Utilize AI for cloud cost optimization
The sticker shock of cloud bills is still a pain point for enterprises. Providers are looking to AI to fix the problem, but can this technology actually meet customers' needs?
Cloud cost optimization is a challenge that isn't going away anytime soon.
Enterprises can train staff on best practices, seek out cloud services that better fit their workloads, and utilize monitoring and cost calculator tools to cut expenses. But, even with those efforts, cost optimization remains a major obstacle as companies underpin more of their business initiatives with the cloud.
This is why enterprises and IT vendors see potential in AI and machine learning as ways to provide more granular control, in-depth anomaly analysis and improved alert management.
The AI effect
The use of AI and machine learning as cloud cost optimization tools is still a work in progress, but some use cases have already begun to emerge. Vendors such as Yotascale use AI as part of services that scan cloud workloads to identify spending spikes and their causes. Machine learning is used to continually improve anomaly detection and present more relevant results.
These tools can work quickly enough that they are able to identify cost anomalies in your billing before your financial department notices. For example, a cloud administrator can receive an alert about a one-off configuration that might drive up the company's bill.
This article is part of
What is cloud management? Definition, benefits and guide
AI and machine learning models can also be used for real-time application resource management through the simultaneous optimization of performance, compliance and costs. This action happens at the cloud team level with other stakeholders being able to access views and reporting.
AI options already available and what comes next
Cloud management vendors have begun to incorporate versions of these types of machine learning or AI models into their offerings. For example, Turbonomic has an AI-based decision engine that continually monitors and recommends reserved instances in concert with resizing automation, while CloudSqueeze uses deep learning to analyze cloud-generated data to predict scaling and resource demands.
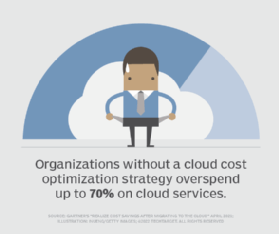
As AI gets better as a service, users can expect to see it power more dynamic views and predictive analysis into the financial impacts of cloud operations and resource management. It could also be used to scan existing workloads and then integrate with cloud catalogs to find services that are a more cost-efficient fit.
AWS, Microsoft and Google have yet to delve too deeply in these areas, but growing customer demands for AI as a cost efficiency tool will likely prompt them to do so sooner rather than later. In addition to offering AI-backed services to optimize costs, this shift could also lead cloud providers to offer new, aggressive pricing models for underlying services as they try to capitalize on the demand for more granular control.
The emergence of AI for cloud cost optimization is also sure to fuel the arms race between established IT management vendors and cloud-native startups. The companies that win out will likely have the algorithm development experience, technical talent and channel presence required to build and market these services.
However, this technological evolution won't come without pain points along the way. Pricing will be all over the place as enterprises work out the kinks with these new kinds of tools. Fee-based professional services are bound to be a requirement, and it's likely that models training for specific workloads will be more time-consuming than vendors and their customers allotted for in their initial implementation timelines.
And, considering the current cloud market and the fear and uncertainty that cloud spending brings, enterprises will have to be cautious about sketchy marketing moves and AI washing, which tries to capitalize on the hype around machine learning.
AI augments humans
Of course, we may never reach a point where cloud cost optimization is going to be completely machine-driven. There's no substitute for building cloud cost optimization expertise in-house. Cloud solution architects, developers and operations staff still need a grounding in cloud economic principles.
First and foremost, current and future AI-driven cloud cost optimization services should enhance the work of humans, not replace them. Organizations struggling with cloud spend can look to AI to cover granular details they've missed in the past and provide a new level of back-end data. But enterprises need their people to be well positioned to take action based on what AI dredges up about their resource and infrastructure management.
Cloud cost optimization is already tricky -- even experienced, cloud-first organizations struggle with it. Adding a set of artificial eyes to their workload might complicate things further, so enterprises need to ensure their IT teams have their training and processes in place first.