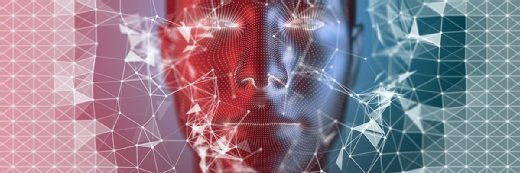
Getty Images
AI May Improve Patient Experience in Decentralized Clinical Trials
Stanford University researchers posit that artificial intelligence may help improve patient experience in decentralized clinical trials by addressing patient recruitment and retention challenges.
In an article published last month in Nature Medicine, researchers argued that artificial intelligence could improve patient experience in decentralized clinical trials through the use of reinforcement learning, computer vision, and AI models designed for temporal data.
Decentralized clinical trials, also known as ‘direct-to-participant trials’ or ‘virtual clinical trials,’ are clinical trials in which some or all health assessments are performed remotely in participants' homes instead of in clinical settings. This shift toward reduced dependence on traditional research facilities relies on tools such as telemedicine, sensory-based technologies, wearable medical devices, home visits, patient-driven virtual healthcare interfaces, and direct delivery of study drugs and materials to patients’ homes.
The authors noted that the COVID-19 pandemic accelerated the transition to decentralized clinical trials as researchers needed to continue to run trials despite the outbreak. They also pointed out that research suggests that in-home participation in clinical trials can help bolster patient recruitment and retention. One study out of Europe indicates that decentralized trials provide opportunities to recruit more diverse patient pools.
However, the authors argued that further innovation is needed to realize these potential benefits in decentralized clinical trials. Decentralization can increase the responsibility placed on trial participants, which may lead to reduced protocol adherence or patients dropping out, they explained.
The authors cited shortcomings in digital health interfaces as one challenge that decentralized trials must address, suggesting that automation via AI could spur user interface improvements.
Similarly, data collection poses a hurdle to decentralized clinical trials as the amount of data collected during trials increases. Much of this data collection is undertaken by study participants who must input their participation data by completing surveys, diaries, and other electronic clinical outcome assessments (eCOA) at home regularly because of the decentralized nature of the trial.
The authors indicated that asking participants to take on these time-consuming data-entry tasks can reduce trial protocol adherence or increase the number of data-entry errors. Some clinical trials leverage electronic notifications to remind participants when they need to complete these tasks, but an excessive number of tasks or notifications can increase not only the risk of errors but also of patients dropping out of the trial.
To combat this, the authors suggested using reinforcement learning, a domain of AI that trains models to make a sequence of decisions that maximizes the probability of achieving a desired outcome. In the case of decentralized clinical trials, the goal would be to identify which notifications to send to participants and when to send them to minimize total notifications while maximizing the completion of eCOA tasks. Using this approach, notifications could be customized to each participant.
Another challenge in some decentralized trials is the requirement for participants to submit images or videos, which is especially common in dermatological trials. The images or videos that participants need to submit must have the appropriate lighting, camera angle, and zoom, but adhering to these guidelines can be challenging for participants.
The authors stated that computer vision, which enables the automatic assessment of images and videos, can provide real-time advice for participants about required adjustments. This approach empowers participants to succeed quickly, improving patient experience, reducing the need to retake photos, and increasing efficiency in central quality control, they noted.
The authors' final concern with decentralized trials was related to tracking participant mobility. In trials that assess patient mobility, wearables and sensors are required to gather detailed information on movement patterns. However, applying the sensors is tedious, often requires live guidance, and can result in errors.
To address this, the authors suggested using AI models designed for temporal data, which can enable more detailed movement measurements with fewer body sensors. These models can be trained to take data from one body part to infer how another is moving. By leveraging AI, researchers could rely on fewer sensors, which may have the potential to allow them to measure mobility outcomes for longer periods and gain additional insights.
The authors concluded that similar technologies are already being implemented to enhance user experience across other industries outside healthcare and clinical trials. They stated that adopting AI in decentralized clinical trials could increase trial enrollment, trial completion, and participant submission of high-fidelity assessments throughout the trial.