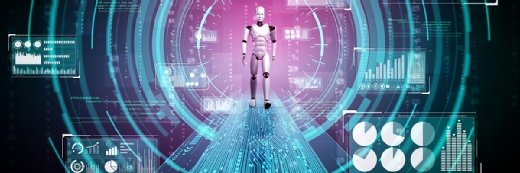
Getty Images
AI May Help Define Tumor Margins in Prostate Cancer Treatment
A new artificial intelligence tool may have the potential to improve tumor treatment margin definition and reduce prostate cancer recurrence rates.
Researchers evaluating an artificial intelligence (AI) model’s ability to estimate prostate cancer extent and define focal treatment margins for tumors demonstrated that the model outperformed conventional methods, according to a study published recently in European Urology Open Science.
University of Florida Health indicates that focal therapy for prostate cancer is a type of treatment that entails removing the tumor and a small margin of normal tissue surrounding the tumor in order to ensure that the cancer is removed without damaging any of the critical surrounding structures, such as those that control erectile function and urinary continence.
Properly estimating a tumor’s extent and defining the treatment margin are crucial aspects of the treatment process, but these rely on magnetic resonance imaging (MRI) techniques, which the study authors noted can underestimate the cancer’s true size and extent.
Such underestimation can complicate the definition of focal treatment margins, leading some experts to posit that AI may improve the accuracy of prostate tumor delineation.
To test this, the researchers conducted a retrospective study looking at a United States Food and Drug Administration (FDA)-cleared AI model’s ability to estimate cancer extent and produce treatment margins.
The model was given multimodal imaging and biopsy data from a cohort of 50 patients who had undergone radical prostatectomy for intermediate-risk cancer.
The AI utilized these data to generate three-dimensional cancer estimation maps and corresponding margins. These were taken and compared to conventional MRI-based regions of interest (ROIs), ten-millimeter margins around ROIs, and hemigland margins for the same tumors to assess the AI tool’s performance.
In addition, the AI model also provided predictions of negative surgical margin probability, which describes the likelihood that the margins encapsulated the entire tumor.
Overall, the AI was able to predict tumor margins more accurately than conventional methods.
The model’s margins had a higher mean sensitivity than those generated using MRI approaches, with the AI achieving 97 percent sensitivity compared to 37 percent for standard ROIs, 93 percent for ten-millimeter ROIs, and 94 percent for hemigland margins.
The AI also significantly outperformed the MRI-based approaches in terms of negative surgical margin probability, achieving up to a 90 percent negative margin rate compared to other methods.
Based on these findings, the researchers concluded that the model was both accurate and effective at estimating prostate cancer extent and defining treatment margins. Further, they noted that the approach has the potential to help standardize and improve treatment margin definition in the future, which could reduce cancer recurrence rates.
The research team also stated that a similar method could provide accurate assessments of negative margin probability and support clinical decision-making.
This research is the latest investigation into how advanced technologies can help advance prostate cancer care.
Earlier this month, Stanford Medicine researchers shared that they had developed a precision medicine approach to improve the accuracy of prostate cancer screening.
The personalized approach aims to identify and separate normal variations in prostate-specific antigen (PSA) caused by genetic factors unrelated to cancer, as PSA screening often suggests the presence of cancer when there is none, leading to overdiagnosis and overtreatment.
By evaluating the genomes and PSA levels of men without prostate cancer, the researchers identified genetic factors contributing to those variations.
Using these factors, the research team developed a polygenic score that accurately predicted nearly ten percent of PSA level variations, which the researchers determined could have spared some patients from unnecessary biopsies.