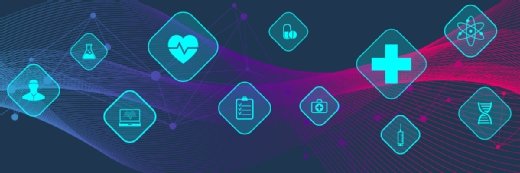
BAIVECTOR - stock.adobe.com
Machine learning enables prediction of pediatric urinary condition
New tool uses machine learning to forecast risk of dilating vesicoureteral reflux (VUR), a common cause of hydronephrosis in infants.
Researchers from Boston Children's Hospital have developed a machine learning model to predict risk of dilating vesicoureteral reflux (VUR) in infants with hydronephrosis – a condition in which urine backs up into the kidney.
Hydronephrosis is commonly identified using prenatal ultrasound, but these tests are limited in their ability to identify dilating VUR, one of the major causes of the condition. A voiding cystourethrogram (VCUG) can more accurately flag VUR, but the approach is costly and invasive.
To improve screening, the research team set out to develop a tool that can utilize ultrasound findings to identify which patients are at highest risk for VUR and may benefit from additional screening via VCUG.
To assess infants for hydronephrosis, urologists rely on a standardized system to classify urinary tract dilation (UTD) based on six pre- and post-natal ultrasound findings. The approach helps characterize UTD severity, as increased kidney dilation often indicates the presence of an anatomical abnormality, but the method cannot indicate a specific diagnosis or forecast patient outcomes.
To address this, the researchers developed a machine learning algorithm using data from 280 infants who had received ultrasounds for hydronephrosis and subsequent VCUGs to confirm VUR at Boston Children's Hospital.
The model uses imaging information and patient details – including UTD classification and demographic data – to predict dilating VUR risk in infants with hydronephrosis. The final model achieved high performance, identifying risk with an area under the curve of 0.81.
The analysis revealed gender, ureteral dilation, parenchymal thickness, parenchymal appearance and central calyceal dilation as significant predictors of VUR in the cohort.
The research team underscored that the model is both accurate and intuitive, making it easily interpretable by clinicians.
“The model can be easily reviewed and utilized in routine clinical settings when counseling patients for the next steps of management for hydronephrosis,” explained study lead Hsin-Hsiao (Scott) Wang, MD, a urologist at Boston Children’s and director of the health system’s Computational Healthcare Analytics Program, in the news release.
The researchers further noted that the tool may be the first of its kind to predict dilating VUR risk using early post-natal ultrasound findings.
Moving forward, they hope to improve the model by incorporating 15,000 patient records with the aim of eventually predicting whether a patient’s hydronephrosis will resolve on its own or require medical intervention to correct.
“We hope to build a crystal ball for urologists so kids don’t have to undergo unnecessary testing,” said Wang.
Artificial intelligence (AI) is increasingly being developed to improve care for pediatric conditions.
Last week, a research team from the University of Pittsburgh (Pitt) and UPMC detailed the development of an AI-driven smartphone application capable of diagnosing acute otitis media (AOM) in pediatric primary care.
AOM and other ear infections are common in pediatric patients, but differentiating between these conditions is critical for proper treatment. The researchers emphasized that AOM requires antibiotics to treat, unlike some other ear infections, which typically don’t involve bacteria or benefit from antibiotics.
However, discerning between AOM and other conditions requires intensive training, and AOM is often incorrectly diagnosed in primary care settings as a result.
The smartphone application is designed to address this by analyzing short videos of the eardrum taken using an otoscope to flag subtle visual findings and assist clinicians with diagnosis.