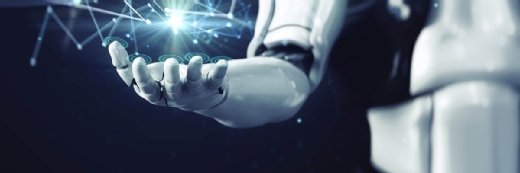
Getty Images
Putting the Pieces Together for a Successful Predictive Analytics Strategy
Good data, solid use cases, and a culture of transformation form the basis of a successful predictive analytics strategy in healthcare.
If the evolution of the healthcare industry were a jigsaw puzzle, then predictive analytics would be the last pieces put into place.
For years, experts have talked about the potential for artificial intelligence, machine learning, and natural language processing to bring together disparate sources of data. Experts believe the technologies can provide clinicians with a clear, complete picture of the medical landscape – one where all the pieces fit.
Perhaps one of the most promising capabilities tied to big data and analytics tools is the ability to predict an outcome based on past events, allowing providers to make more informed decisions.
Predictive analytics can help organizations improve anything from chronic disease management to patient engagement and precision medicine. For those looking to stay one step ahead of the uncertainty that pervades healthcare, predictive analytics tools offer a viable way to anticipate potential consequences before they occur.
Payers and providers alike have recognized the benefit of predictive insights, and many are currently leveraging these strategies within their own organizations. In a 2019 survey conducted by the Society of Actuaries (SOA), 60 percent of healthcare executives reported using predictive analytics, and 20 percent said they plan to use predictive analytics within the next year.
But before stakeholders can put the fragments together and stand back to see the big picture, they’ll need to evaluate the small, individual pieces of the puzzle.
Data challenges, clinician buy-in, and confusion about how to transform information into actionable clinical insights continue to hinder the use of predictive analytics in the medical field.
The same SOA survey showed that executives feel they have too much data to implement a predictive analytics solution, while many aren’t confident in their organization’s skill level or knowledge when it comes to predictive analytics use.
Overcoming these issues will require leaders to start with the basics: assessing the quality of their information, defining the most pressing issues among their patient populations, and most importantly, fostering an environment of change and advancement within their organizations.
By having the right people use the right information to stay ahead of potential events, healthcare organizations can leverage predictive insights to enhance care delivery and health outcomes.
To find the right answers, start with the right data
Like almost any healthcare strategy, the foundation of a predictive analytics strategy is complete, clean, and accurate data. In order to obtain a reliable glimpse of the future, providers need to have a comprehensive idea of what’s happened in the past.
But as anyone familiar with the medical field knows, this is typically easier said than done. There is no shortage of information in healthcare, and while this is a good thing for developing analytics technologies, it’s simply overwhelming for humans.
“It used to be that a physician could review a chart and the abnormal results were flagged – and they still are – but for every ten laboratory results, maybe now there are factors of ten. And there are new laboratory tests and new clinical findings developing every day. It’s getting beyond the realm of human comprehension,” Donna Wolk, MHA, PhD, division director of Molecular and Microbial Diagnostics and Development at Geisinger Health System, recently told HealthITAnalytics.
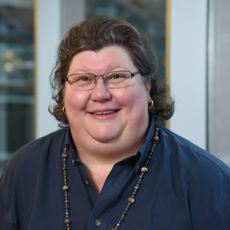
“Vetting the data, cleaning the data, validating the data, and working through the data with people who understand the human side of things is really the crux of what has to happen, at least in healthcare. Because we don't get the luxury of making an error.”
The delicate nature of predictive analytics is well-illustrated in use cases involving sepsis, a potentially life-threatening condition impacting more than 1.7 million Americans each year. While early intervention is essential to prevent complications, the condition is complex and often difficult to identify.
“There are other diseases that mimic sepsis, and there is no true pattern to the condition,” said Wolk.
“There are lots of publications that show the elderly, neonates, people with cancer, people with immune suppression, and transplant patients are at higher risk. But there are other normal, everyday factors that we can attribute to sepsis risk and septic shock.”
Wolk and her team recently partnered with the IBM Data Science Elite team to build predictive models that could help providers identify patients at high risk of developing sepsis. Using EHR data from patients over a ten-year period, the group identified about 30 factors associated with higher risk of septic death for hospitalized individuals.
“This project included a wide array of laboratory data, as well as the usual clinical symptomology like fever and white blood cell counts. But we also included information on microbiology and drug-resistant organisms – some of which did show up as associations,” Wolk said.
"There are new laboratory tests and new clinical findings developing every day. It’s getting beyond the realm of human comprehension."
Going beyond the scope of what’s contained in the EHR can significantly improve the accuracy of predictive models – provided the information is reliable, noted Oscar C. Marroquin, MD, chief clinical analytics officer at University of Pittsburgh Medical Center (UPMC).
“It’s necessary to have large amounts of data that can be used to train predictive models and ensure accuracy,” he said.
“However, you have to acknowledge that the data underlying the analytics were not necessarily created for analytics purposes, but for billing, care delivery, and other tasks. Early on, we spent most of our time not building predictive models but rather doing all of the necessary work grooming that data—harmonizing and cleaning it—so that the models are usable.”
For clinicians seeking the root causes of costly, adverse medical events, it’s helpful to recognize that more doesn’t always equal better.
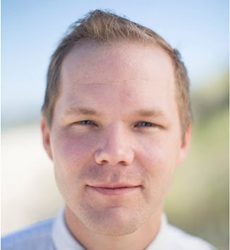
“These days the big push is for big data. The idea is that if we get enough data, we can draw conclusions about things. But really, big data is only helpful if it's good data,” said Kyle Morawski, MD, primary care doctor at Atrius Health.
Morawski and a team of researchers developed a model to predict hospitalizations among diverse groups of patients. They used information already available to most health systems, acknowledging that certain sources would be more reliable for certain data points.
“There isn’t regulation or standardization in the EHR, so clinical diagnoses or events such as hospitalizations are probably more accurate in claims data than in a patient’s medical record,” Morawski explained. “But there are certain things that are not in claims, such as the types of medicines a patient is taking. That kind of granularity is best left to the EHR.”
By drawing accurate, comprehensive data from reliable sources, the team was able to build and compare the performance of three models: one using EHR data, one using claims data, and a third that combined both EHR and claims information.
“In the end, it turned out that both the EHR-derived model and the claims-derived model had solid predictive value. Putting both data sources together made the predictive value even better,” said Morawski.
Big data, but make it actionable
Having the right data and making the right predictions are challenging enough on their own, but these tasks are only at the base of the predictive analytics mountain. After identifying trends and recognizing the risks, the question is, what’s next?
“Once you predict something, what do you do with that prediction? How do you actually make someone's life better?” asked Morawski. “The information we have is a lot of math. We’re using odds and ratios, and you put all that together and you get an output. But then what do you do with that? That's actually the hardest part – trying to use the models you get to improve outcomes and improve patients' lives.”
For Marroquin, improvements happen once providers can identify the root causes of events.
“Rarely do people come to us and say, ‘I need a predictive analytics solution for problem X.’ More often than not, our clinicians or administrators are asking us to help understand why something is happening,” he said. “Our work always starts with a question that our clinicians and leaders are trying to tackle about how we are delivering care or how efficient or inefficient we are in a particular area.”
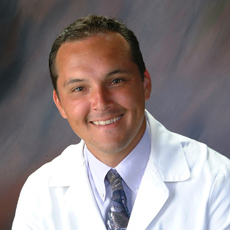
For example, providers could ask why a certain group of patients is at greater risk than others for hospital readmissions, Marroquin said. From there, clinicians can generate hypotheses, build analytics models, and seize opportunities to improve patient care.
“It’s part of a continuum of data-driven exploration and discovery, development of insights, and identification of opportunities that lead to the design and build of our predictive models. Predictive models are used as tools that can help us implement change,” he continued.
At Atrius Health, clinicians try to make a habit of translating data and numbers into actionable practices.
“We try to call patients whom we predict are at high risk of hospitalization once a quarter. We’ll just say, ‘Hey, if you need anything, or if anything didn't get done while you were here in the clinic, we’re available to you.’ So instead of waiting for them to come to us, we're being proactive and calling them,” Morawski said.
“We’re trying to not just show the math behind the prediction, but also provide possible interventions that could help mitigate risk,” he stressed.
"Our clinicians or administrators are asking us to help understand why something is happening."
In other cases, converting research into clinical practice takes a little more time to develop, explained Vida Abedi, PhD, Geisinger staff scientist in the Department of Molecular and Functional Genomics and researcher on the Geisinger-IBM sepsis project.
“Once we fine-tune the model, our next step is actually implementing the algorithm in the EHR using a prospective approach. So, we’ll be evaluating what happens over time and looking at our data and seeing how we can improve the model iteratively,” she said.
“Things change all the time in clinical settings – medications, new factors associated with disease – and these things have to be integrated into the final model.”
The end goal, always, is to deliver preventive, proactive care.
“After we prove that the associations are real one at a time, we’ll try to develop a pattern of clinical and laboratory variables that could be used to alert our providers that this person is at risk for developing sepsis,” Wolk said. “Our overall aim is to flag those patients as high risk in real time and focus on the patients that need the care the most before they can’t recover from the septic event.”
Teamwork makes the anticipated dream work
One of the most critical, but often overlooked, pieces of the analytics puzzle is also the most integral part of the healthcare system: providers themselves.
With all the talk about data and technology, it can be easy to neglect the people who will use these algorithms on a day-to-day basis. But it’s essential to involve them in algorithm development, Marroquin stated.
“Part of the process we’ve implemented is to ensure that our data scientists aren’t kept in isolation where they are building these algorithms in a vacuum. Our data scientists work closely with our clinicians and leaders, participating in the whole process together. This leads to synergies and alignment that translates into buy-in for the analytics that we produce,” he said.
“Being able to win acceptance of your models by clinicians—who are skeptics by nature—is often a challenge. By making end-users part of the whole process, we’ve been able to overcome that in the majority of cases.”
Because the potential of analytics models also comes with well-documented concerns, clinicians may be hesitant to adopt and use these tools. Anxieties over data privacy and security, the depersonalization of care delivery, and overly complex algorithms can hinder analytics implementation.
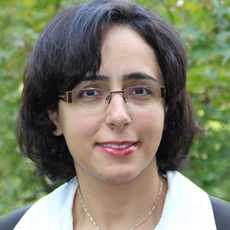
Many are also afraid these new methods will perpetuate care disparities and inequities. A recent study found that a predictive analytics platform referring high-risk patients to care management programs was skewed to favor white, healthier patients over less healthy black patients.
Additionally, there is the fear that these decision support tools will only make care delivery more complicated, inundating providers with unnecessary alerts and alarms that will result in patient safety issues and clinician burnout.
“As AI and machine learning are moving into the high-risk healthcare field, it’s very important to keep humans in the loop. The models are there to support the physicians and to help them make the best possible decisions for the future,” said Abedi. “And to do that, we really need to work hand in hand with the clinical laboratory. All the pieces have to be in place to make sure that the models are actually doing what they are supposed to do, and not making healthcare more complex.”
At Geisinger, an interdisciplinary team of clinicians and researchers from the laboratory, data sciences, intensive care unit, and emergency department came together to collaborate on the sepsis risk prediction project.
“We worked together to come up with a body of literature to feed into the software because even though the infrastructure was there, there was a lot of back and forth to try to design it in a way that it could be useful for the clinicians. At the end of the day, the interaction between the clinical team and the researchers is what’s going to make the algorithm useful,” Abedi said.
“As AI and machine learning are moving into the high-risk healthcare field, it’s very important to keep humans in the loop."
While it may take more time, collaboration across the industry will drastically improve the end result, Wolk noted.
“We take our time to include people from all walks of healthcare into our processes. And it does slow things down for sure. But that’s what has to happen for us to actually leverage this technology appropriately and safely,” she said.
In order to create a culture where change and innovation are accepted, those at the top of the heap should employ effective and positive Change management.
“In addition to the analysts and the data scientists, organizations need to have engaged clinicians who are willing to roll up their sleeves to work with them to come up with models that are accurate and conducive to being used. And the executives of the organization have to provide the support and institutional culture that make analytics a priority in the organization and not just a ‘nice to have,’” Marroquin said.
The emphasis shouldn’t be on perfection, but instead on trial and error to find a solution that really works, Morawski said.
“The most important thing is having a culture where experimentation and measuring outcomes is encouraged. Everyone in a healthcare organization should know what outcome they’re looking for and assess whether they’re getting there. And if they’re not, they need to be willing to try,” he stated.
Ultimately, the successful use of predictive analytics in healthcare will depend on several moving pieces. It’s about fitting these pieces together to create a comprehensive, holistic picture of health at the patient and population levels.
“There are no boundaries on predictive analytics right now,” said Wolk. “Just like everything else we do in healthcare, the idea behind it needs to be, first, do no harm. If everyone uses these tools in the same manner, we will be able to improve patient care and predict who needs our help and when.”