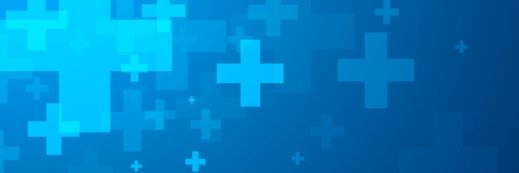
Getty Images
5 Successful Risk Scoring Tips to Improve Predictive Analytics
Strategies for successful risk scoring can improve predictive analytics and population health management.
Risk scoring allows organizations to understand their population based on defined risk factors and anticipate the future risk of the group. A risk score is a metric used to predict aspects of a patient’s care (cost, risk of hospitalization, etc.). This metric is developed using indicators from the patient and compared to a standard population.
By using risk adjustment, payers and providers can properly allocate the tools and costs needed to care for their patient population. Most often, health plans use risk adjustment to determine reimbursement rates, but integrated care plans also use the practice to predict the expected costs of treatment for their insured patients.
For example, a 55-year-old male with heart disease, diabetes, and depression would have a higher risk score than a healthy 24-year-old female marathon runner. Based on historical data, the male patient would be more likely to have complex medical issues, perhaps be seen in the emergency room more, and incur higher costs for a health plan. As plans and care management teams establish budgets and resource capabilities, understanding the risks of their population will help maximize population health management and minimize unnecessary cost burdens.
In a health system that is shifting away from a fee-for-service model of payment and toward value-based reimbursement, providers and payers must understand the risks of their specific patient population in order to optimize care for patients. Risk scoring a vital piece of the puzzle.
When selecting a method for risk scoring, several aspects of a model need to be considered: the risk factors or indicators used to make the prediction, underlying data integrity, methodology preference, and resource capabilities.
Select Indicators that Best Represent the Risk Factors of the Population
Traditionally, only two indicators formed the basis when calculating a risk score: age and gender. Many newer models still include these basic demographic details but have grown to include many additional indicators.
The most commonly used additional indicators are diagnostic information and disease status. Not all conditions qualify for inclusion in every risk model. Many models consider a hierarchy of disease based on the severity and cost associated with the disease. But the easiest way to underestimate an individual's risk is to include neither their diagnosis nor other indicators in the model. Continuously reassessing which indicators should be included in a model helps to minimize the risk of underestimating individuals’ risks.
Information on diagnoses and disease status can be obtained from medical records, claims, hospital discharge, or prescription drug data.
Some risk scoring models consider additional indicators: severity of disease, prescription drug utilization, disability and employment status, and Medicare/Medicaid eligibility.
There are several disease-specific risk adjustment methodologies (e.g., the Framingham Risk Score for cardiovascular disease) which might be more appropriate to use than general population-level methods. A strong understanding of the underlying population when selecting indicators is the first step in successful risk scoring and begins the process of narrowing down which model fits an organization best.
Use High-Quality Data Sources and Ensure Data Integrity
Different types of data can be used to define indicators. Mortality, morbidity, pharmaceutical, and self-report are the most frequently used, each with its own strengths and pitfalls.
Mortality data is easily ascertained as hospitals and public record widely report this information. However, a hospital reporting a high mortality rate is not necessarily a lower quality hospital. This hospital could have a particularly sick patient population or see more cases of a specific disease than other institutions. The underlying patient population must be known for comparability. If mortality data is used to determine indicators, the data must be adjusted to the underlying patient population.
Morbidity data gives a more holistic view of the patient’s health status as it captures chronic and debilitating conditions as well as acute diagnoses. This information can be ascertained from inpatient and/or outpatient records as well as through diagnostic information. Models can focus on only one type of claim (e.g., inpatient) or examine all points of care in an integrated approach. Diagnostic information is likely to be more accurate as it is not subject to the interpretation of treatments and procedures for various conditions. However, there is the potential for upcoding in this data as it is primarily a cost-based classification.
Pharmaceutical data can be used to determine chronic conditions and supplement traditional morbidity data. This data tends to be more readily available and standardized as a result of the Anatomical Therapeutic Chemical Classification System with Defined Daily Doses from the World Health Organization. Standardization like this can help prevent upcoding.
Self-reported health status data can be used to capture more of the social determinants of health impacting an individual’s health status and severity of a disease. While standard methods such as the SF-12 and SF-36 can be used to collect patient-reported health status, specific information related to social determinants of health such as socioeconomic status are less likely to be reported and can be difficult to obtain from individuals.
Not all data is created equal. To score risk successfully, the underlying data source must be high-quality and accurate. Risk scoring is only as good as the data it uses. If there is inaccurate or missing information, the risk assessment will also be inaccurate. Standardize coding practices ensure the most accurate information is captured and regularly check the data's integrity. Standard coding practices and definition of indicators can help to minimize discrepancies and ensure the availability of high-quality data. Regular review of the data will also help to make sure it is as up to date and accurate as possible.
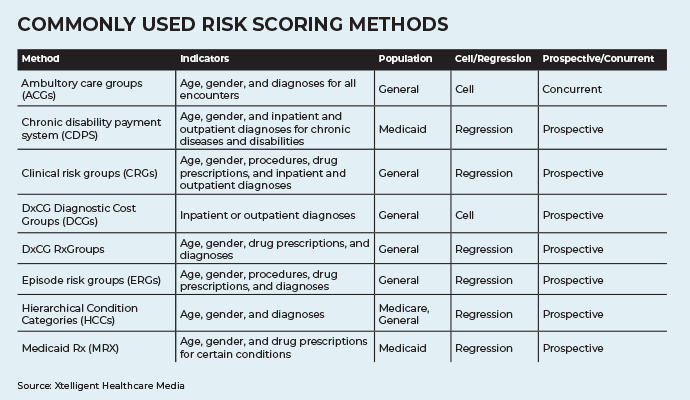
Establish a Methodological Framework
Once important indicators are established, the next step to successful risk scoring is to understand the different methodologies behind risk scoring.
There are two different frameworks commonly used to implement risk scoring. The first is a cell-based method. In this approach, each member of the population falls into a risk "bucket" or cell based on her indicators. Typically, the patients with the most complex medical needs and highest cost are sorted first and subsequent patients follow until the lowest risk and lowest cost patients are placed into the final buckets.
The second framework is the regression method. In this method, each of the established indicators is used to form a predictive regression line for the risk of the entire population. An individual’s risk is then determined by fitting her unique set of indicators to the regression line.
A regression method is significantly more complex as it requires a large number of predictors to create the most accurate model and advanced statistical knowledge. However, this method is often considered to be more accurate than the cell-based method.
The best method to use depends on resource availability and the goal of risk scoring for each organization. However, several models using different methods require the same indicators, so the two methods are not necessarily mutually exclusive. Once all the indicators have been collected, patients can be divided into buckets and a regression approach can be used. The results of these two methods can subsequently be compared.
Understand Different Ways to Look at the Model
After selecting a risk-scoring methodology, the data can be examined in two different time frames: prospectively or retrospectively.
Prospective risk uses historical claims to predict future risk. This method is beneficial to a user with several years of data which will allow for an examination of a population’s risk over time.
Retrospective risk, also called concurrent risk, uses risks in the same year to predict that year’s risk. Imagine a typically healthy patient who broke his arm in a biking accident this year. His spending would be much higher than it was historically. However, the following year he is expected to return to his normal, healthy self. Therefore, using his historical risk would not be beneficial to understanding his current risk score.
While the concurrent risk methodology is more complex and requires more advanced statistical techniques, many analysts believe it is a more accurate assessment of an individual’s risk at a particular moment because of potential accidental or shock claims.
Several risk scoring models can be looked at prospectively and concurrently provided the data is available. The models use the same indicators and change the approach to looking at the model allowing the user to compare risk scores based on these two different approaches.
Set Realistic Expectations Based on Resource Availability
Successful risk scoring can be difficult in both resource-limited and non-resource-limited settings. There are significant administrative costs associated with implementing these methodologies, including:
- finding and securing staff with advanced knowledge of statistics
- training for software utilization and standard practices
- data quality assurance strategies
- implementation specialists
Several of the most common methodologies have software available to help with risk scoring. However, these often have a licensing fee and limit the number of people in an organization who can use the software.
Setting realistic expectations about an organization’s capacity is key to successful risk scoring. When just beginning to risk score, it is unlikely there will be robust data to use for predictive analysis. Demographic and other population-level factors can be used to predict risk until a more robust claims database is created and can be incorporated into risk assessment. This phase-in implementation methodology will help to minimize problems resulting from a lack of data in the early phases of development.
Conclusion
Risk scoring is beneficial both for its predictive capabilities and its application to population health management. However, selecting the proper risk scoring model for a population can be complicated. To find a model that is the best fit for an organizations goals, first establish a set of indicators that will be used to define an individual's risk. Then, ensure data quality and standardization techniques followed by creating a meaningful methodological approach that best fits the goals of risk scoring. Finally, consider an organization’s resource capabilities and set realistic expectations of what can be achieved.
The key to successful risk scoring is to start the conversation about the goals and needs of risk scoring, which will then help to narrow down the best model to help achieve those goals.