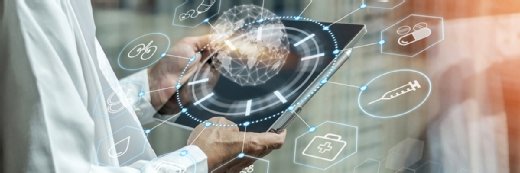
Chinnapong - stock.adobe.com
AI for Medical Imaging Boosts Cancer Screenings with Provider Aid
New research shows that a decision-referral approach in which radiologists and artificial intelligence work together for breast cancer screening is better than either’s performance alone.
A study published in The Lancet Digital Health this month highlights how a decision-referral approach, in which radiologists work with artificial intelligence (AI) models to evaluate breast cancer screenings, achieves better results than clinicians or algorithms would have alone.
The researchers note that the rise of AI use in medical imaging has spurred significant research into the development of accurate cancer screening algorithms. Some studies suggest that AI performs on par with clinicians in terms of image interpretation, but more evidence is needed in areas such as breast cancer screening. Other research further suggests that combining the strengths of radiologists and AI leads to improved screening accuracy, but this area also requires more research.
To add to these areas of medical research, the study’s authors set out to evaluate the performance of an AI, a radiologist, and the two together when tasked with breast cancer screening. To develop the AI model, the researchers used a retrospective dataset consisting of 1,193,197 full field, digital mammography studies carried out between Jan 1, 2007, and Dec 31, 2020. These mammograms were sourced from 453,104 patients at eight separate screening centers in Germany.
Data from six of the sites were used for model development and internal testing, and the data from the other two were used for model validation and external testing. The internal-test dataset consisted of 1,670 screen-detected cancers and 19,997 normal mammography exams, while the external-test dataset contained 2,793 screen-detected cancers and 80,058 normal exams. Labels used by the model to classify the images were derived from annotations of radiological findings and biopsy information.
Following model development, the researchers simulated a scenario in which the AI classified each image as normal or suspicious for cancer. As the model classified the images, it also gave an indication of its confidence on its classification. Any images deemed suspicious or that the AI was unconfident about were referred to the radiologist without any indications of the AI’s classification of the images.
By structuring the simulation in this way, the researchers could evaluate sensitivity and specificity for cancer detection in a scenario that mirrors how the AI would be used in a clinical setting. In the clinical setting, an AI’s predictions that were both labelled as normal and confident would not need to be shown to the radiologist for further consideration. However, those that were labelled as suspicious or unconfident would receive final classification from a radiologist.
On its own, the AI model achieved a sensitivity of 84.2 percent and a specificity of 89.5 percent on internal-test data, and a sensitivity of 84.6 percent and a specificity of 91.3 percent on external-test data. The radiologist achieved a sensitivity of 85.7 percent and a specificity of 93.4 percent on the internal-test dataset, and a sensitivity of 87.2 percent and a specificity of 93.4 percent on the external-test dataset.
The radiologist alone outperformed the AI, but the combined decision-referral approach that relied on both the clinician and the model achieved the highest performance, significantly improving the specificity and sensitivity scores.
These findings indicate that leveraging the combined strengths of AI and radiologists surpasses either’s performance alone. Because of this, the researchers posit, their decision-referral approach has the potential to improve the screening accuracy of radiologists, is adaptive to the requirements of screening, and could allow for the reduction of radiologist workload without sacrificing their expertise.