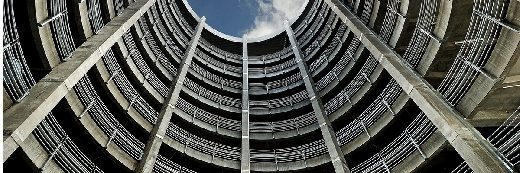
keller - Fotolia
How DataOps architecture benefits your analytics strategy
A DataOps framework can provide access more efficiently throughout an organization to ease the analytics process and deliver insights faster.
According to a recent Forrester Research blog, only 20% of raw business and operational data makes it into analytical databases and applications. That may contribute to another Forrester statistic that only 7% of surveyed companies reported advanced insights-driven practices.
The success of any analytics strategy hinges on the ability to access relevant data. Realizing that, more companies are adopting a DataOps architecture.
What is DataOps?
DataOps supports a distributed data architecture that maintains a range of open source tools and frameworks. The architecture is meant to break down data silos across operations and improve communications between stakeholders and data professionals within a business.
Like DevOps, which has become popular in the software development space, a key goal of DataOps is to make an organization more Agile. It involves getting usable data into the hands of data scientists, analysts and citizen data scientists faster and more efficiently.
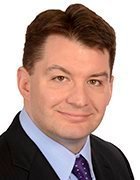
Providing data on demand doesn't just happen. The data must be in a usable state and have all the appropriate guardrails around it, including governance, compliance and security.
"The point of DataOps is to create more predictable delivery and change management in data, data models and other artifacts using technology to help automate and orchestrate," said Nick Heudecker, vice president analyst at Gartner. "But it's also a people discipline, and that might mean introducing new roles and rearchitecting organizations as far as how they might collaborate and communicate around data."
How a DataOps architecture benefits analytics
Data quality and availability directly impact the quality of data analysis. However, the data also must be governed, compliant and secure. DataOps combines the rigor of sound data engineering and data management with fast, role-based data access.
Rajesh Gill, associate director of commercial insights at Amgen, a biopharmaceutical company, said half of his work each week is unplanned because people in the organization have a lot of ad hoc questions. For example, an executive might ask about the market share impacts of patients trying a drug for the first time, trying the drug for the second time or trying the drug after using another drug.
"Those are three different questions that typically derail our analytics work because we start planning things, we set a goal and we move towards it," Gill said. "With DataOps, you're able to switch gears and produce the analysis. You may not be able to produce a 100% picture, but at least you can provide a working draft."
Producing a quick working draft provides two benefits. First, it demonstrates that the analyst is actively working on the problem. More importantly, it gives the executive and the analyst an opportunity to refine and question an analysis if necessary.
Who should be involved in DataOps?
DataOps engineer titles are emerging, but Heudecker said the problem with that is that data engineers tend not to act as the interface between DataOps and data consumers.
"We're seeing the emergence of a data product manager and that person's role is to collaborate with business stakeholders and relay those requirements to the data engineering team or teams," Heudecker said. "That data product manager may report to the chief data officer or a similar type of role."
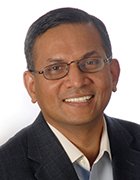
Anand Rao, global and U.S. data and analytics leader at PwC, underscored the need for someone who understands everything from the data in the data lake to streaming data as well as a data scientist who's building models.
Although a DataOps architecture is primarily considered a technical function, it's also important to understand the use cases, including who will use the data and why. That means understanding the scope of queries and the data necessary to answer those queries for reporting, analytics and machine learning purposes.
DataOps challenges
Technologically speaking, DataOps challenges can arise from tooling that isn't integrated. Even if the tools are integrated, Heudecker said they're unable to share data, performance information and other metrics, so it's a challenge to get a holistic view of the end-to-end process.
Heudecker said processes may be so ad hoc in a DataOps architecture that there's no standard process for onboarding a new data asset into the data pipeline. When the data is inconsistently managed and deployed, it causes data reliability problems.
Rajesh Parab, research director of applications for data and analytics at Info-Tech Research Group, said many organizations struggle to drive the value they could get from analytics because they haven't optimized their practice. Instead, they're throwing tools at the problem and expecting too much from end users and analysts. For one thing, end users tend not to be data literate.
"Culture and data literacy are probably the two biggest challenges teams are facing," Parab said. "Most organizations are looking at these buzzwords and to adopt [a DataOps] practice, [but] you're not building hundreds of reports and tens of dashboards. What problem are you solving and how does it help your business? How do [you] turn that data solution into a data product or a service for your end users?"
Bottom line
DataOps is an emerging practice designed to create data pipelines so users, analysts and data scientists can access the governed, compliant and secure information they need faster and easier. Creating a DataOps architecture should be done within several contexts, including how data is collected, managed and used in the organization and how those things may change in the future to meet business goals.