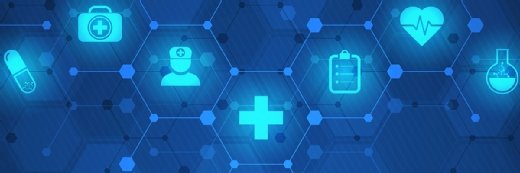
bestbrk/istock via Getty Images
Using Machine Learning to Calculate Unreported COVID-19 Cases
Researchers at the Chan Zuckerberg Biohub are leveraging machine learning to estimate the number of undetected COVID-19 cases.
To reduce and track the spread of COVID-19, researchers and provider organizations have increasingly turned to artificial intelligence and machine learning tools to improve their surveillance efforts.
For more coronavirus updates, visit our resource page, updated twice daily by Xtelligent Healthcare Media.
From predicting patient outcomes to anticipating future hotspots across the country, big data analytics systems have helped health leaders stay ahead of the pandemic, resulting in more efficient care delivery.
However, healthcare organizations’ level of pandemic preparation is only as good as the data available to them. While the industry is no stranger to data issues, the COVID-19 pandemic has brought a host of unique challenges to the forefront of care delivery.
The novel, global nature of the virus has led to significant gaps in COVID-19 data, with inconsistencies in information leaving officials unsure of the effectiveness of public health interventions.
“It's now well-known that asymptomatic infections are a common phenomenon in the spread of coronavirus. And it's very important to understand that phenomenon because, depending on how many asymptomatic infections there are, public health interventions might be different,” Lucy Li, PhD, data scientist at the Chan Zuckerberg Biohub, told HealthITAnalytics.
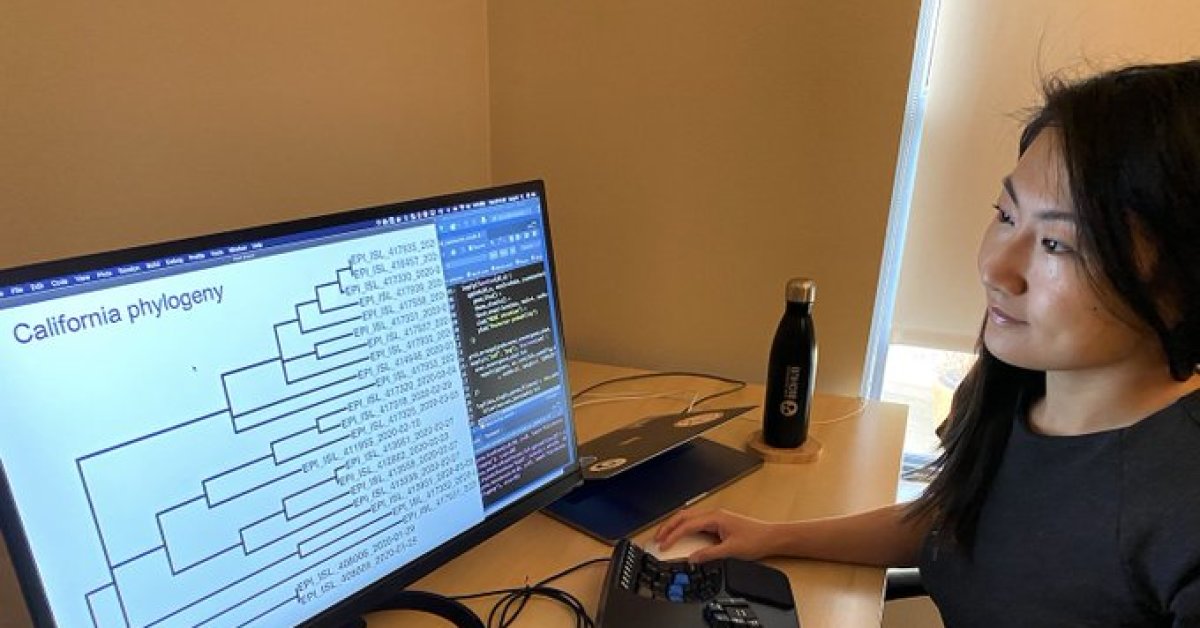
Researchers at the Chan Zuckerberg Biohub are working to overcome this challenge. Using machine learning and cloud computing technology, Li estimated the number of undetected infections at 12 locations in Asia, Europe, and the US over the course of the pandemic.
The results showed that a wide range of infections were undetected in these locations, with the rate of undetected infections as high as over 90 percent in Shanghai.
Additionally, when the virus was first transmitted to these 12 locations, over 98 percent of infections were undetected during the first few weeks of the outbreak. This suggests that the pandemic was already well underway by the time intense testing began to occur.
These findings have important implications for public health policy and provider organizations, Li noted.
“For disease outbreaks where you can detect every single infection, rapid testing and just a small amount of contact tracing is enough to get the epidemic under control. But for coronavirus, because there are so many asymptomatic infections out there, testing alone won't help control the pandemic,” she said.
“Because usually when you do testing, you’re testing symptomatic patients. But that's only a subset of the total number of infections out there. You're really missing a lot of people who are able to spread the infection, but are not quarantining. Being able to get a sense of what that number might be is helpful for allocating resources.”
Li’s research was supported by the AWS Diagnostic Development Initiative, a global effort to accelerate diagnostic research and innovation during the COVID-19 pandemic and to help mitigate future disease outbreaks.
The initiative allows individuals to take advantage of the cloud and other innovative tools, something that Li said was essential for her research.
“The data I'm using are the viral genomes – the viral DNA. As the viral genomes spread through the population, they accumulate mutations. Generally, these mutations are not good or bad, they're just changes in the genome. Every time the virus is spread to a new person, it could accumulate new mutations. So, if we know how quickly the virus mutates, we can infer how many missing transmission links there were in between the observed genomes,” she said.
“That’s the data I’m fitting the models to. And because there are many different scenarios that could explain what we see in the viral genomes, I have to leverage machine learning and cloud computing to test all of those hypotheses and to see which one can explain the observed changes in the viral genomes.”
These data analytics tools are well-suited to meeting the challenges brought on by COVID-19, Li pointed out.
“In order to try to quantify the unreported infections, we formulate models of how disease spreads in the population. And then we generate many simulations from these models, and we find out which of those simulations fits the data that we see,” she said.
“That allows us to test different levels of under-reporting and understand which of those can best explain the data that we see. That's not really possible without a lot of computational resources, and it's a very time-intensive process. The machine learning tool allows us to explore different explanations of the data that we're seeing, and we can test many hypotheses. It's a crucial tool for this type of analysis.”
With machine learning and cloud computing technologies, Li was able to streamline a previously time-consuming task.
“Before cloud computing became more common and these big computational resources became available, some of these analyses could take months to run. I've seen papers that were based on months of running a very complex model,” Li said.
“But by having access to more computational resources in the cloud, we can shorten that time from months to days, because we're able to leverage much more memory and better parallelize our analysis.”
The research could help public health officials monitor the rate of under-reporting in real-time, which could indicate how well current surveillance systems are operating.
“The better the current public health surveillance system is at detecting infections, the fewer underreported cases we would have. But if we see the underreported cases increasing, that would suggest that there needs to be more testing in the population. The results of this research can help the public health department determine how much more testing they would need,” Li said.
“This type of research can also help indicate how close we are to the end of the pandemic. By tracking how many people in the population have been infected by the virus or the number of undetected cases, we could get a sense of how far are we from eliminating this disease.”
With the amount of information generated by the COVID-19 pandemic, analytics tools are critical for uncovering new insights and potential solutions.
“Since the start of the pandemic, we've racked our brains to figure out what we can do to help the public health departments in reducing the spread of COVID-19. The number one request that we get from public health departments is information. And sometimes, just presenting the raw data to these departments is sufficient by itself,” Li concluded.
“But quite often, we need to use machine learning and mathematical models to infer these parameters or numbers that we can't directly see in the data. There has been so much effort from different research groups around the world in developing new models to help us tease out the underlying information that's not obvious from the data alone.”