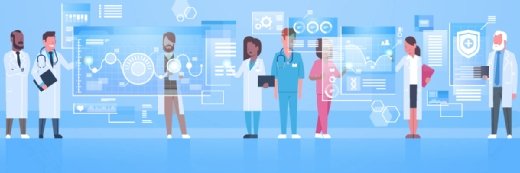
mast3r - stock.adobe.com
Predictive Analytics Warns of Potential Complications from COVID-19
A predictive analytics tool continuously monitors patients with COVID-19 and accurately forecasts possible clinical issues before they occur.
A team from UVA Health has developed a predictive analytics tool that can determine COVID-19 patients’ risk of experiencing an adverse event over the next 12 hours.
The tool, called CoMET, uses continuous monitoring and analytics algorithms to help clinicians measure patients’ stability and risk for clinical issues, as well as decide which actions should be taken to protect patients’ health.
CoMET creates a graphic representing risk on a large LCD screen, informed by minute-to-minute data drawn from a patient’s EKG, laboratory results, and vital signs. Using models updated every 15 minutes, the software can give clinicians enough time to get ahead of a potential crisis before it occurs.
For patients with COVID-19, whose prognoses can rapidly change, the system is especially valuable.
“Vital sign measurements and labs can come too late, but early detection through predictive analytics has the power to improve patients’ outcomes, especially for catastrophic illnesses like COVID-19,” said Randall Moorman, MD, a cardiologist and professor of medicine at UVA Health.
The platform shows stable patients’ comets as small, yellow, and close to the X-Y axis on the display. However, if the risk level rises, the comets will glow, turn bright orange or red, and move up and across the screen – indicating cardiovascular instability, respiratory instability, or both.
This signals providers to deploy proactive strategies to stabilize patients’ vital signs before serious medical events occur, like sepsis, blood poisoning, respiratory distress or cardiac instability, and the need for ICU-level care.
With the global surge in COVID-19 cases, predictive analytics tools will help clinicians determine the best possible courses of treatment for patients before it’s too late.
“Using precision predictive analytics systems like this one helps nurses initiate clinical response before the scenario becomes, quite literally, life and death,” said Jessica Keim-Malpass, a professor in the School of Nursing and Moorman’s research partner.
Recently, Keim-Malpass and Jaime Bourque, a UVA cardiologist, began a two-year, randomized controlled study of the CoMET tool across UVA’s entire fourth floor. In the coming years, they’ll randomly assign a CoMET display to half the beds and compare the outcomes of patients in the experimental and control groups to assess the tool’s impact.
CoMET is unique in that it analyzes each new data point from the patient’s EHR and bedside monitor, making sense of subtle changes across multiple predictors to continuously update and calculate their risk. Additionally, other patient monitoring systems provide a portrait of risk at four- or eight-hour intervals, or use alerts that contribute to alarm fatigue.
Beyond predicting adverse events, the CoMET tool also enables clinicians to evaluate the impact of therapies in real time, improves assessments and interventions through an additional health indicator, and gives nurses more autonomy as well as the ability to proactively deliver care.
The team expects that CoMET could help other health systems improve care for patients with COVID-19.
“In the fight against COVID-19, CoMET offers us the potential to change the clinical paradigm from reactive to proactive,” Keim-Malpass said.
In the months since COVID-19 has spread throughout the US, predictive analytics tools have been critical in the healthcare industry’s efforts to reduce the pandemic’s impact. From determining virus hotspots to filling gaps in critical data, predictive analytics technology has demonstrated its ability to help organizations get and stay ahead of poor outcomes.
Most recently, a study published in the British Journal of Anaesthesia revealed how predictive analytics could help researchers identify COVID-19 patients at risk of quickly deteriorating up to 24 hours in advance.
“You can see large variability in how different patients with COVID-19 do, even among close relatives with similar environments and genetic risk. At the peak of the surge, it was very difficult for clinicians to know how to plan and allocate resources,” said Nicholas J. Douville, one of the study’s lead authors.
“The closer we were to the event, the higher our ability to predict, which we expected. But we were still able to predict the outcomes with good discrimination at 48 hours, giving providers time to make alterations to the patient’s care or to mobilize resources.”