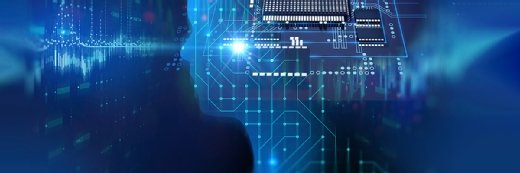
Getty Images
AI Framework Differentiates Alzheimer’s, Other Dementias
Researchers have developed an artificial intelligence framework that can successfully identify and differentiate between normal cognition, mild cognitive impairment, Alzheimer’s disease, and non-Alzheimer’s dementias.
A study published in Nature Communications outlines a newly-developed artificial intelligence (AI) framework that can successfully identify and differentiate between normal cognition (NC), mild cognitive impairment (MCI), Alzheimer’s disease (AD), and non-AD dementias (nADD) on par with neurologists and neuroradiologists.
The researchers note that AD is one of the most common causes of dementia worldwide, and that future cases may increase clinical demand past projected capacity of qualified specialists. This potential future lack of neurological specialists, and thus, lack of access to accurate diagnoses for patients with cognitive impairment or dementia, the authors argue, requires the development of clinical support tools to fill this gap.
To develop such a tool, the researchers turned to AI and deep learning. To create their framework, they developed and validated three deep learning models designed to classifying subjects with NC, MCI, AD, and nADD in multiple cohorts of participants with different causes of dementia and varying levels of cognitive function: an MRI model, a non-imaging variable model, and a combined model. Each model was developed and externally validated using data from the National Alzheimer’s Coordinating Center (NACC).
The models were then evaluated based on their ability to perform various differential diagnosis tasks. The first was the cognition (COG) task, which required the models to label patients as having either NC, MCI, or dementia (DE) due to any cause. The second was the Alzheimer’s disease/dementia (ADD) task, which all patients labelled as DE were then further labeled as AD or nADD. By completing these two tasks, the models were able to execute a four-way classification that fully delineated NC, MCI, AD, and nADD.
The combined model, which used both MRIs and clinical variables from demographics, past medical history, neuropsychological testing, and functional assessments, provided the most accurate classification performance of all models. The MRI-only model and the non-imaging variable model presented moderate performance reductions in comparison, but still achieved relatively high performance.
To further validate their models, the researchers also compared each one to both neurologists and neuroradiologists. Each clinician was asked to perform diagnostic tasks on a subset of the NACC dataset, and their results were compared to those of the models for the same data subset. In the overall four-way classification of NC, MCI, AD, and nADD, the accuracy of the combined model was found to reach that of the neurologists. A similar accuracy was achieved by the non-imaging model, but not the MRI model. However, the MRI model did achieve a moderate improvement in diagnostic accuracy over the neuroradiologists.
These findings indicate that these models are capable of achieving high performance across various cognitive statuses and dementia subtypes with flexible combinations of imaging and non-imaging input data. Because this framework relies on MRI and clinical data, the researchers note, it also demonstrates a strategy for expanding the scope of medical deep learning while ensuring that predictions remain grounded in established medical knowledge.