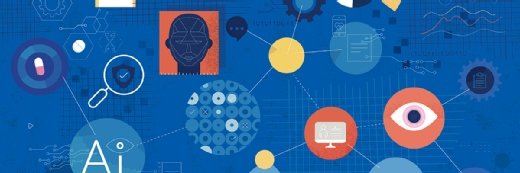
DrAfter123/DigitalVision Vectors
Should Population-Based Payments Account for Social Risk Factors?
A new study shows that adding social risk factors to risk adjustment models for population-based payments may entrench, not improve, health disparities.
Efforts to make the healthcare system more efficient and equitable by adjusting population-based payments for social risk factors may be missing the mark, suggests a new study from Harvard and Yale. The study published in the latest edition of Health Affairs puts current risk adjustment methodologies under a microscope to find out if they truly support equitable delivery of care.
Researchers tied individual-level predictors of social disadvantage (i.e., race, ethnicity, and educational attainment) to the Hierarchical Condition Categories (HCC) model currently used to risk-adjust payments in Medicare Advantage and benchmarks in the Medicare Shared Savings Program. They found that Medicare spending was similar or substantially lower for groups at higher risk of experiencing social disadvantage. For example, total annual Medicare spending per beneficiary was $574 lower for Black beneficiaries and $1,462 lower for Hispanic beneficiaries than for White beneficiaries.
The findings run counter to what some may think: groups at higher risk of experiencing social disadvantage should have higher spending compared to groups with less risk. Healthcare stakeholders have expressed concerns that risk adjustment methods will fail to account for the higher spending associated with historically marginalized populations, resulting in worse health disparities.
However, Harvard and Yale researchers say that “adding social factors, particularly race and ethnicity, to the HCC model can entrench health disparities instead of reducing them, by lowering population-based payments to more accurately predicted levels of spending.”
Historical and current levels of spending, which are typically at the core of risk adjustment methods, are not the desired levels of spending for high-risk groups, researchers explain. Therefore, prediction models that include social risk factors in their calculations may accurately predict spending but also reduce payments for underserved populations compared to models that omit the factors.
Predictive accuracy may not be the best goal for risk adjustment, the study indicates. Instead, researchers suggest “a population-based payment system that set payments above current levels of fee-for-service spending for groups with greater deficits in health care access or quality (and set payments below current spending for others) would create incentives for providers or plans to attract those groups and help address resource disparities that contribute to health care disparities.”
Risk adjustment, they say, will need to add more individual-level and area-level payment adjustments to deliver appropriate population-based payments to give providers the resources to effectively treat and manage high-risk populations based on social risk factors. For accountable care organizations (ACOs), this means a move away from using historical spending to determine spending benchmarks.
ACOs and others involved in value-based care have been calling for ACO benchmarking reform, specifically asking CMS to retire historical spending as the basis for benchmarks.
Approaches “that are based on historical spending with periodic rebasing create a disincentive for APM participants to fully maximize potential savings, and ultimately can drive providers to drop models,” Jeff Micklos, executive director of the Health Care Transformation Task Force, said in a 2021 CMS call with stakeholders. Instead, “CMMI should focus efforts on developing benchmarking methodologies that support appropriate spending levels on care, limit rebasing and do not penalize model participants for the savings they achieve for their assigned populations."
Aisha Pittman, vice president of policy with Premier at the time, also said the current benchmarking approach “really prevents certain types of providers and patient populations from being included in the models. Providers who are paid at cost, like critical access hospitals, cannot achieve savings on top of their historical spend.”
Researchers suggest one approach that uses “constrained regression to increase the payment weights on HCCs that are more prevalent among beneficiaries with” certain social risk factors, such as less education. Therefore, payment would exceed current spending on that beneficiary by the desired amount. Stakeholders could also “implement post-estimation adjustments (after estimation of the risk-adjustment model) to redistribute payment toward the group of interest.”
However, community-level adjustments are also needed to advance health equity despite being considered poorly targets, researchers add.
“[T[hey may nevertheless be important complements to individual-level adjustments,” they write. “The latter are critical to establish incentives for MA plans or ACOs to compete for underserved patients. For plans or ACOs to act effectively on those incentives, however, they must include providers serving those patients’ communities. Payment reallocations at the area level may have greater influence on market entry and network inclusion decisions made by plans and ACOs.”