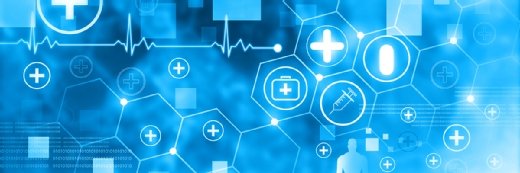
Rasi Bhadramani/istock via Getty
AI model boosts delirium detection rate in hospitals
Research shows that using a new AI model resulted in a four-fold increase in the rate of delirium detection among hospitalized patients, enhancing delirium treatment.
A multimodal AI model increased the rate of delirium detection in hospitalized patients, improving treatment of the condition, according to a new study published in JAMA Network Open.
Delirium is a state of severe confusion stemming from various medical, surgical, pharmacological and environmental factors. It occurs frequently among hospitalized individuals. Though it can increase the risk of morbidity and mortality, delirium diagnoses are often missed and delayed, the study authors noted.
Thus, the researchers from the Icahn School of Medicine at Mount Sinai and Mount Sinai Health System set out to assess whether a multimodal machine learning model can accurately stratify the risk of hospital delirium. Machine learning, a type of AI, 'learns' from data and can improve its performance over time.
In the study, the researchers described the development and testing of a multimodal ML model developed at Mount Sinai. The model leverages EMR patient data features and clinical note features processed using natural language processing to stratify the risk of non-intensive care unit (ICU) delirium. The model uses the Confusion Assessment Method (CAM) as the diagnostic reference standard.
The researchers used data from non-ICU patients aged 60 or older at Mount Sinai Hospital between January 2016 and January 2020 to train and test the ML model. The model was validated in live clinical practice from March 2023 to March 2024.
The overall sample included 32,284 inpatient admissions. During live clinical deployment, the model achieved an Area Under the Receiver Operating Characteristic curve of 0.94, a sensitivity of 83% and a specificity of 90%.
The researchers found that monthly delirium detection rates significantly increased during the deployment period, with median rates rising from 4.42% before the ML was implemented to 17.17% after. This represents a four-fold increase in delirium detection rates.
Researchers also found that patients in the post-ML cohort received significantly lower daily doses of benzodiazepine, an anti-anxiety medication, and olanzapine, an anti-psychotic drug.
Researchers concluded that the model demonstrated acceptable performance in live clinical practice and could help enhance clinical decision-making and resource allocation.
"Our model isn’t about replacing doctors -- it's about giving them a powerful tool to streamline their work," said the study's senior corresponding study author, Joseph Friedman, MD, founder and director of delirium services for the Mount Sinai Health System, in a press release. "By doing the heavy lifting of analyzing vast amounts of patient data, our machine learning approach allows health care providers to focus their expertise on diagnosing and treating patients more effectively and with greater precision."
This is the latest example of a machine learning tool that supports delirium prediction.
In 2023, Johns Hopkins University researchers developed machine learning algorithms that could detect the early warning signs of delirium and predict which patients will be at high risk of delirium during their ICU stay.
The researchers applied AI algorithms to a publicly available dataset containing data on 200,000 ICU stays at 208 hospitals across the U.S. Using this data, they developed two ML models, one static and one dynamic, for predicting delirium risk.
They tested each model on two other data sets from a Boston hospital and found that the static model could accurately predict which patients would experience delirium 78.5% of the time, while the dynamic model could predict delirium-prone patients up to 90% of the time.
Anuja Vaidya has covered the healthcare industry since 2012. She currently covers the virtual healthcare landscape, including telehealth, remote patient monitoring and digital therapeutics.