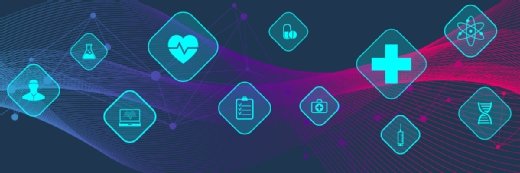
BAIVECTOR - stock.adobe.com
Novel Technique Uses Clinical Data to Predict Disease Risk
A new study described how researchers used clinical data from 53 million patient notes to identify disease tendencies and patterns.
Published in the journal Artificial Intelligence in Medicine, new study findings describe how researchers from Children’s Hospital of Philadelphia (CHOP) and Drexel University used a new technique to gather patient and clinical data and determine a correlation between medical histories and common disease patterns.
Although new technologies have helped advance data analysis, a lack of standardization often leads researchers to describe similar symptoms differently, the press release notes.
Previously, the Epilepsy Neurogenetics Initiative (ENGIN) at CHOP analyzed clinical data to determine genetic targets in patients with different genetic childhood epilepsies. Building off this, the Department of Biomedical Health Informatics (DBHi) at CHOP, the College of Computing and Informatics at Drexel University, and ENGIN began using a suite of tools known as Arcus.
Created at CHOP, Arcus combines biological, clinical, research, and environmental data to assist in conducting new research efforts involving large data.
“This work follows trends in the artificial intelligence and machine learning field where complex, disparate information is transformed into something we can represent in a standardized way, thereby allowing machines to classify medical conditions and predict future disease risk,” said senior study author Scott Haag, PhD, assistant research professor in the Department of Computer Science at Drexel’s College of Computing & Informatics and the supervisor of the Arcus Data Science Team at CHOP, in a press release.
The researchers recently conducted a study that involved an analysis of 53.9 million electronic notes from 1.5 million patients within the Arcus data repository. From the variety of diagnoses represented by the patient population, researchers identified a total of 9,477 phenotypes.
“This study demonstrated that utilizing an array made up of the clinical terms we identified could exceed the capacity of other much more computationally complex methods of analyzing phenotypes,” said first author Maryam Daniali, a PhD candidate in computer science at Drexel University, in a press release. “This allowed us to map similarities between phenotypes using millions of points of data, significantly surpassing previous methods that relied on thousands of data points.”
They also noted that this practice allowed for the division of phenotypes from the Human Phenotype Ontology (HPO) into their appropriate arrays, which could further assist researchers in deep phenotyping tasks. Containing over 15,000 clinical terms, the HPO is a resource for analyzing clinical information and allows for more efficient application of precision medicine into clinical practice.
Efforts surrounding predicting disease patterns are growing common as this can provide researchers with valuable insights.
In November 2022, a study published in JAMA Network Open described the creation of a risk-scoring tool to predict subjective dementia patterns. The researchers used data from a UK population-based prospective cohort study, focusing on five-, nine- 13-year dementia risks as outcomes. With a study population of 444,695 people, they found that dementia occurrence during the 13 years was 0.7 percent for men and 0.5 percent for women.
Based on this research, they also found that socioeconomic adversity, sleep phenotypes, physical activity, and comorbidities were all risk factors for dementia.