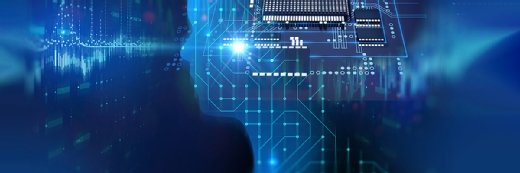
Getty Images
AI for Psychiatric Diagnosis Presents Bias, Clinical Applicability Issues
A new systematic review suggests that artificial intelligence models for psychiatric diagnosis display a high risk for bias and poor clinical applicability.
A systematic review published last week in JAMA Network Open evaluating potential risks of translating neuroimaging-based artificial intelligence (AI) models into direct clinical applications, such as psychiatric diagnosis, found that most models must address the risk of bias and other applicability issues prior to implementation in the clinical setting.
The researchers noted that a lack of biomarkers to inform psychiatric diagnostic practices has increased interest in AI- and machine learning (ML)-based neuroimaging approaches. These models aim to enable the use of what the authors call “an objective, symptom-centered, individualized and neurobiologically explicable estimate of psychiatric conditions,” compared to a clinician-based diagnosis, which relies on discrete symptoms.
However, the researchers indicated that the lack of evidence-based evaluations of such tools limits their application in clinical practice.
To help bridge this research gap, the authors conducted a review of 517 peer-reviewed, full-length studies discussing 555 neuroimaging-based AI models for psychiatric diagnostics published between Jan. 1, 1990, and March 16, 2022, and available via PubMed.
Following study selection, researchers extracted data to assess the risk of bias and reporting quality of each model using the Checklist for Critical Appraisal and Data Extraction for Systematic Reviews of Prediction Modeling Studies (CHARMS) and Preferred Reporting Items for Systematic Reviews and Meta-analyses (PRISMA) frameworks.
Benchmarks taken from the Prediction Model Risk of Bias Assessment Tool (PROBAST) and modified Checklist for Evaluation of Image-Based Artificial Intelligence Reports (CLEAR) were also used to evaluate the risk of bias and reporting quality.
Overall, 461 models were rated as having a high risk of bias, particularly in the analysis domain, due to factors such as inadequate sample size, poor model performance examination, and lack of handling data complexity. The models also showed poor reporting quality, with all articles providing incomplete reports for validation. The average reporting completeness across all models reached only 61.2 percent.
These findings show that the high risk of bias and poor reporting quality are significant barriers to the clinical applicability and feasibility of neuroimaging-based AI models for psychiatric diagnostics, the researchers stated. Moving forward, the authors recommend that work in this area address the risk of bias in these models before they are used in clinical practice.
This review highlights multiple challenges clinicians, researchers, and public health stakeholders face in using big data analytics to improve mental healthcare.
Another major hurdle when leveraging AI in this area is a broader concern within medical imaging: AI’s performance in image-based diagnostics.
Research published last year in Scientific Reports found that deep neural networks (DNNs) are more prone to making mistakes in image-based medical diagnoses than human clinicians, a variation that researchers hypothesize may indicate that clinicians and AI use different features for analysis when looking at medical images.
The research team could not conclude that humans and machines use different features to detect microcalcifications in breast cancer images, but they could show that the two must use different features to detect soft tissue lesions. The analyses of soft tissue lesions and microcalcifications were separated to help avoid artificially inflating the similarity between human and machine perception, which the researchers noted as a flaw in other research.
They ultimately concluded that more research was needed to improve medical imaging-based diagnostics.