, RN, CNOR, CRCST, patient safety analyst and consultant, ECRI Institute. “Diagnostic errors are the result of cognitive, systemic, or a combination of cognitive and systemic factors.”
They are also exceedingly difficult to track and pinpoint, ECRI added. Just like with other adverse patient safety events, providers may be reluctant to admit that they may have made a mistake, even if their employers purport to have non-punitive policies in place.
In addition, diagnostic errors often do not show themselves immediately. With nearly 30 percent of liability claims related to cancer and 8 percent involving cardiovascular conditions, according to Coverys, longer-term illnesses may obscure the original problem.
“To help improve diagnostic accuracy, improve patient safety, and reduce claim frequency, physicians and healthcare providers need data-driven insights, fresh perspectives, and new ways of thinking about the everyday activities that contribute to making a patient diagnosis,” said Hanscom.
For many healthcare organizations, “new ways of thinking” about diagnostics will involve health IT tools such as clinical decision support (CDS) software, risk scoring strategies, and EHR workflows that include checklists based off recognized clinical guidelines.
CDS tools are becoming increasingly popular as a way to synthesize large volumes of information and ensure that key details do not escape the attention of busy clinical staff.
Globally, healthcare organizations will spend around $1.3 billion on clinical decision support tools by 2025, says a new report by Transparency Market Research, with diagnostic decision-making and prescription drug support among the most lucrative segments.
The rapid rise of machine learning and artificial intelligence techniques has produced especially significant excitement over the potential of CDS tools to revolutionize diagnostics: from reading radiology images to recognizing patterns in pathology slides, these methodologies are full of promise for augmenting the skills of human clinicians.
Yet adoption of advanced AI imaging analytics is still in its infancy, and healthcare organizations are approaching this new category of tools with caution.
A recent KLAS Research brief found that only 17 percent of large integrated delivery networks and hospital systems are currently piloting AI-driven imaging analytics tools, and 53 percent have no concrete plans to dive into the world imaging analytics at the moment.
Workflow concerns, unclear use cases, the expense of implementing new systems, and uncertainty about whether or not clinicians would accept these new tools are slowing down adoption, the report noted.
That could be because many organizations have been burned by lackluster CDS tools before. The first generation of CDS options has garnered a reputation for being less than a joy to work with, especially when integrated into electronic health record workflows.
Constant alerts, alarms, notifications, and pop-ups can down workflows and aggravate providers to a shocking degree – in 2014, physicians within the VA healthcare system said that handling dozens of low-value EHR alerts every day was likely to drive them to quit their positions all together.
Alert fatigue has remained a top challenge since then. Alarm fatigue was a constant theme throughout ECRI’s 2017 patient safety list – the same report that tapped diagnostic errors as the number one concern this year.
Providers desperate to avoid alerts that don’t help them make better decisions may turn to workarounds, the number four hazard on ECRI Institute’s 2018 patient safety list, contributing to the challenges of the next item in the report: the overall dangers of poorly integrating health IT into tasks related to keeping patients safe.
“It is not only how we use [health IT] in daily workflow, but also how we use it effectively by optimizing the benefits and reducing the risks,” says Robert C. Giannini, NHA, CHTS-IM/CP, patient safety analyst and consultant, ECRI Institute.
Turning clinical decision support tools into a help instead of a hindrance for diagnostics and other aspects of patient care requires developers and providers to create smarter alerts supported by better analytics – including machine learning – to create intuitive and logical workflows.
The first step involves conducting a thorough assessment of the organization’s cultural readiness to embrace quality CDS tools, says CMS.
“CDS implementation and adoption cannot begin until it is clear that your organization is ready to use CDS for your selected clinical goals and priorities,” the agency says in a resource guide.
“Assessing this readiness is critical. To understand how well your organization can handle change (what type of change, how much, and over what time period) is a key aspect of readiness. The concept of ‘adaptive reserve’ – what resources are available to plan for, adapt to, and reflect on major change in an organization – is helpful to consider.”
Organizations should also take a close look at the quality and integrity of their clinical documentation and other data assets to determine if existing datasets are sufficient to support new algorithms designed to extract actionable insights from clinical, financial, and operational information.
Clinical decision support cannot be effective if providers or organizational leaders do not trust the accuracy and reliability of the underlying data, or if they feel that not enough data is being used to generate suggestions for care.
Identifying a well-defined use case for a new CDS effort will help organizations understand what data they need and how much of it is required for success.
While an inpatient sepsis detection tool might require real-time data from bedside monitoring devices and speedy access to lab test results, a tool to support diagnosing rare cancers may instead rely on ingesting and processing huge volumes of historical medical literature from journals and case studies.
Choosing the right CDS vendor with the right set of skills to address specific problems will help to avoid waste while ensuring that providers do not receive alerts or alarms that are irrelevant to the situation at hand.
Provider organizations should also be careful to measure the effectiveness and overall impact of any new tools. Before implementation, leaders should take baseline measurements about productivity, alert overrides, and the number of patient safety or diagnostic error reports.
Measurements should be repeated at regular intervals during and after implementation to gauge improvements or trouble spots, and clinicians should be consulted throughout the process to tweak workflows or make adjustments.
Listening closely to provider concerns about the functionality of CDS tools may help organizations implement software systems that can drive down the rate of diagnostic errors instead of potentially contributing to them.
Creating a culture that truly prioritizes self-reporting and collaborative, non-judgmental discussion around errors and missed opportunities is also essential for reducing potentially hazardous situations.
As choosier patients demand more accountability and higher quality from their healthcare providers, understanding the origins and propagation of diagnostic errors across the care continuum will become a crucial competitive differentiator for health systems and individual clinicians.
More effective health IT use may also help to drive down high indemnity payments and avoid legal action from patients. Fewer legal entanglements can only aid a health system’s brand and community reputation.
The addition of artificial intelligence and machine learning to the CDS market segment may be the key to realizing these improvements to care quality and financial health.
As AI and machine learning become increasingly sophisticated, moving from pilot programs to system-wide implementation, providers have an opportunity to create effective health IT tools that support clinicians in their work.
Doing so will require careful planning and comprehensive assessments of data assets, organizational readiness, a collaborative attitude, and viable use cases for an important category of emerging patient safety and care quality tools.