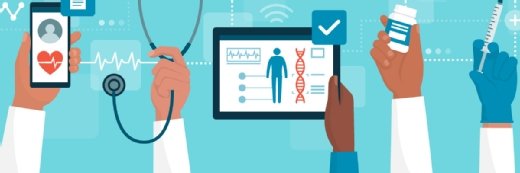
elenabs/istock via getty images
Machine Learning Improves Patient Collections at Allina Health
Allina Health saw a $2 million patient collections boost after using machine learning to develop a propensity-to-pay model and personalized collection strategies.
Patient collections is a significant undertaking at Allina Health, a 12-hospital system with over 90 clinics, 52 rehabilitation facilities, and dozens of other care sites across Minnesota and western Wisconsin. The not-for-profit health system attempts to collect outstanding balances for approximately 20,000 patients every month.
Financial representatives are sensitive to the fact that patients are struggling to afford their healthcare. They have seen deductibles go from an average of $303 in 2006 to over $1,200 a decade later. And on top of growing deductibles, many health plans also require patients to make copayments or coinsurance payments when receiving certain healthcare services.
“At Allina, we've done our best to try and come up with a program that can meet patients where they are with their personal financial situation,” Barbara Ball, director of accounts receivable services at Allina Health, recently told RevCycleIntelligence.com. “But in order to do that we have to have a conversation with them.”
Engaging in a dialogue around patient collections and financial responsibility gives the health system the opportunity to create a personalized patient financial experience in which staff offer interest-free loan options, connect patients with finance assistance programs, or even discuss the burden of healthcare costs.
Those conversations also enable health systems to address patient financial responsibility, which accounts for 88 percent more of hospital revenue compared to seven years ago.
However, the sheer size of Allina Health was preventing financial representatives from having the conversations they wanted to with all their patients.
“Allina is a huge, complex organization. We have anywhere between twenty and forty thousand accounts that qualify to be called every day,” Ball stated. “We would need an army of people to be able to do that and obviously we don't have an army of people. We just weren’t getting through all the accounts.”
Ball and her team acknowledged that they needed a way to identify patients who financial representatives should call and when. And to achieve that, the health system needed to enlist the help of a propensity-to-pay model.
Propensity-to-pay models use data points like previous bad debt and how often a patient has paid in the past to score accounts based on a patient’s likelihood to pay a medical bill. The scores help providers determine who is likely to pay a bill after placing a call, as well as those who are likely to pay without any intervention.
Artificial intelligence and machine learning have elevated propensity-to-pay models, allowing providers to predict a patient’s likelihood of paying a medical bill before the account lands in the bad debt pile.
Allina Health knew their financial representatives would benefit from a propensity-to-pay predictive model that uses innovative machine learning. The scores would help them quickly and accurately process patient collections data for tens of thousands of patients while also helping them to personalize conversations to ensure patient collection success.
But the health system could not implement an out-of-the-box model. Ball and her team needed a model that could not only analyze the large volumes of data coming from dozens of hospitals and clinics, but also understand the system’s guarantor method of medical billing.
“If you do guarantor billing, you have a household statement that might have a spouse and three children, as well as the financially responsible party, or guarantor. So, we’re not necessarily sending separate statements for everybody in a household,” Ball said.
“Interpreting data and trying to come up with a propensity-to-pay for the household when you've got maybe thirty visits from five people is difficult. It’s challenging to handle the various relationships within the data that need to roll up to the algorithm.”
Ball decided to develop a propensity-to-pay predictive model with the health IT company Health Catalyst, which already had a relationship with Allina Health and experience with an Epic EHR system like the one used by the health system.
“Health Catalyst ran the data through their proprietary algorithm and gave me the data to perform validation,” Ball elaborated. “There's machine learning that happens along the way, but there's also some common sense that needs to take place because we wanted different weights placed on different types of data within the model.”
Ball and her team wanted to customize propensity-to-pay categories by expanding them beyond the traditional high, medium, low propensity-to-pay scores.
“We wanted to factor in the various pieces of information that were available to us and that were maybe unique to Allina,” she explained. “For example, Allina has a charity care program and a patient in the program may also have been on medical assistance within the past two or three years. So, that makes him more likely to qualify for medical assistance again in the future and/or likely to qualify for our charity program going forward. We would want to apply a different strategy to that patient account.”
“Additionally, there might be a fairly new patient,” she added. “There just isn't a lot of data out there to analyze in that case. So, you wouldn't want to necessarily rank that person as having a low propensity-to-pay just because they don't have data.”
Ball developed a propensity-to-pay predictive model that assigns a wide range of scores, from high and medium-high to low and extreme low. She even came up with a low propensity-to-pay score based on previous history of government assistance or charity care.
“We want to segment the populations to develop a strategy using the tools that we had available to us, as well as the programs that we have available to us,” she said.
Using the granular scores, financial representatives are now calling patients with a patient collection strategy already in place. The scores help indicate whether a patient just needs a gentle reminder that they owe their provider or if they would benefit from a payment plan through the health system.
“We go into the conversation with a strategy in the back of our minds, so it is less of a cold call,” Ball stressed. “We ask more prompting questions and listen for those cues.”
Ball and her team also developed a strategy to streamline the patient collections process and get to more accounts each day.
“If a patient has a high propensity-to-pay score, then the data shows us that 95 percent of the time, they are going pay us without being bothered. In those cases, we wouldn't even bother calling until it was close to the point where it would be going to a final notice,” she said.
Using machine learning to assign detailed propensity-to-pay scores helped Allina collect $2 million more in the first nine months of 2018 compared to all of 2017, Ball reported.
The health system also saw a 37.5 percent relative improvement in the number of outbound calls, as well as a 21 percent relative improvement in the number of inbound calls, meaning Allina Health is finally having the financial conversations they always wanted to have with patients.